Recurrent Autoencoder with Sequence-Aware Encoding
ICCS (2)(2020)
摘要
Recurrent Neural Networks (RNN) received a vast amount of attention last decade. Recently, the architectures of Recurrent AutoEncoders (RAE) found many applications in practice. RAE can extract the semantically valuable information, called context that represents a latent space useful for further processing. Nevertheless, recurrent autoencoders are hard to train, and the training process takes much time. This paper proposes a new recurrent autoencoder architecture with sequence-aware encoding (RAES), and its second variant which employs a 1D Convolutional layer (RAESC) to improve its performance and flexibility. We discuss the advantages and disadvantages of the solution and prove that the recurrent autoencoder with sequence-aware encoding outperforms a standard RAE in terms of model training time in most cases. The extensive experiments performed on a dataset of generated sequences of signals shows the advantages of RAES(C). The results show that the proposed solution dominates over the standard RAE, and the training process is the order of magnitude faster.
更多查看译文
关键词
Recurrent,AutoEncoder,RNN,Sequence
AI 理解论文
溯源树
样例
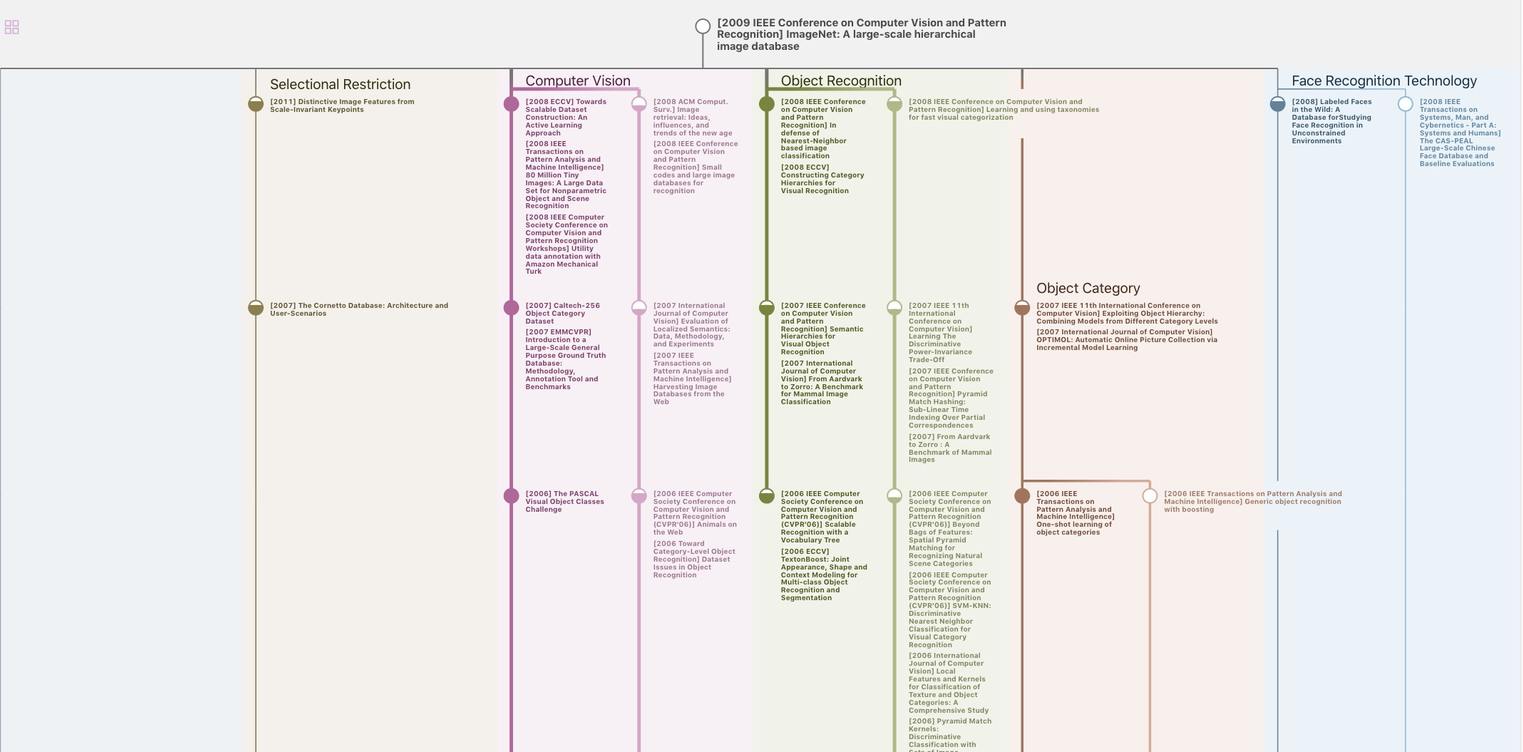
生成溯源树,研究论文发展脉络
Chat Paper
正在生成论文摘要