Too Much Information Kills Information: A Clustering Perspective
arxiv(2020)
摘要
Clustering is one of the most fundamental tools in the artificial intelligence area, particularly in the pattern recognition and learning theory. In this paper, we propose a simple, but novel approach for variance-based k-clustering tasks, included in which is the widely known k-means clustering. The proposed approach picks a sampling subset from the given dataset and makes decisions based on the data information in the subset only. With certain assumptions, the resulting clustering is provably good to estimate the optimum of the variance-based objective with high probability. Extensive experiments on synthetic datasets and real-world datasets show that to obtain competitive results compared with k-means method (Llyod 1982) and k-means++ method (Arthur and Vassilvitskii 2007), we only need 7% information of the dataset. If we have up to 15% information of the dataset, then our algorithm outperforms both the k-means method and k-means++ method in at least 80% of the clustering tasks, in terms of the quality of clustering. Also, an extended algorithm based on the same idea guarantees a balanced k-clustering result.
更多查看译文
关键词
much information kills information,clustering perspective
AI 理解论文
溯源树
样例
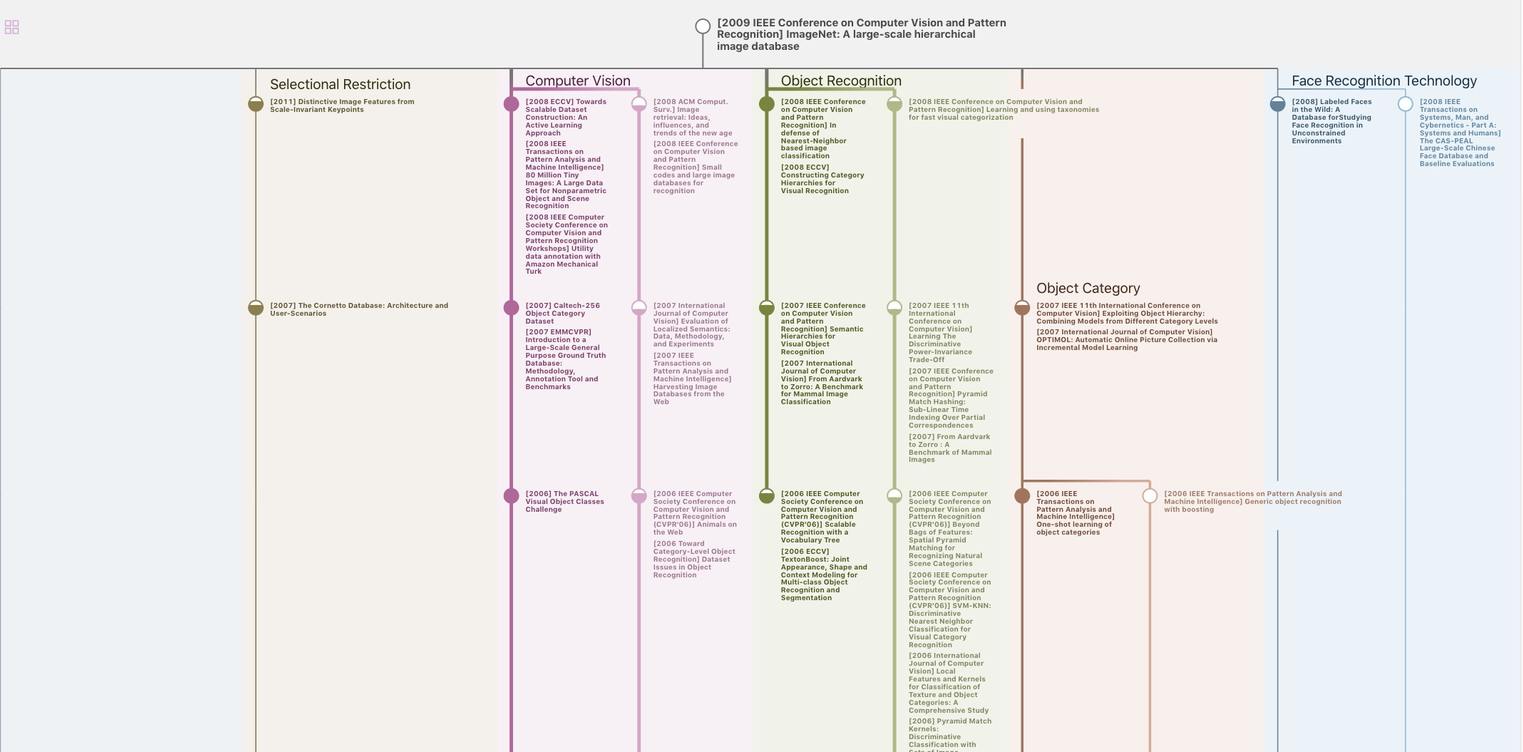
生成溯源树,研究论文发展脉络
Chat Paper
正在生成论文摘要