Design to Data for mutants of β-glucosidase B from Paenibacillus polymyxa: Q22T, W123R, F155G, Y169M, W438D, V401A
biorxiv(2019)
摘要
A key goal of protein engineering is to accurately model the stability and catalytic activity of enzymes. However, the limitations of functional predictive abilities pose a major challenge for modeling algorithm design, and can be attributed to the lack of large data sets quantifying the functional properties of enzymes. Here, the thermal stability (TM) and Michaelis-Menten constants ( k cat, KM, and k cat/KM) of six new variants of the β-glucosidase B (BglB) protein are quantitatively characterized. Molecular stability of the enzyme variants were hypothesized using the Foldit software and BglB was synthesized in E. coli cells. Testing was done through a colorimetric kinetic assay and thermal stability fluorescence-based protein unfolding assay. Results from the assays suggest that all mutations, with the exception of variant Y169M, all experienced reduced catalytic efficiency compared to the wildtype. Assay results indicate that variant W123R is more thermally stable compared to the wildtype, while the differences in thermal stability between the other variants, and the wildtype are negligible. The collected thermal stability and catalytic efficiency data has been added to a data set with the aim of improving Rosetta algorithms for modeling and predicting the functional interactions between biomolecules through a machine learning algorithm and facilitate the precise engineering of protein catalysts.
更多查看译文
AI 理解论文
溯源树
样例
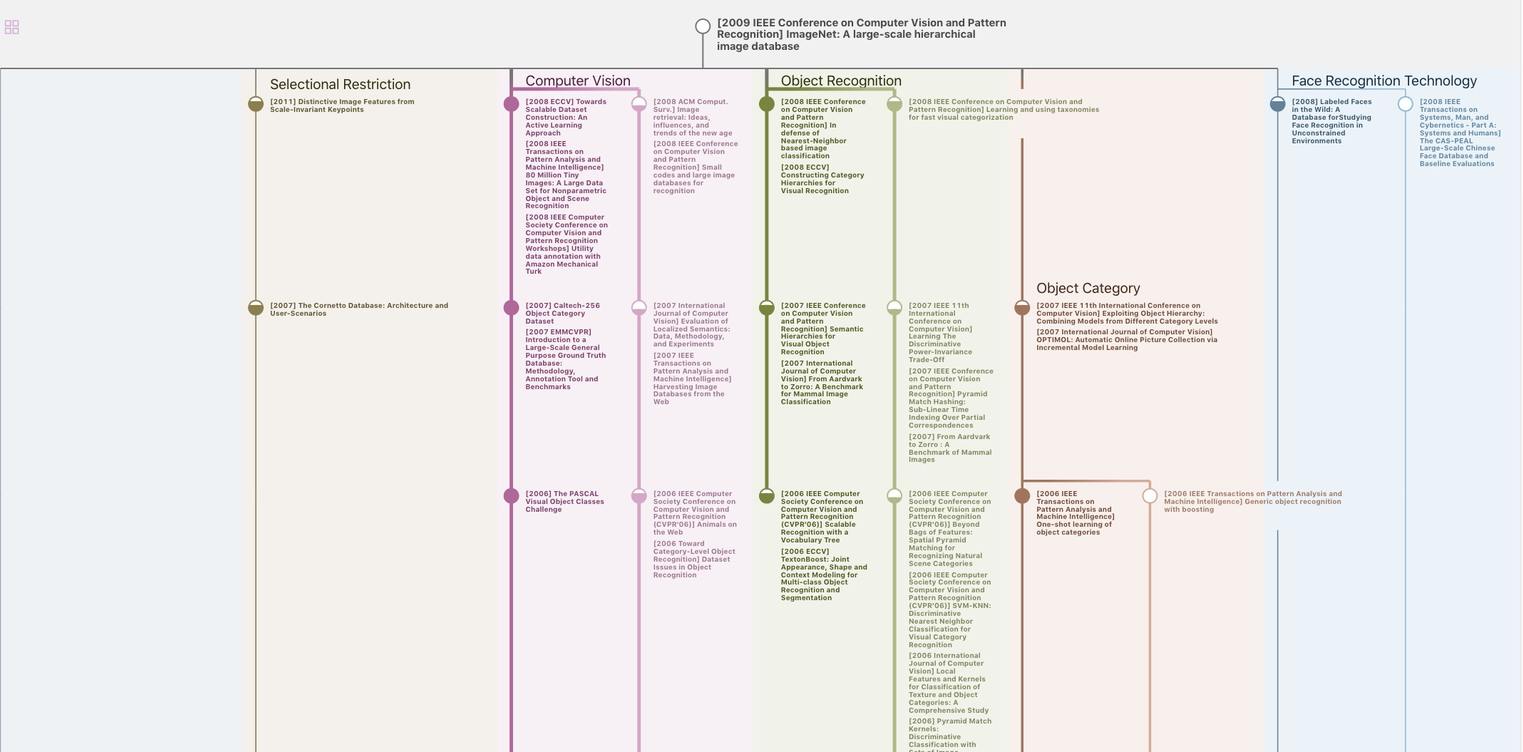
生成溯源树,研究论文发展脉络
Chat Paper
正在生成论文摘要