Arbitrary Video Style Transfer Via Multi-Channel Correlation
THIRTY-FIFTH AAAI CONFERENCE ON ARTIFICIAL INTELLIGENCE, THIRTY-THIRD CONFERENCE ON INNOVATIVE APPLICATIONS OF ARTIFICIAL INTELLIGENCE AND THE ELEVENTH SYMPOSIUM ON EDUCATIONAL ADVANCES IN ARTIFICIAL INTELLIGENCE(2021)
摘要
Video style transfer is attracting increasing attention from the artificial intelligence community because of its numerous applications, such as augmented reality and animation production. Relative to traditional image style transfer, video style transfer presents new challenges, including how to effectively generate satisfactory stylized results for any specified style while maintaining temporal coherence across frames. Towards this end, we propose a Multi-Channel Correlation network (MCCNet), which can be trained to fuse exemplar style features and input content features for efficient style transfer while naturally maintaining the coherence of input videos to output videos. Specifically, MCCNet works directly on the feature space of style and content domain where it learns to rearrange and fuse style features on the basis of their similarity to content features. The outputs generated by MCC are features containing the desired style patterns that can further be decoded into images with vivid style textures. Moreover, MCCNet is also designed to explicitly align the features to input and thereby ensure that the outputs maintain the content structures and the temporal continuity. To further improve the performance of MCCNet under complex light conditions, we also introduce illumination loss during training. Qualitative and quantitative evaluations demonstrate that MCCNet performs well in arbitrary video and image style transfer tasks. Code is available at https://github.com/diyiiyiii/MCCNet.
更多查看译文
关键词
arbitrary video style transfer,style transfer,correlation,multi-channel
AI 理解论文
溯源树
样例
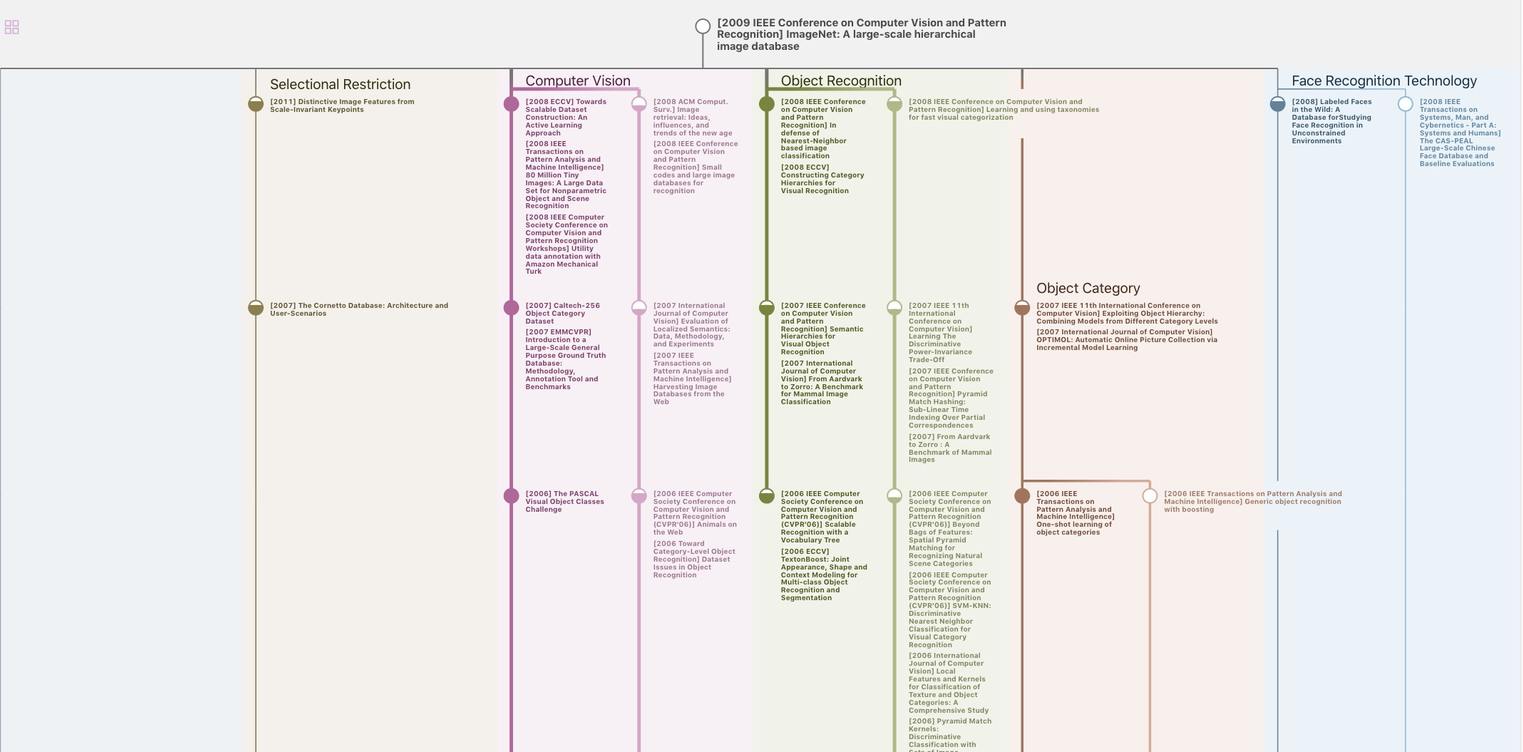
生成溯源树,研究论文发展脉络
Chat Paper
正在生成论文摘要