Biological machine learning combined with bacterial population genomics reveals common and rare allelic variants of genes to cause disease
biorxiv(2019)
摘要
Highly dimensional data generated from bacterial whole genome sequencing is providing unprecedented scale of information that requires appropriate statistical frameworks of analysis to infer biological function from bacterial genomic populations. Application of genome wide association study (GWAS) methods is an emerging approach with bacterial population genomics that yields a list of genes associated with a phenotype with an undefined importance among the candidates in the list. Here, we validate the combination of GWAS, machine learning, and pathogenic bacterial population genomics as a novel scheme to identify SNPs and rank allelic variants to determine associations for accurate estimation of disease phenotype. This approach parsed a dataset of 1.2 million SNPs that resulted in a ranked importance of associated alleles of using multiple spatial locations over a 30-year period. We validated this approach using previously proven laboratory experimental alleles from an guinea pig abortion model. This approach, termed BioML, defined intestinal and extraintestinal groups that have differential allelic variants that cause abortion. Divergent variants containing indels that defeated gene callers were rescued using biological context and knowledge that resulted in defining rare and divergent variants that were maintained in the population over two continents and 30 years. This study defines the capability of machine learning coupled to GWAS and population genomics to simultaneously identify and rank alleles to define their role in abortion, and more broadly infectious disease.
更多查看译文
关键词
Infectious disease,XGboost,<italic>Campylobacter</italic>,abortion,protein modeling
AI 理解论文
溯源树
样例
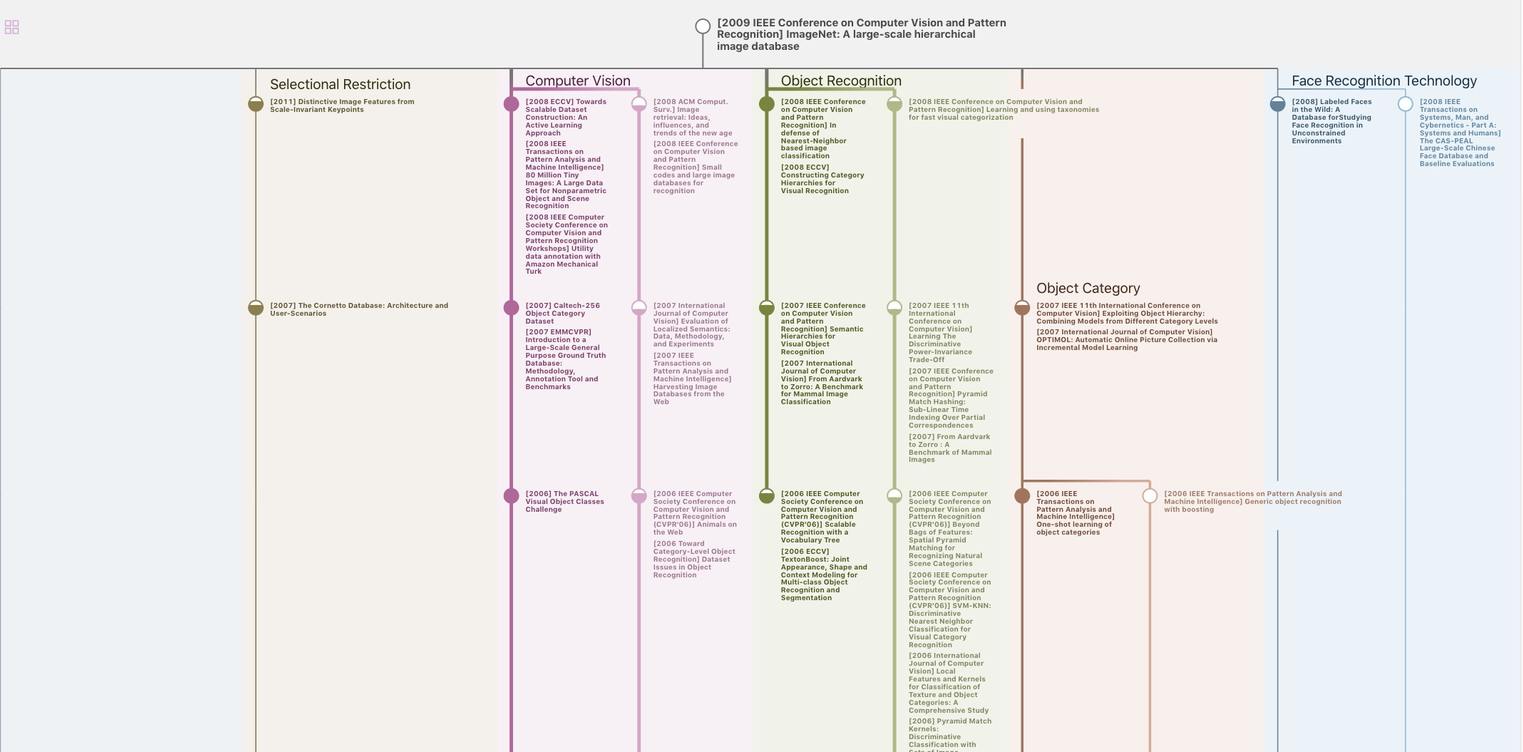
生成溯源树,研究论文发展脉络
Chat Paper
正在生成论文摘要