Gene set inference from single-cell sequencing data using a hybrid of matrix factorization and variational autoencoders
biorxiv(2020)
摘要
Recent advances in single-cell RNA sequencing have driven the simultaneous measurement of the expression of thousands of genes in thousands of single cells. These growing datasets allow us to model gene sets in biological networks at an unprecedented level of detail, in spite of heterogeneous cell populations. Here, we propose a deep neural network model that is a hybrid of matrix factorization and variational autoencoders, which we call restricted latent variational autoencoder (resVAE). The model uses weights as factorized matrices to obtain gene sets, while class-specific inputs to the latent variable space facilitate a plausible identification of cell types. This artificial neural network model seamlessly integrates functional gene set inference, experimental covariate effect isolation, and static gene identification, which we conceptually demonstrate here for four single-cell RNA sequencing datasets.
更多查看译文
关键词
Gene regulatory networks,Genome informatics,Machine learning,Engineering,general
AI 理解论文
溯源树
样例
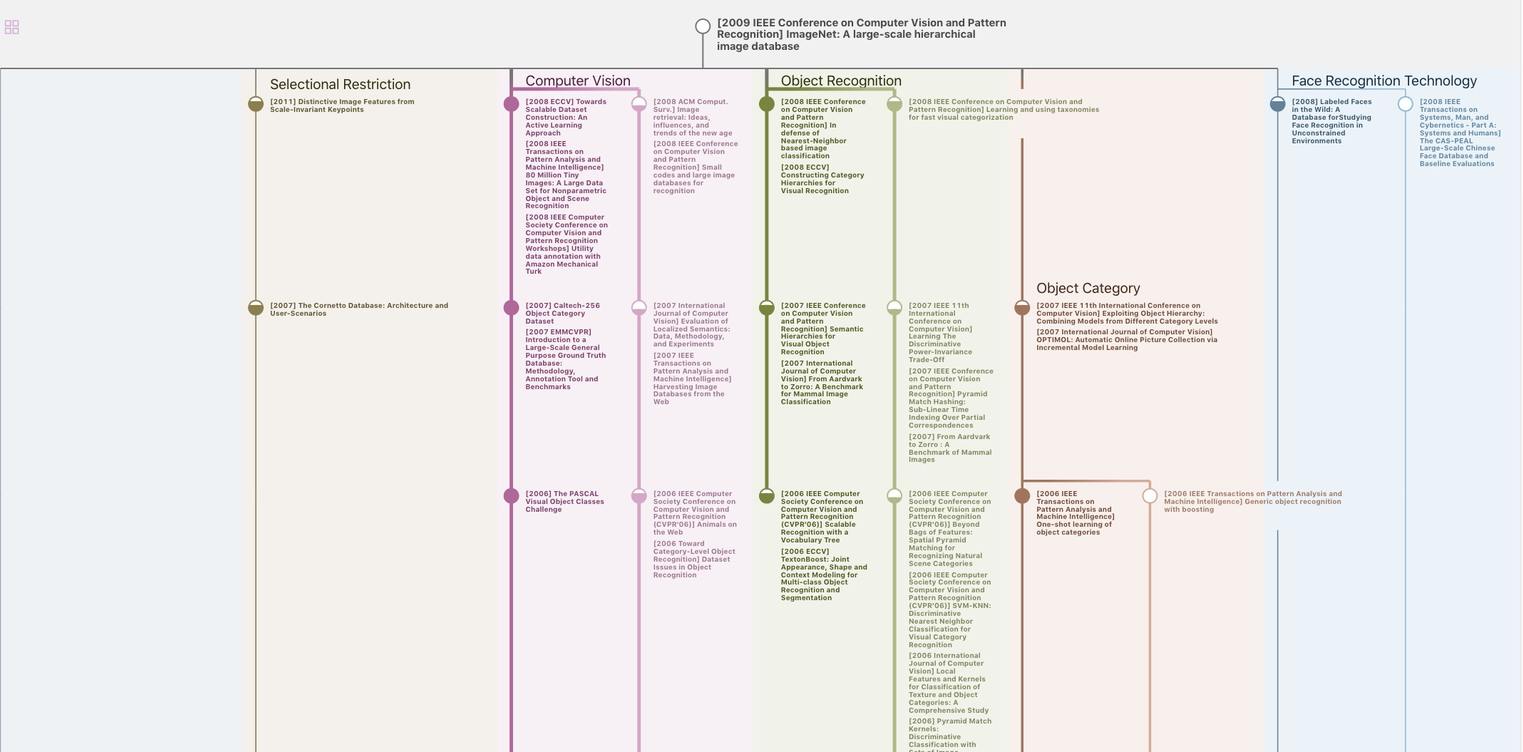
生成溯源树,研究论文发展脉络
Chat Paper
正在生成论文摘要