Impact of radiomics on prostate cancer detection: a systematic review of clinical applications.
CURRENT OPINION IN UROLOGY(2020)
摘要
Purpose of review To systematically review the current literature to assess the role of radiomics in the detection and evaluation of prostate cancer (PCa). Recent findings Radiomics involves the high-throughput extraction of radiologic features from clinical imaging, using a panel of sophisticated data-characterization algorithms to make an objective and quantitative determination of diagnoses and clinical characteristics. Radiomics evaluation of existing clinical images would increase their clinical value in many cancer management pathways, including PCa. However, a consensus on the implementation of radiomics has not been established across different sites, delaying its implementation in clinical practice. There are many potential advantages to radiomics. The ability to extract features from existing clinical imaging is one such advantage. A second is the empiric nature of the analysis. The third lies in the application of new technologies, such as machine learning, to be able to evaluate large quantities of data to make clinical conclusions. In this systematic review, we identify publications regarding the role of radiomics in PCa detection and evaluation. Many of these studies noted that radiomics, when incorporated into predictive models, had an advantageous impact on detection of PCa, clinically significant PCa, and extracapsular extension. This may assist in individualized decision making not only for diagnosis of PCa, but also for surveillance and surgical planning. With additional validation in large sample sizes, and randomized, multicenter studies using a consensus driven methodology, radiomics has the potential to alter the landscape of PCa detection and management, necessitating further prospective randomized investigation. Radiomics is a promising new field, allowing for high-throughput analysis of imaging features for PCa detection and evaluation. These features can be extracted from existing data; therefore, the potential for future study is immense.
更多查看译文
关键词
early detection of cancer,machine learning,prostatic neoplasms,radiologic,technology
AI 理解论文
溯源树
样例
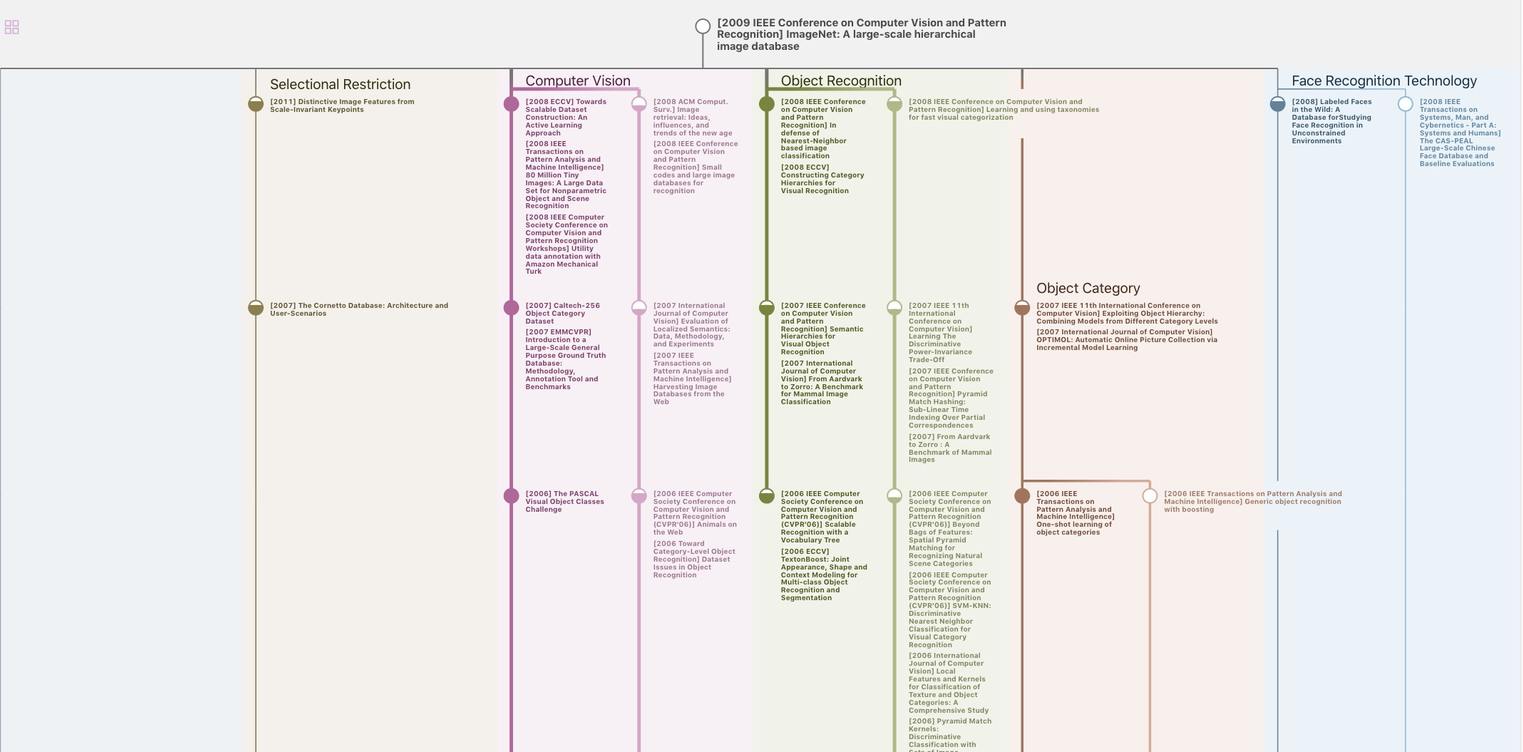
生成溯源树,研究论文发展脉络
Chat Paper
正在生成论文摘要