A Deep Learning approach predicts the impact of point mutations in intronic flanking regions on micro-exon splicing definition
biorxiv(2019)
摘要
While mammalian exons are on average 140-nt-long, thousands of human genes harbor micro-exons (≤ 39 nt). Large numbers of micro-exons have their splicing altered in diseases such as autism and cancer, and yet there is no systematic assessment of the impact of point mutations in intronic flanking-sequences on the splicing of a neighboring micro-exon. Here, we constructed a model using the Convolutional Neural Network (CNN) to predict the impact of point mutations in intronic-flanking-sequences on the splicing of a neighboring micro-exon. The prediction model was based on both the sequence contents and conservation among species of the two 100-nt intronic regions (5’ and 3’) that flank all human micro-exons and a set with the same number of randomly selected long exons. After training our CNN model, the micro-exon splicing event prediction accuracy, using an independent validation dataset, was 0.71 with an area under the ROC curve of 0.76, showing that our model had identified sequence patterns that have been conserved in evolution in the introns that flank micro-exons. Next, we introduced point mutations at each of the 200 nucleotides in the introns that flank a micro-exon and used the trained CNN algorithm to predict splicing for every mutated intronic sequence version. This analysis identified thousands of point mutations in the flanking introns that significantly decreased the power of the CNN model to correctly predict a neighboring micro-exon splicing event, thus pointing to predictive bases in intronic regions important for micro-exon splicing signaling. We found these predictive bases to locate within conserved RNA-binding-motifs for RNA-binding-proteins (RBPs) known to relate to micro-exon splicing. Experimental data of minigene splicing reporter changes upon intron-base point-mutation confirmed the effect predicted by the CNN model for some of the micro-exon splicing events. The model can be used for validating novel micro-exons assembled from RNA-seq data, and for an unbiased screening of introns, identifying genomic bases that have high micro-exon-splicing predictive power, possibly revealing critical point mutations that would be related in a yet unknown manner to a given disease.
更多查看译文
关键词
micro-exon splicing,Convolutional Neural Network (CNN),deep learning,in silico point mutation screening,micro-exon splicing prediction,predictive conserved base identification,intron sequence conservation,enriched RNA-binding-motifs.
AI 理解论文
溯源树
样例
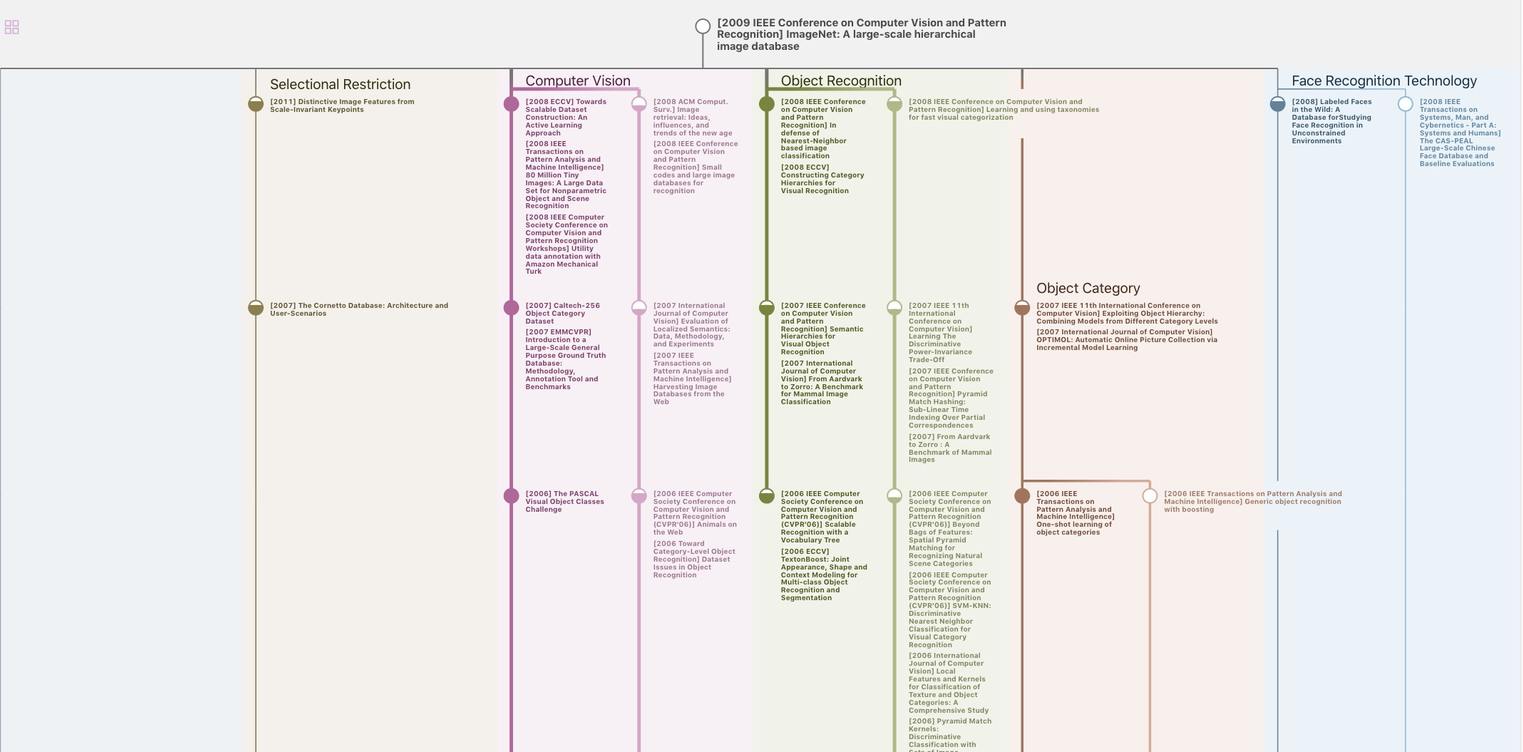
生成溯源树,研究论文发展脉络
Chat Paper
正在生成论文摘要