End-to-end multitask learning, from protein language to protein features without alignments
biorxiv(2020)
摘要
Correctly predicting features of protein structure and function from amino acid sequence alone remains a supreme challenge for computational biology. For almost three decades, state-of-the-art approaches combined machine learning and evolutionary information from multiple sequence alignments. Exponentially growing sequence databases make it infeasible to gather evolutionary information for entire microbiomes or meta-proteomics. On top, for many important proteins (e.g. and ) evolutionary information remains limited. Here, we introduced a novel approach combining recent advances of Language Models (LMs) with multi-task learning to successfully predict aspects of protein structure (secondary structure) and function (cellular component or subcellular localization) without using any evolutionary information from alignments. Our approach fused self-supervised pre-training LMs on an unlabeled big dataset (UniRef50, corresponding to 9.6 billion words) with supervised training on labelled high-quality data in one single end-to-end network. We provided a proof-of-principle for the novel concept through the semi-successful per-residue prediction of protein secondary structure and through per-protein predictions of localization (Q10=69%) and the distinction between integral membrane and water-soluble proteins (Q2=89%). Although these results did not reach the levels obtained by the best available methods using evolutionary information from alignments, these less accurate multi-task predictions have the advantage of speed: they are 300-3000 times faster (where HHblits needs 30-300 seconds on average, our method needed 0.045 seconds). These new results push the boundaries of predictability towards grayer and darker areas of the protein space, allowing to make reliable predictions for proteins which were not accessible by previous methods. On top, our method remains scalable as it removes the necessity to search sequence databases for evolutionary related proteins.
更多查看译文
关键词
Machine Learning,Language Modelling,Semi-Supervised Learning,Multi-Task Learning,Protein Secondary Structure Prediction,Protein subcellular-localization Prediction
AI 理解论文
溯源树
样例
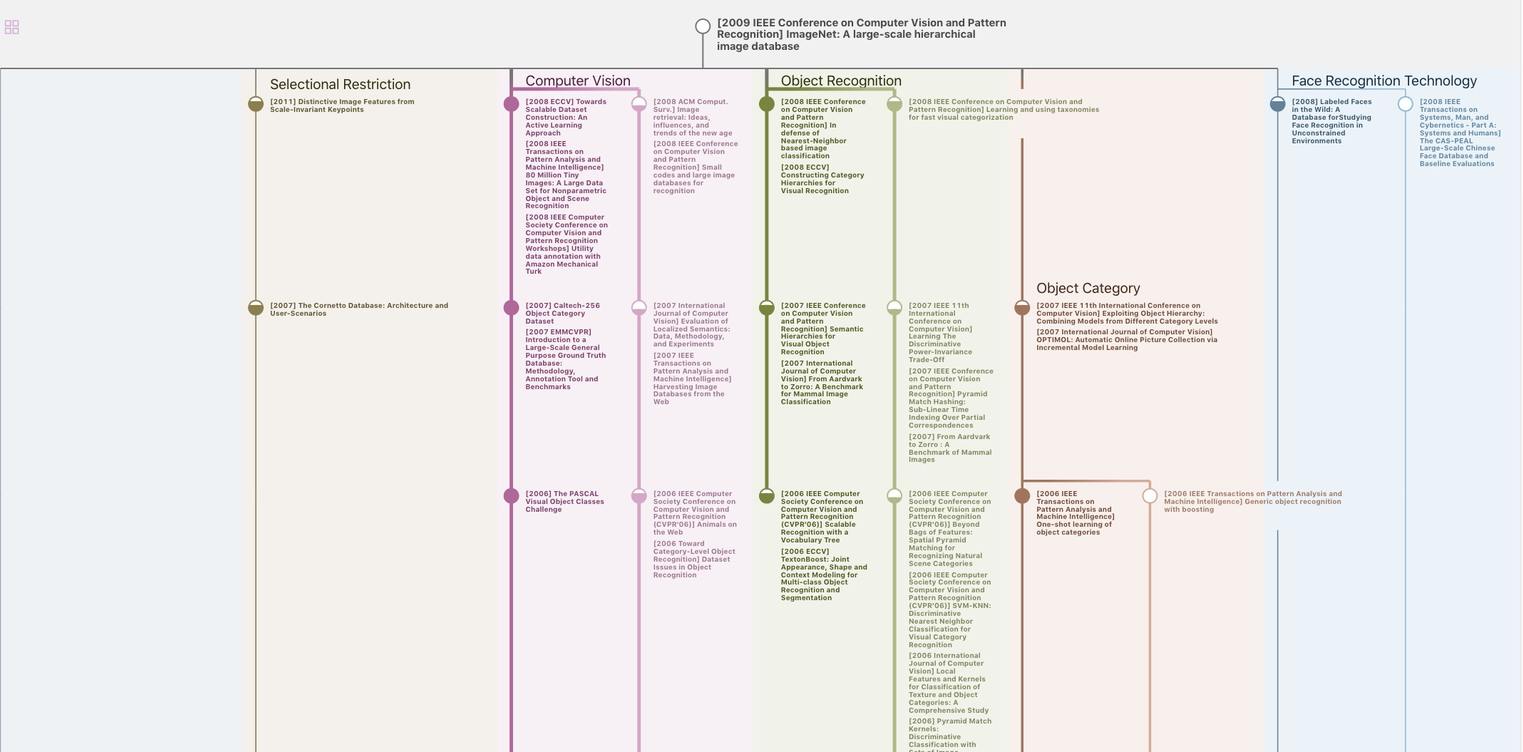
生成溯源树,研究论文发展脉络
Chat Paper
正在生成论文摘要