Real-time artificial intelligence-based histologic classification of colorectal polyps with augmented visualization.
Gastrointestinal endoscopy(2020)
摘要
BACKGROUND AND AIMS:Artificial intelligence (AI)-based computer-aided diagnostic (CADx) algorithms are a promising approach for real-time histology (RTH) of colonic polyps. Our aim is to present a novel in situ CADx approach that seeks to increase transparency and interpretability of results by generating an intuitive augmented visualization of the model's predicted histology over the polyp surface.
METHODS:We developed a deep learning model using semantic segmentation to delineate polyp boundaries and a deep learning model to classify subregions within the segmented polyp. These subregions were classified independently and were subsequently aggregated to generate a histology map of the polyp's surface. We used 740 high-magnification narrow-band images from 607 polyps in 286 patients and over 65,000 subregions to train and validate the model.
RESULTS:The model achieved a sensitivity of .96, specificity of .84, negative predictive value (NPV) of .91, and high-confidence rate (HCR) of .88, distinguishing 171 neoplastic polyps from 83 non-neoplastic polyps of all sizes. Among 93 neoplastic and 75 non-neoplastic polyps ≤5 mm, the model achieved a sensitivity of .95, specificity of .84, NPV of .91, and HCR of .86.
CONCLUSIONS:The CADx model is capable of accurately distinguishing neoplastic from non-neoplastic polyps and provides a histology map of the spatial distribution of localized histologic predictions along the delineated polyp surface. This capability may improve interpretability and transparency of AI-based RTH and offer intuitive, accurate, and user-friendly guidance in real time for the clinical management and documentation of optical histology results.
更多查看译文
AI 理解论文
溯源树
样例
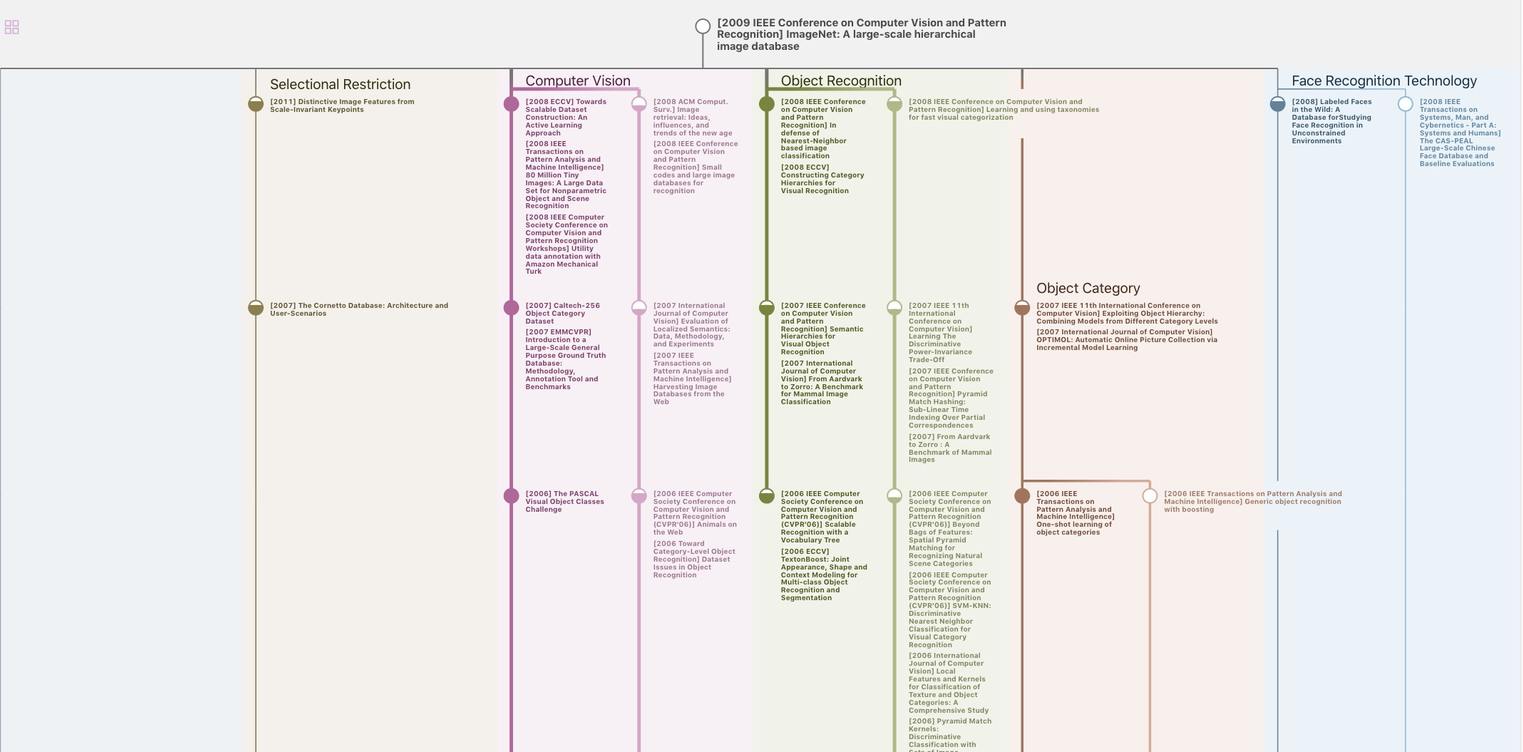
生成溯源树,研究论文发展脉络
Chat Paper
正在生成论文摘要