Generation-Augmented Retrieval for Open-Domain Question Answering.
59TH ANNUAL MEETING OF THE ASSOCIATION FOR COMPUTATIONAL LINGUISTICS AND THE 11TH INTERNATIONAL JOINT CONFERENCE ON NATURAL LANGUAGE PROCESSING (ACL-IJCNLP 2021), VOL 1(2021)
摘要
We propose Generation-Augmented Retrieval (GAR) for answering open-domain questions, which augments a query through text generation of heuristically discovered relevant contexts without external resources as supervision. We demonstrate that the generated contexts substantially enrich the semantics of the queries and GAR with sparse representations (BM25) achieves comparable or better performance than state-of-the-art dense retrieval methods such as DPR (Karpukhin et al., 2020). We show that generating diverse contexts for a query is beneficial as fusing their results consistently yields better retrieval accuracy. Moreover, as sparse and dense representations are often complementary, GAR can be easily combined with DPR to achieve even better performance. GAR achieves state-of-the-art performance on Natural Questions and TriviaQA datasets under the extractive QA setup when equipped with an extractive reader, and consistently outperforms other retrieval methods when the same generative reader is used.
更多查看译文
关键词
retrieval,generation-augmented,open-domain
AI 理解论文
溯源树
样例
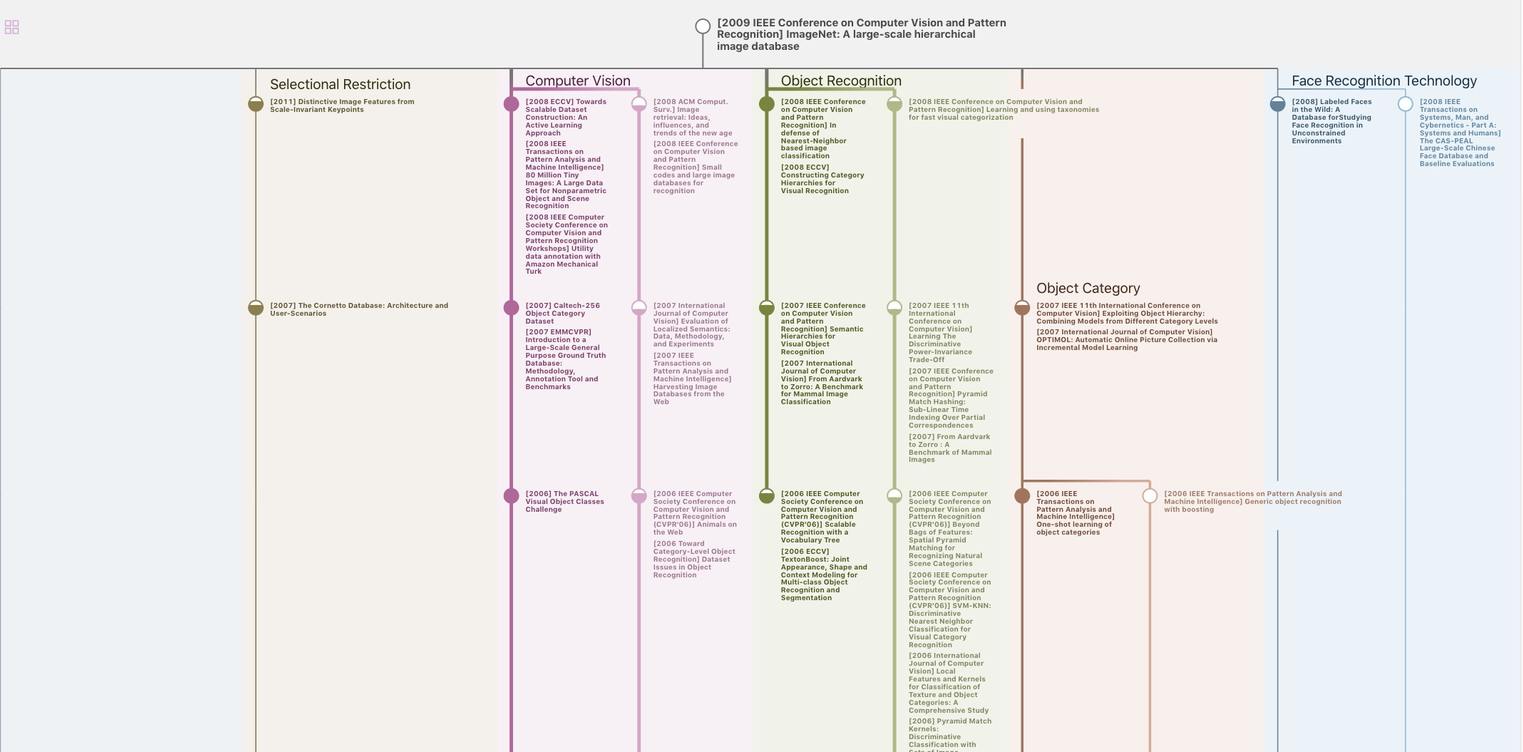
生成溯源树,研究论文发展脉络
Chat Paper
正在生成论文摘要