Reinforcement Learning for Weakly Supervised Temporal Grounding of Natural Language in Untrimmed Videos
MM '20: The 28th ACM International Conference on Multimedia Seattle WA USA October, 2020(2020)
摘要
Temporal grounding of natural language in untrimmed videos is a fundamental yet challenging multimedia task facilitating cross-media visual content retrieval. We focus on the weakly supervised setting of this task that merely accesses to coarse video-level language description annotation without temporal boundary, which is more consistent with reality as such weak labels are more readily available in practice. In this paper, we propose a Boundary Adaptive Refinement (BAR) framework that resorts to reinforcement learning (RL) to guide the process of progressively refining the temporal boundary. To the best of our knowledge, we offer the first attempt to extend RL to temporal localization task with weak supervision. As it is non-trivial to obtain a straightforward reward function in the absence of pairwise granular boundary-query annotations, a cross-modal alignment evaluator is crafted to measure the alignment degree of segment-query pair to provide tailor-designed rewards. This refinement scheme completely abandons traditional sliding window based solution pattern and contributes to acquiring more efficient, boundary-flexible and content-aware grounding results. Extensive experiments on two public benchmarks Charades-STA and ActivityNet demonstrate that BAR outperforms the state-of-the-art weakly-supervised method and even beats some competitive fully-supervised ones.
更多查看译文
关键词
Temporal grounding of natural language in untrimmed videos, Reinforcement learning, Boundary adaptive refinement
AI 理解论文
溯源树
样例
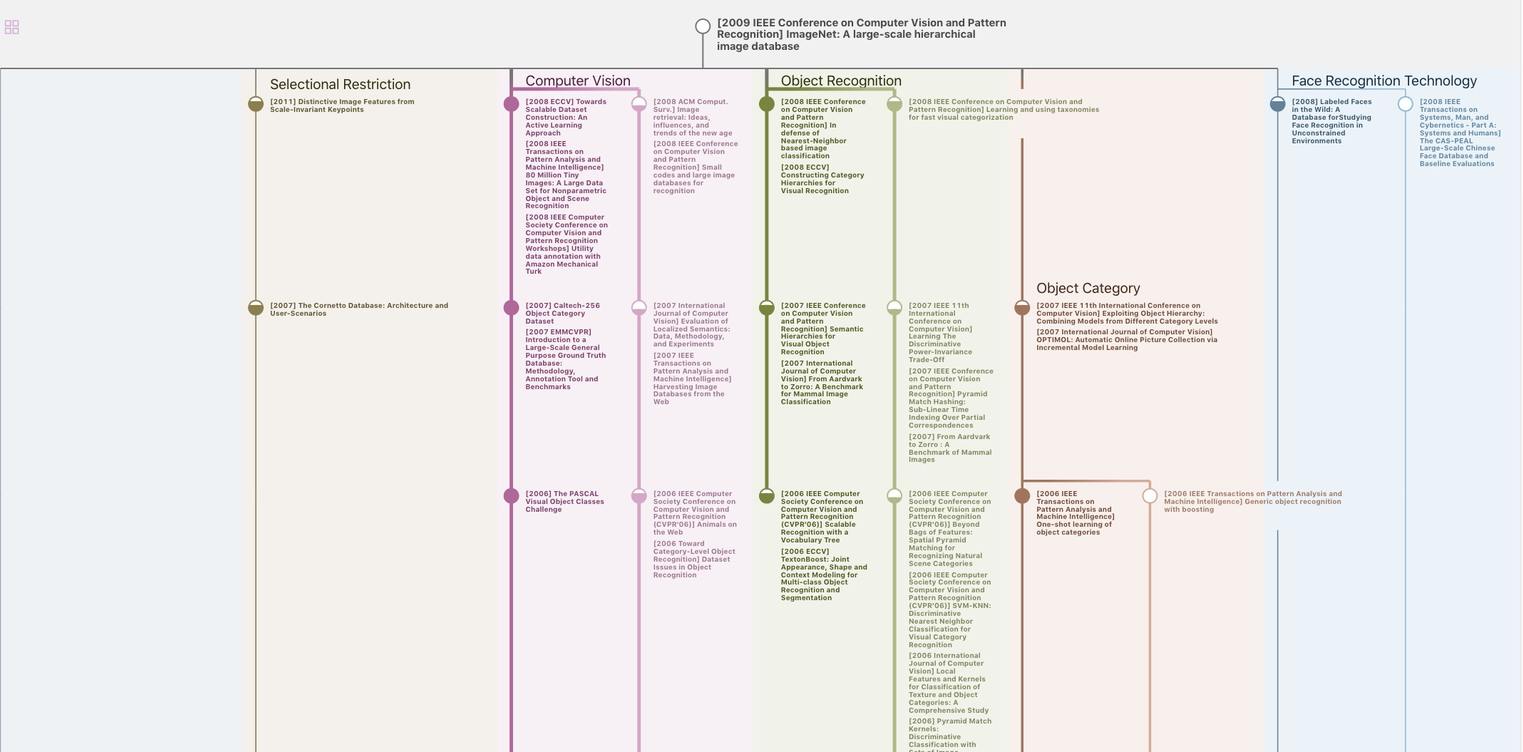
生成溯源树,研究论文发展脉络
Chat Paper
正在生成论文摘要