Progressive Semantic-Aware Style Transformation for Blind Face Restoration
2021 IEEE/CVF CONFERENCE ON COMPUTER VISION AND PATTERN RECOGNITION, CVPR 2021(2021)
摘要
Face restoration is important in face image processing, and has been widely studied in recent years. However, previous works often fail to generate plausible high quality (HQ) results for real-world low quality (LQ) face images. In this paper, we propose a new progressive semantic-aware style transformation framework, named PSFR-GAN, for face restoration. Specifically, instead of using an encoder-decoder framework as previous methods, we formulate the restoration of LQ face images as a multi-scale progressive restoration procedure through semantic-aware style transformation. Given a pair of LQ face image and its corresponding parsing map, we first generate a multi-scale pyramid of the inputs, and then progressively modulate different scale features from coarse-to-fine in a semantic-aware style transfer way. Compared with previous networks, the proposed PSFR-GAN makes full use of the semantic (parsing maps) and pixel (LQ images) space information from different scales of input pairs. In addition, we further introduce a semantic aware style loss which calculates the feature style loss for each semantic region individually to improve the details of face textures. Finally, we pretrain a face parsing network which can generate decent parsing maps from real-world LQ face images. Experiment results show that our model trained with synthetic data can not only produce more realistic high-resolution results for synthetic LQ inputs but also generalize better to natural LQ face images compared with state-of-the-art methods.
更多查看译文
关键词
blind face restoration,face image processing,plausible high quality results,real-world low quality face images,semantic-aware style transformation framework,named PSFR-GAN,encoder-decoder framework,LQ face image,multiscale progressive restoration procedure,corresponding parsing map,multiscale pyramid,different scale features,previous networks,LQ images,semantic aware style loss,feature style loss,semantic region,face textures,face parsing network,decent parsing maps,real-world LQ face images,synthetic LQ inputs,natural LQ face images
AI 理解论文
溯源树
样例
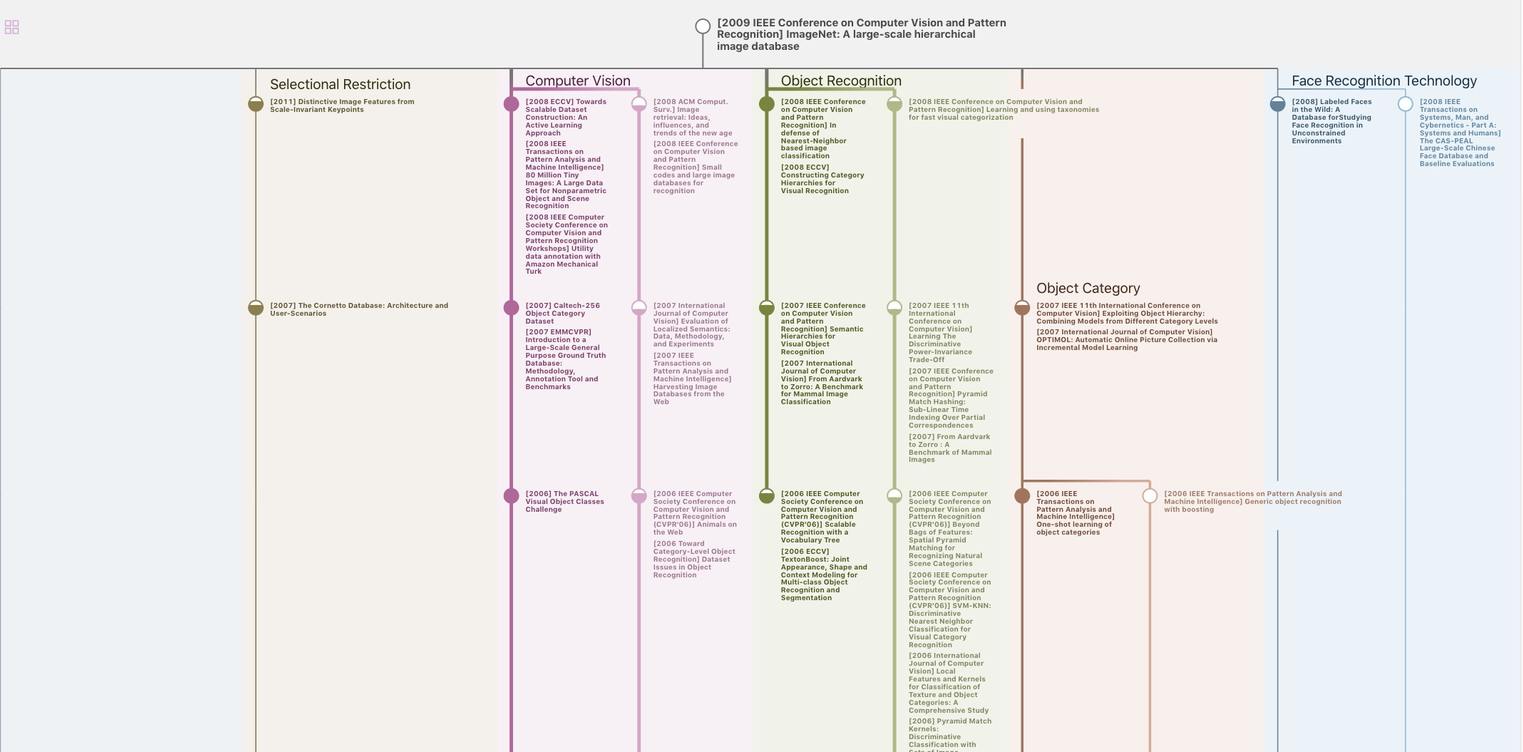
生成溯源树,研究论文发展脉络
Chat Paper
正在生成论文摘要