Forecasting time series with encoder-decoder neural networks
arxiv(2020)
摘要
In this paper, we consider high-dimensional stationary processes where a new observation is generated from a compressed version of past observations. The specific evolution is modeled by an encoder-decoder structure. We estimate the evolution with an encoder-decoder neural network and give upper bounds for the expected forecast error under specific structural and sparsity assumptions. The results are shown separately for conditions either on the absolutely regular mixing coefficients or the functional dependence measure of the observed process. In a quantitative simulation we discuss the behavior of the network estimator under different model assumptions. We corroborate our theory by a real data example where we consider forecasting temperature data.
更多查看译文
关键词
neural networks,series,encoder-decoder
AI 理解论文
溯源树
样例
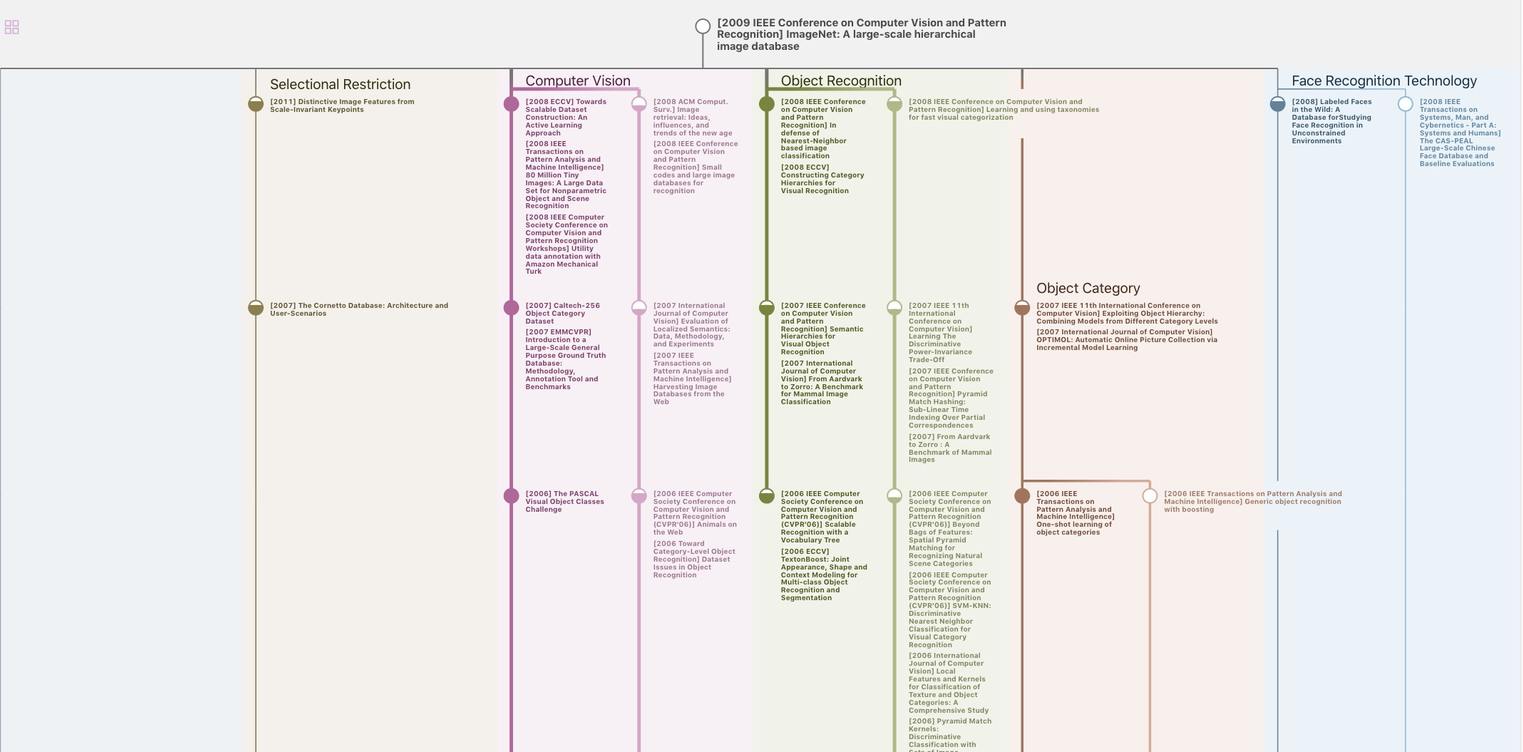
生成溯源树,研究论文发展脉络
Chat Paper
正在生成论文摘要