Cellbow: a robust customizable cell segmentation program
QUANTITATIVE BIOLOGY(2020)
摘要
Background Time-lapse live cell imaging of a growing cell population is routine in many biological investigations. A major challenge in imaging analysis is accurate segmentation, a process to define the boundaries of cells based on raw image data. Current segmentation methods relying on single boundary features have problems in robustness when dealing with inhomogeneous foci which invariably happens in cell population imaging. Methods Combined with a multi-layer training set strategy, we developed a neural-network-based algorithm — Cellbow. Results Cellbow can achieve accurate and robust segmentation of cells in broad and general settings. It can also facilitate long-term tracking of cell growth and division. To facilitate the application of Cellbow, we provide a website on which one can online test the software, as well as an ImageJ plugin for the user to visualize the performance before software installation. Conclusions Cellbow is customizable and generalizable. It is broadly applicable to segmenting fluorescent images of diverse cell types with no further training needed. For bright-field images, only a small set of sample images of the specific cell type from the user may be needed for training.
更多查看译文
关键词
deep neural network,cell segmentation,fluorescent cell imaging,bright-field cell imaging,lineage tracking
AI 理解论文
溯源树
样例
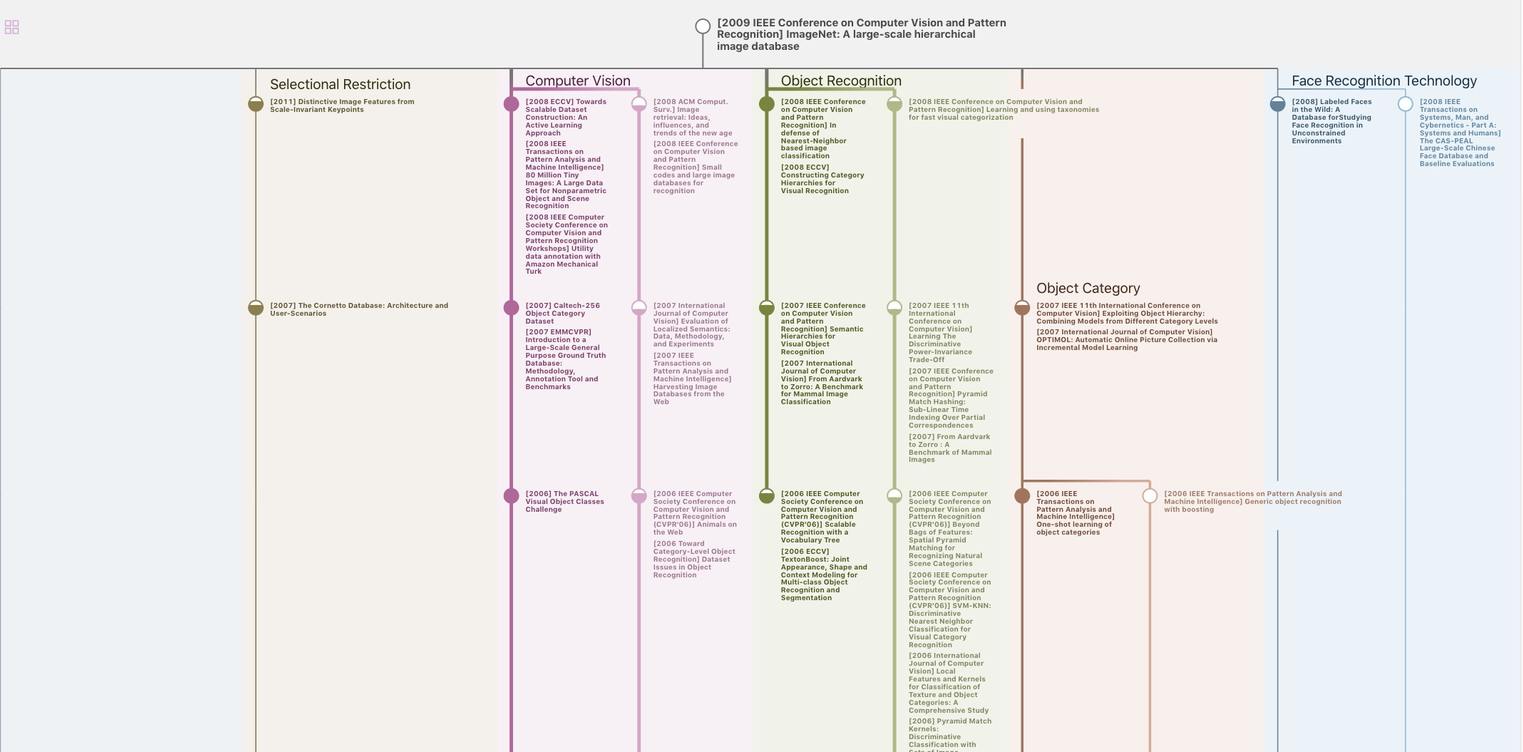
生成溯源树,研究论文发展脉络
Chat Paper
正在生成论文摘要