Tradeoffs in Sentence Selection Techniques for Open-Domain Question Answering
arxiv(2020)
摘要
Current methods in open-domain question answering (QA) usually employ a pipeline of first retrieving relevant documents, then applying strong reading comprehension (RC) models to that retrieved text. However, modern RC models are complex and expensive to run, so techniques to prune the space of retrieved text are critical to allow this approach to scale. In this paper, we focus on approaches which apply an intermediate sentence selection step to address this issue, and investigate the best practices for this approach. We describe two groups of models for sentence selection: QA-based approaches, which run a full-fledged QA system to identify answer candidates, and retrieval-based models, which find parts of each passage specifically related to each question. We examine trade-offs between processing speed and task performance in these two approaches, and demonstrate an ensemble module that represents a hybrid of the two. From experiments on Open-SQuAD and TriviaQA, we show that very lightweight QA models can do well at this task, but retrieval-based models are faster still. An ensemble module we describe balances between the two and generalizes well cross-domain.
更多查看译文
关键词
sentence selection techniques,open-domain
AI 理解论文
溯源树
样例
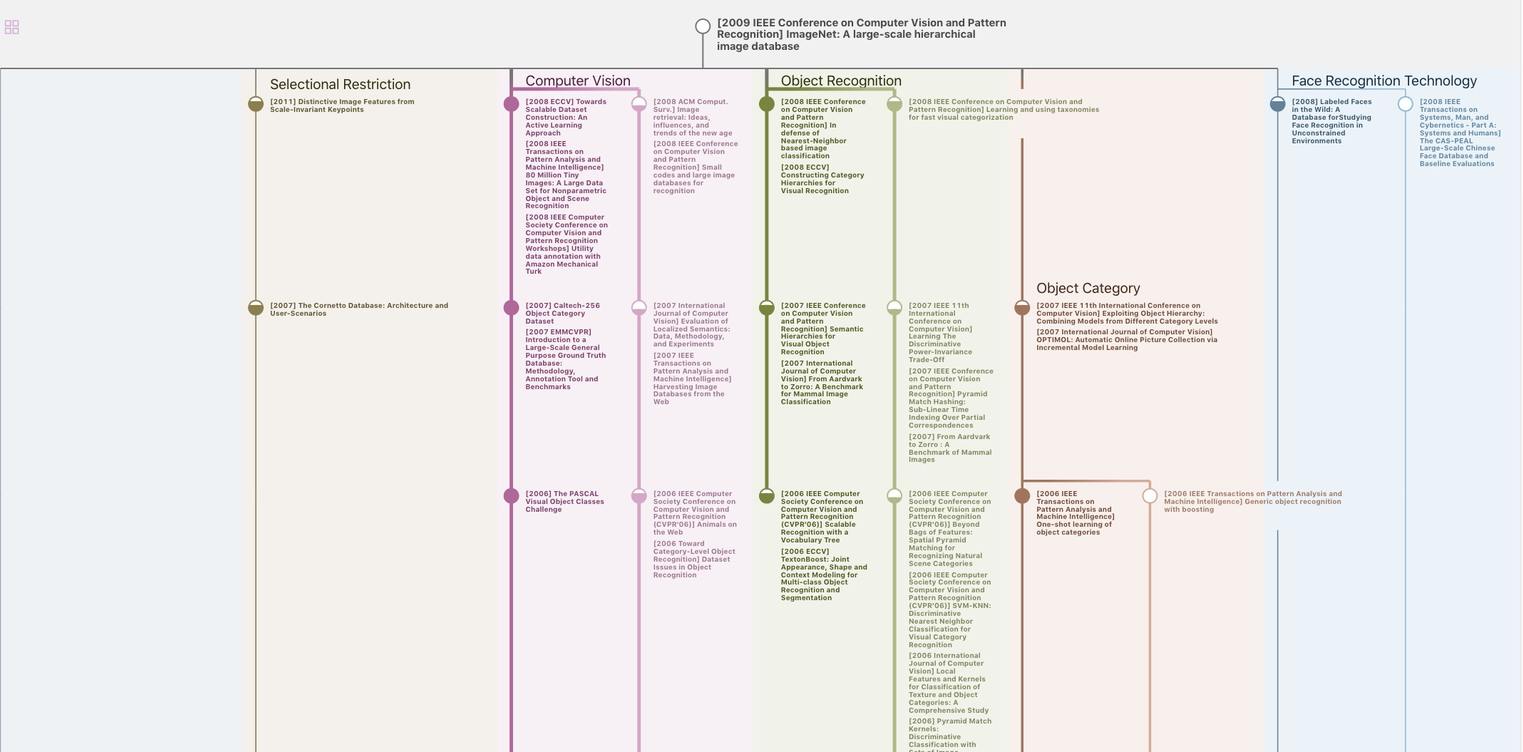
生成溯源树,研究论文发展脉络
Chat Paper
正在生成论文摘要