Inductive Learning on Commonsense Knowledge Graph Completion
2021 INTERNATIONAL JOINT CONFERENCE ON NEURAL NETWORKS (IJCNN)(2021)
摘要
Commonsense knowledge graph (CKG) is a special type of knowledge graph (KG), where entities are composed of free-form text. Existing CKG completion methods focus on transductive learning setting, where all the entities are present during training. Here, we propose the first inductive learning setting for CKG completion, where unseen entities may appear at test time. We emphasize that the inductive learning setting is crucial for CKGs, because unseen entities are frequently introduced due to the fact that CKGs are dynamic and highly sparse. We propose InductivE as the first framework targeted at the inductive CKG completion task. InductivE first ensures the inductive learning capability by directly computing entity embeddings from raw entity attributes. Second, a graph neural network with novel densification process is proposed to further enhance unseen entity representation with neighboring structural information. Experimental results show that InductivE performs especially well on inductive scenarios where it achieves above 48% improvement over previous methods while also outperforms state-of-the-art baselines in transductive settings.
更多查看译文
关键词
Commonsense Knowledge Graph, Inductive Learning, Graph Learning, Knowledge Graph Completion
AI 理解论文
溯源树
样例
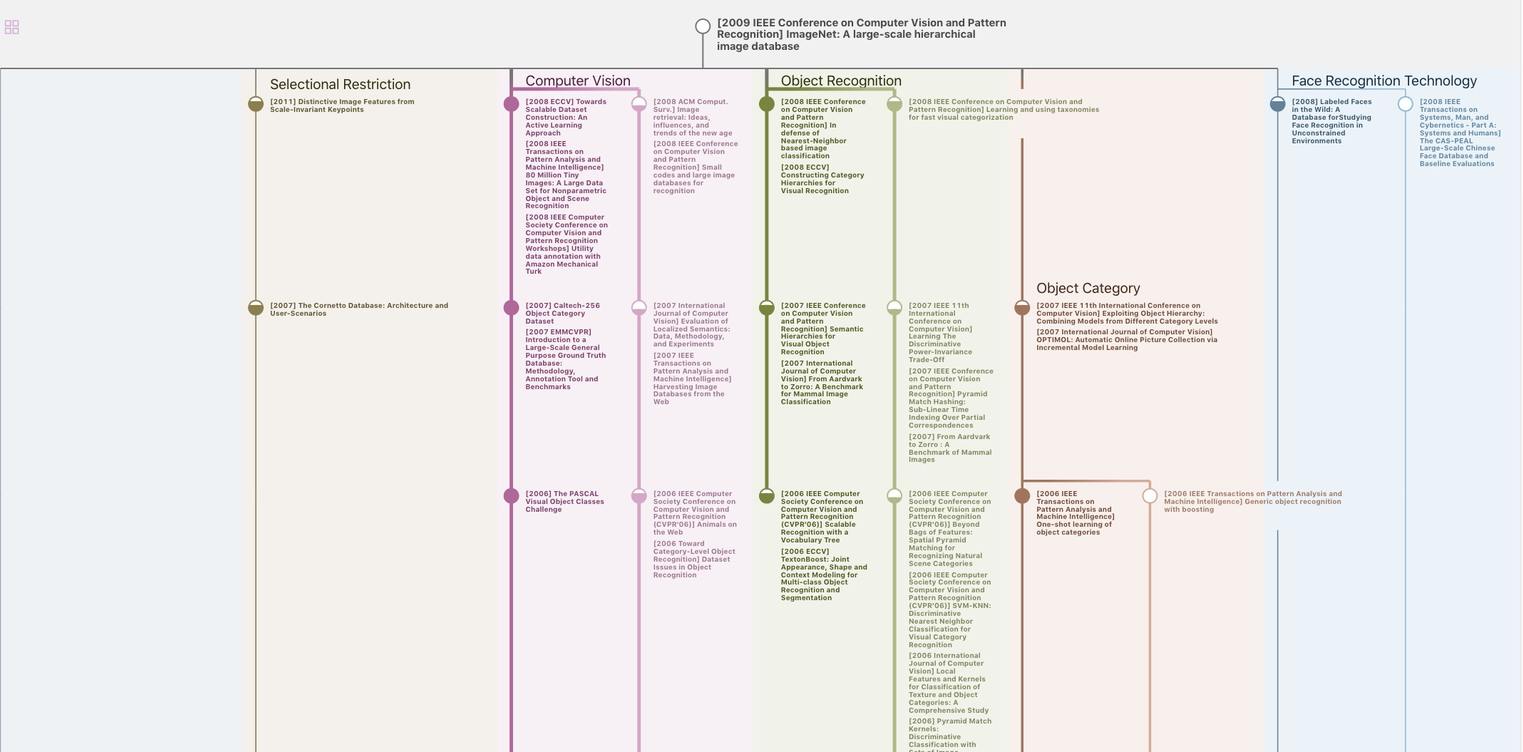
生成溯源树,研究论文发展脉络
Chat Paper
正在生成论文摘要