Mobile Cellular-Connected UAVs: Reinforcement Learning for Sky Limits.
2020 IEEE GLOBECOM WORKSHOPS (GC WKSHPS)(2020)
摘要
A cellular-connected unmanned aerial vehicle (UAV) faces several key challenges concerning connectivity and energy efficiency. Through a learning-based strategy, we propose a general novel multi-armed bandit (MAB) algorithm to reduce disconnectivity time, handover rate, and energy consumption of UAV by taking into account its time of task completion. By formulating the problem as a function of UAV's velocity, we show how each of these performance indicators (PIs) is improved by adopting a proper range of corresponding learning parameter, e.g. 50% reduction in HO rate as compared to a blind strategy. However, results reveal that the optimal combination of the learning parameters depends critically on any specific application and the weights of PIs on the final objective function.
更多查看译文
关键词
Reinforcement learning, multi-armed bandit, unmanned aerial vehicle (UAV), cellular networks, handover rate, energy efficiency
AI 理解论文
溯源树
样例
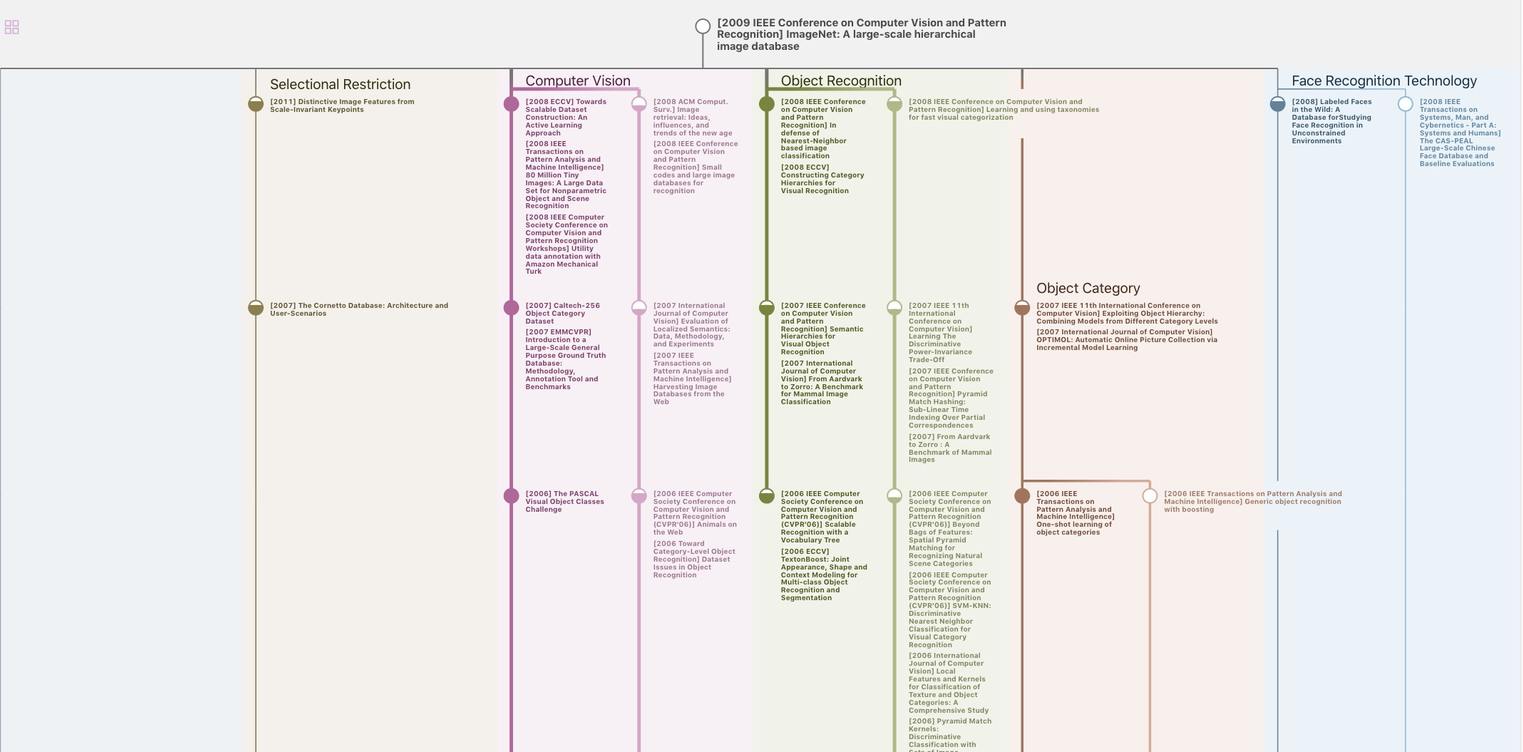
生成溯源树,研究论文发展脉络
Chat Paper
正在生成论文摘要