Computational Prediction of Drug-Disease Association Based on Graph-Regularized One Bit Matrix Completion.
biorxiv(2022)
摘要
Investigation of existing drugs is an effective alternative to the discovery of new drugs for treating diseases. This task of drug re-positioning can be assisted by various kinds of computational methods to predict the best indication for a drug given the open-source biological datasets. Owing to the fact that similar drugs tend to have common pathways and disease indications, the association matrix is assumed to be of low-rank structure. Hence, the problem of drug-disease association prediction can be modeled as a low-rank matrix completion problem. In this work, we propose a novel matrix completion framework that makes use of the side-information associated with drugs/diseases for the prediction of drug-disease indications modeled as neighborhood graph: Graph regularized 1-bit matrix completion (GR1BMC). The algorithm is specially designed for binary data and uses parallel proximal algorithm to solve the aforesaid minimization problem taking into account all the constraints including the neighborhood graph incorporation and restricting predicted scores within the specified range. The results have been validated on two standard databases by evaluating the AUC across the 10-fold cross-validation splits. The usage of the method is also evaluated through a case study where top 5 indications are predicted for novel drugs, which then are verified with the CTD database.
更多查看译文
关键词
Drug-disease association,graph regularization,matrix completion
AI 理解论文
溯源树
样例
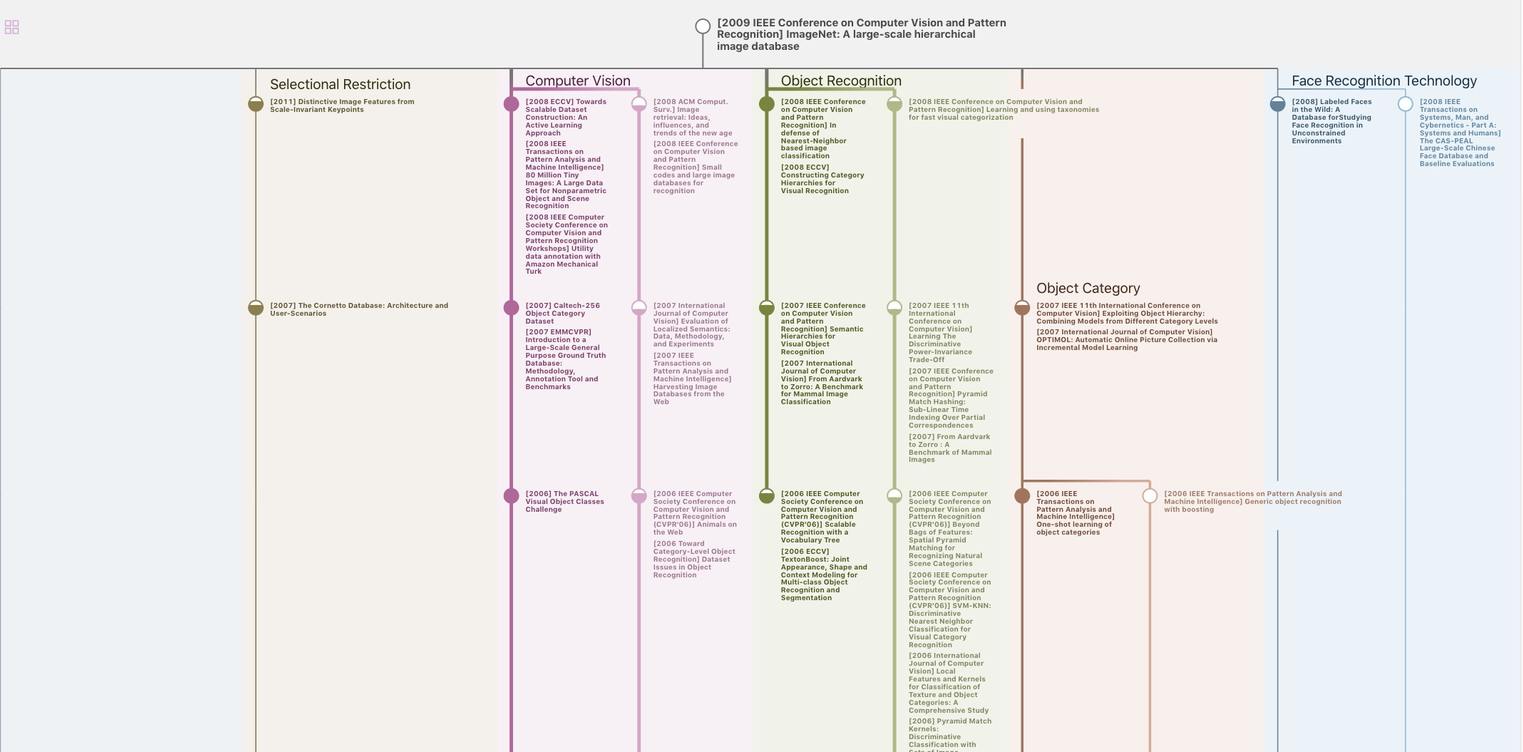
生成溯源树,研究论文发展脉络
Chat Paper
正在生成论文摘要