"Click" Is Not Equal to "Like": Counterfactual Recommendation for Mitigating Clickbait Issue
arxiv(2020)
摘要
The ubiquity of implicit feedback (e.g., click) makes it the default choice to train recommenders. However, such implicit feedback usually has intrinsic noises and bias that interfere the inference of user preference. For instance, many users click micro-videos due to their attractive titles, and then quickly quit because they dislike the content. The inconsistency between clicks and actual user preference induces that learning recommender from clicks will have clickbait bias: items with attractive clickbait content will be frequently recommended. However, mitigating clickbait bias within implicit feedback has received relatively little scrutiny. The reason for clickbait bias is the discrepancy between pre-click features (e.g., title) and post-click ones (e.g., video), i.e., pre-click features attract users' interests while post-click features are dissatisfying. Recommenders will rely more on pre-click features to also fit these clicks well during training, and thus assign high prediction scores to items with clickbait content during inference. As such, the key to mitigating clickbait bias is to decrease the direct effect of pre-click features on predictions. To this end, we propose the counterfactual recommendation (shorted asCFRec) which utilizes counterfactual thinking to model the direct effect of pre-click features. In the counterfactual world, CFRec imagines the items only have pre-click features and estimates the prediction scores purely affected by pre-click features. Thereafter, we exclude the direct effect by subtracting it from the total effect of both pre-click and post-click features, i.e., the prediction score in the factual world. We implement CFRec on MMGCN and test it on two widely used recommendation benchmarks. Extensive experimental results validate the effectiveness of the proposed CFRec.
更多查看译文
AI 理解论文
溯源树
样例
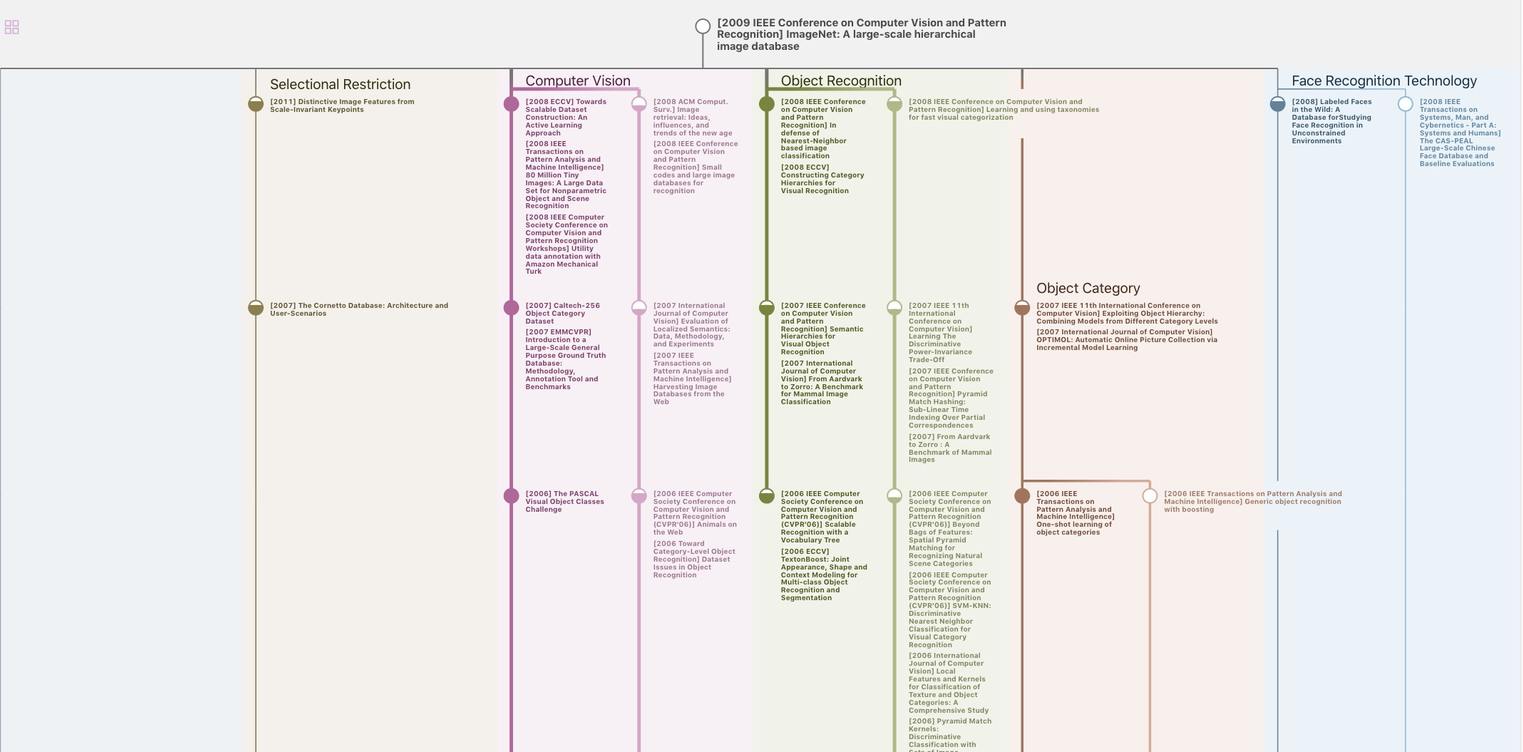
生成溯源树,研究论文发展脉络
Chat Paper
正在生成论文摘要