Investigating Deep Feedforward Neural Networks for Classification of Transposon-Derived Pirnas
Complex & intelligent systems(2021)
摘要
PIWI-interacting RNAs (piRNAS) form an important class of non-coding RNAs that play a key role in gene expression regulation and genome integrity by silencing transposable elements. However, despite the importance of piRNAs and the large application of deep learning in computational biology, there are few studies of deep learning for piRNAs prediction. Still, current methods focus on using advanced architectures like CNN and variations. This paper presents an investigation on deep feedforward network models for classification of human transposon-derived piRNAs. We developed a lightweight predictor (when compared to other deep learning methods) and we show by practical evidence that simple neural networks can perform as well as better than complex neural networks when using the appropriate hyperparameters. For that, we train, analyze and compare the results of a multilayer perceptron with different hyperparameter choices, such as numbers of hidden layers, activation functions and optimizers, clarifying the advantages and disadvantages of each choice. Our proposed predictor reached a F -score of 0.872, outperforming other state-of-the-art methods for human transposon-derived piRNAs classification. In addition, to better access the generalization of our proposal, we also showed it achieved competitive results when classifying piRNAs of other species.
更多查看译文
关键词
piRNAs classification,Neural networks,Deep learning,Hyperparameters optimization
AI 理解论文
溯源树
样例
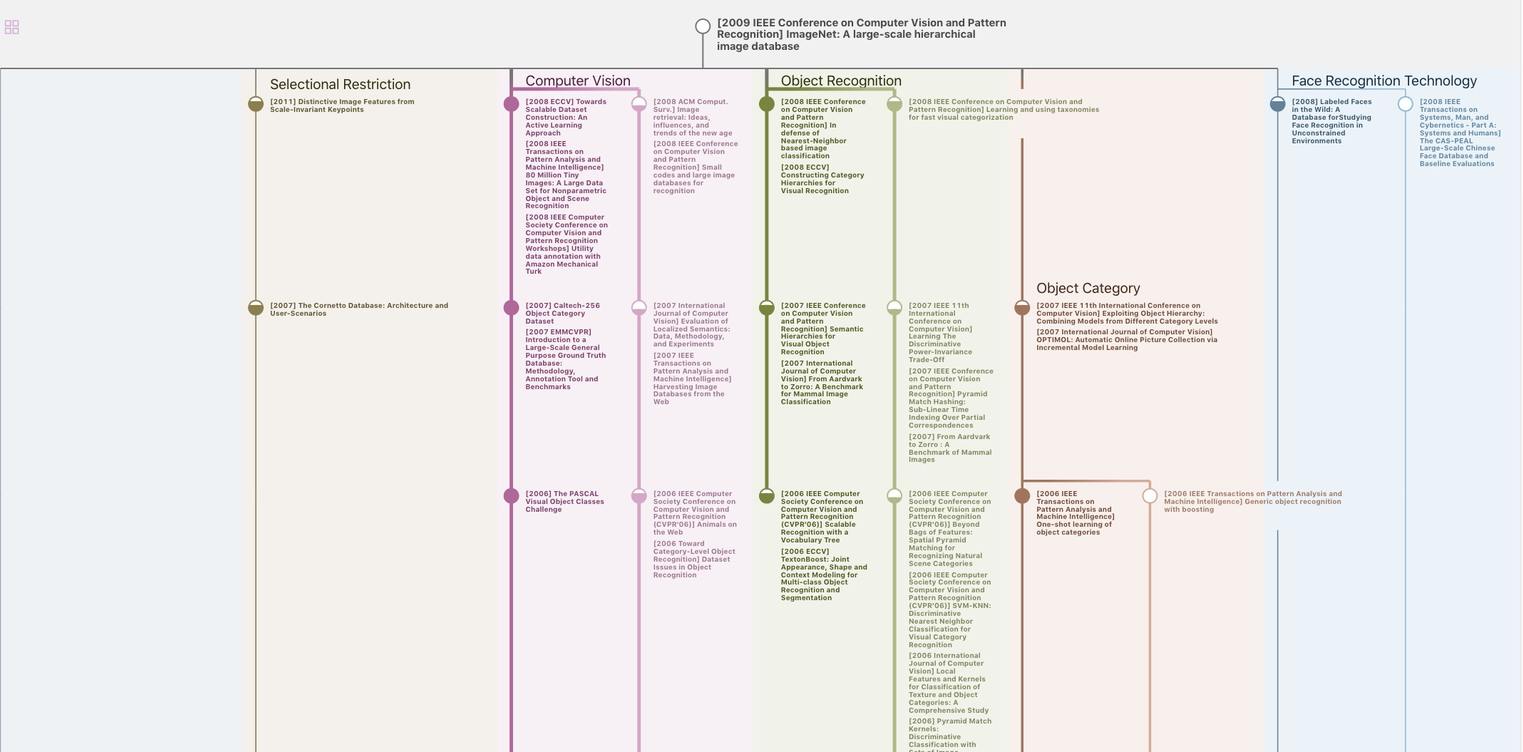
生成溯源树,研究论文发展脉络
Chat Paper
正在生成论文摘要