Transfer learning improves antibiotic resistance class prediction
biorxiv(2020)
摘要
Motivation Antibiotic resistance is a growing public health problem, which affects millions of people worldwide, and if left unchecked is expected to upend many aspects of healthcare as it is practiced today. Identifying the type of antibiotic resistant genes in genome and metagenomic sample is of utmost importance in the prevention, diagnosis, and treatment of infections. Today there are multiple tools available that predict antibiotic resistance class from DNA and protein sequences, yet there is a lack of benchmarks on the performances of these tools.
Results We have developed a dataset that is curated from 15 available databases, and annotated with their antibiotic class labels. We also developed a transfer learning approach with neural networks, TRAC, that outperforms existing antiobiotic resistance prediction tools. While TRAC provides the current state-of-the-art performance, we hope our newly developed dataset will also provide the community with a much needed standardized dataset to develop novel methods that can predict antibiotic resistance class with superior prediction performance.
Availability TRAC is available at github () and the datasets are available at figshare ().
Contact mhamid{at}mgh.harvard.edu, idoerg{at}iastate.edu
### Competing Interest Statement
The authors have declared no competing interest.
更多查看译文
关键词
antibiotic resistance class prediction,learning,transfer
AI 理解论文
溯源树
样例
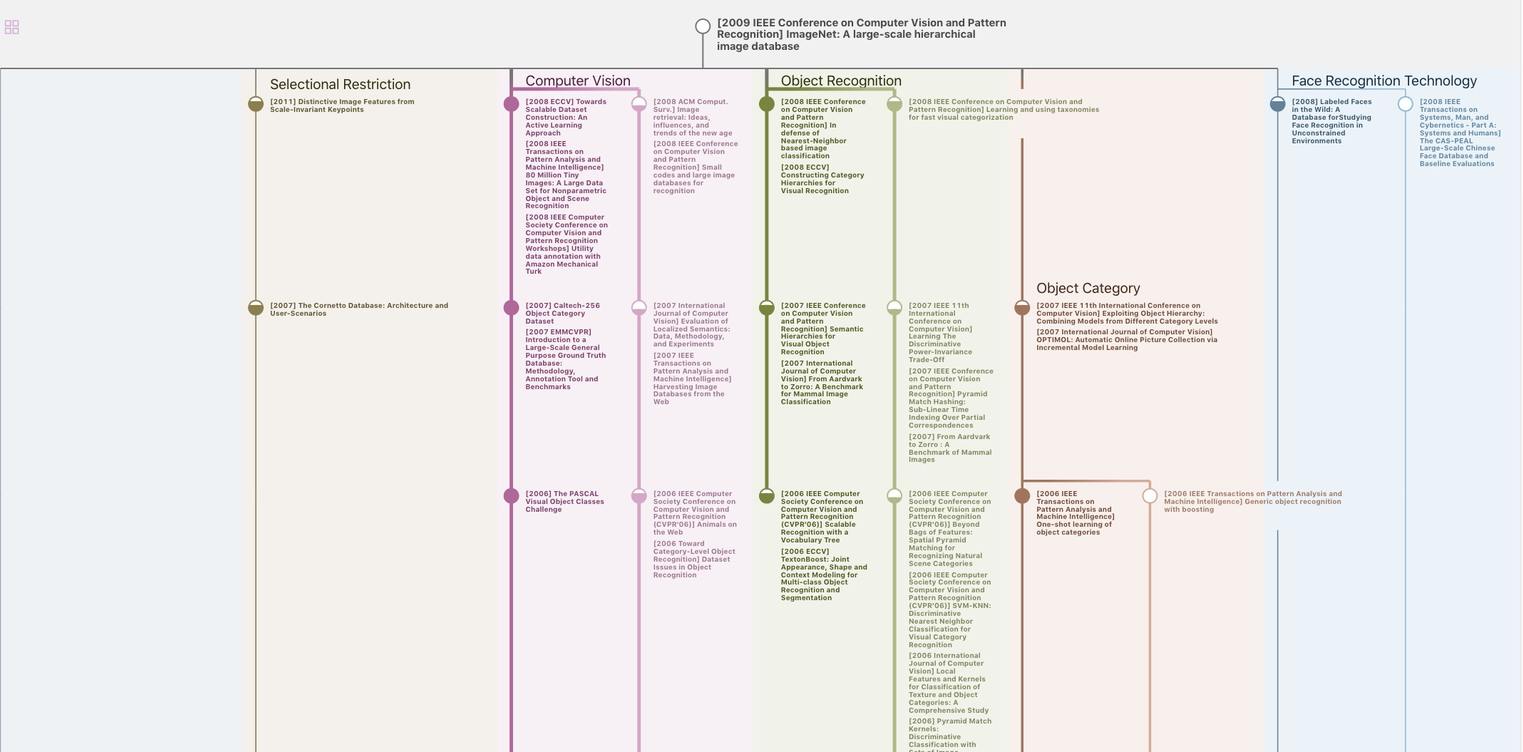
生成溯源树,研究论文发展脉络
Chat Paper
正在生成论文摘要