Deep Learning Improves Macromolecules Localization and Identification in 3D Cellular Cryo-Electron Tomograms
biorxiv(2020)
摘要
Cryo-electron tomography (cryo-ET) allows one to visualize and study the 3D spatial distribution of macromolecules, in their native states and at nanometer resolution in single cells. While this label-free cryogenic imaging technology produces data containing rich structural information, automatic localization and identification of macromolecules are prone to noise and reconstruction artifacts, and to the presence of many molecular species in small areas. Hence, we present a computational procedure that uses artificial neural networks to accurately localize several macromolecular species in cellular cryo-electron tomograms. The DeepFinder algorithm leverages deep learning and outperforms the commonly-used template matching method on synthetic datasets. Meanwhile, DeepFinder is very fast when compared to template matching, and is better capable of localizing and identifying small macro-molecules than other competitive deep learning methods. On experimental cryo-ET data depicting ribosomes, the localization and structure resolution (determined through subtomogram averaging) results obtained with DeepFinder are consistent with those obtained by experts. The DeepFinder algorithm is able to imitate the analysis performed by experts, and is therefore a very promising algorithm to investigate efficiently the contents of cellular tomograms. Furthermore, we show that DeepFinder can be combined with a template matching procedure to localize the missing macromolecules not found by one or the other method. Application of this collaborative strategy allowed us to find additional 20.5% membrane-bound ribosomes that had been missed or discarded during manual template matching-assisted annotation.
更多查看译文
AI 理解论文
溯源树
样例
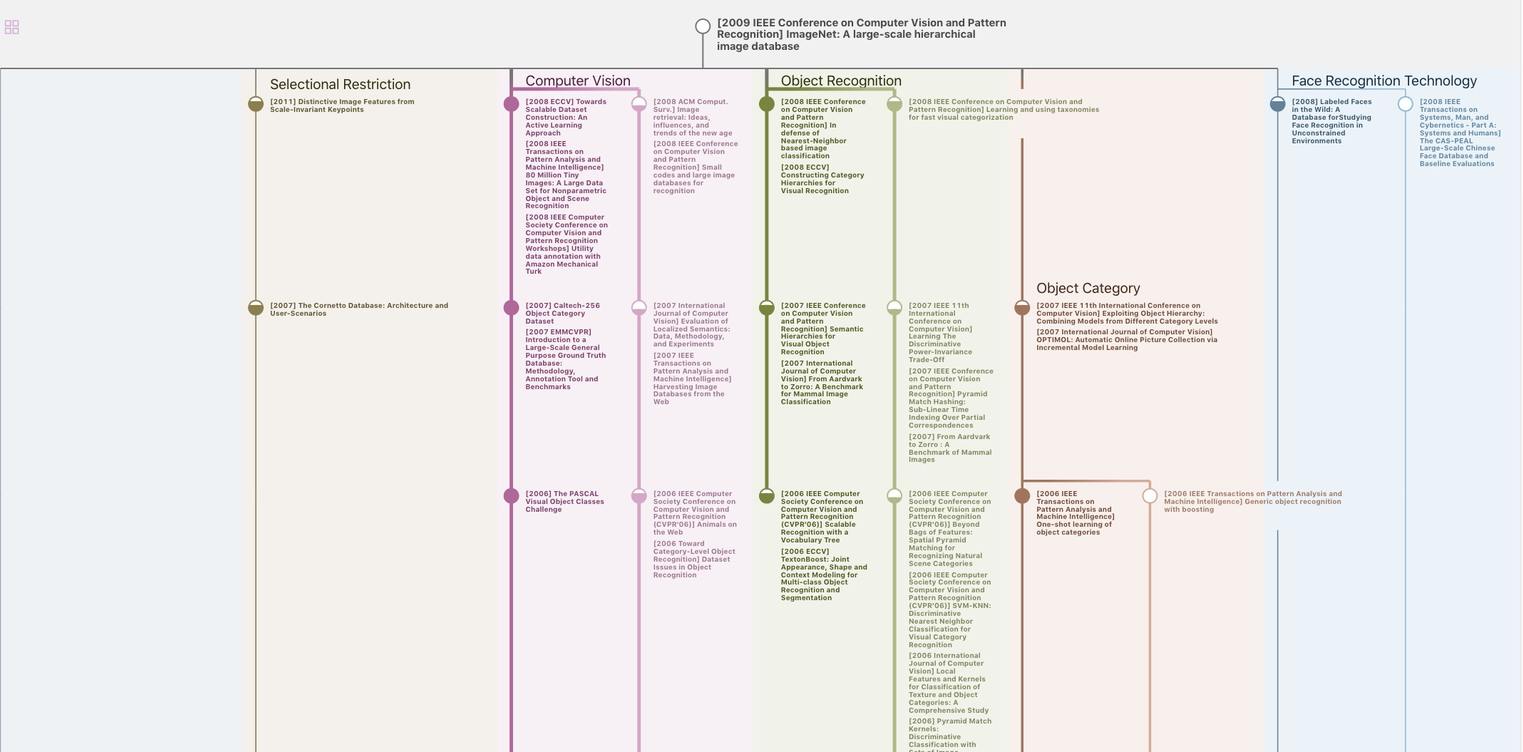
生成溯源树,研究论文发展脉络
Chat Paper
正在生成论文摘要