Optimal provable robustness of quantum classification via quantum hypothesis testing
NPJ QUANTUM INFORMATION(2021)
摘要
Quantum machine learning models have the potential to offer speedups and better predictive accuracy compared to their classical counterparts. However, these quantum algorithms, like their classical counterparts, have been shown to also be vulnerable to input perturbations, in particular for classification problems. These can arise either from noisy implementations or, as a worst-case type of noise, adversarial attacks. In order to develop defense mechanisms and to better understand the reliability of these algorithms, it is crucial to understand their robustness properties in the presence of natural noise sources or adversarial manipulation. From the observation that measurements involved in quantum classification algorithms are naturally probabilistic, we uncover and formalize a fundamental link between binary quantum hypothesis testing and provably robust quantum classification. This link leads to a tight robustness condition that puts constraints on the amount of noise a classifier can tolerate, independent of whether the noise source is natural or adversarial. Based on this result, we develop practical protocols to optimally certify robustness. Finally, since this is a robustness condition against worst-case types of noise, our result naturally extends to scenarios where the noise source is known. Thus, we also provide a framework to study the reliability of quantum classification protocols beyond the adversarial, worst-case noise scenarios.
更多查看译文
关键词
Computer science,Quantum information,Physics,general,Quantum Physics,Quantum Information Technology,Spintronics,Quantum Computing,Quantum Field Theories,String Theory,Classical and Quantum Gravitation,Relativity Theory
AI 理解论文
溯源树
样例
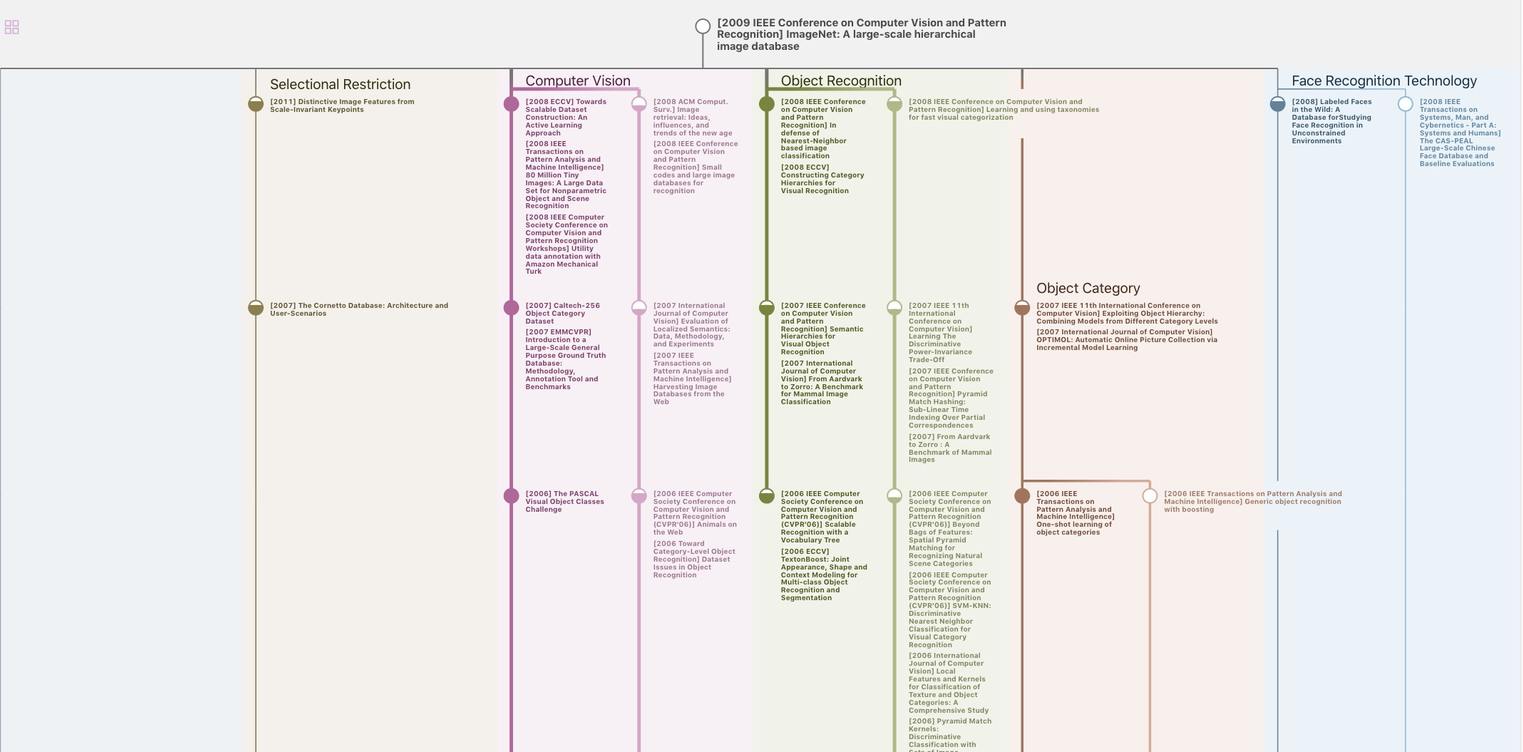
生成溯源树,研究论文发展脉络
Chat Paper
正在生成论文摘要