Attribution of genetic engineering: A practical and accurate machine-learning toolkit for biosecurity
biorxiv(2020)
摘要
The promise of biotechnology is tempered by its potential for accidental or deliberate misuse. Reliably identifying telltale signatures characteristic to different genetic designers, termed genetic engineering attribution , would deter misuse, yet is still considered unsolved. Here, we show that recurrent neural networks trained on DNA motifs and basic phenotype can reach 70% attribution accuracy distinguishing between over 1,300 labs. To make these models usable in practice, we introduce a framework for weighing predictions against other investigative evidence using calibration, and bring our model to within 1.6% of perfect calibration. Additionally, we demonstrate that simple models can accurately predict both the nation-state-of-origin and ancestor labs, forming the foundation of an integrated attribution toolkit which should promote responsible innovation and international security alike.
### Competing Interest Statement
E.C.A. is President and J.S. is Co-Founder of Alt. Technology Labs (altLabs), a not-for-profit organization hosting an open data science attribution prize. E.C.A. and K.M.E are board members of altLabs, and G.M.C. is a member of the altLabs Scientific Advisory Board. A full list of G.M.C.'s tech transfer, advisory roles, and funding sources can be found on the lab's website http://arep.med.harvard.edu/gmc/tech.html.
更多查看译文
关键词
genetic engineering,attribution,machine-learning machine-learning
AI 理解论文
溯源树
样例
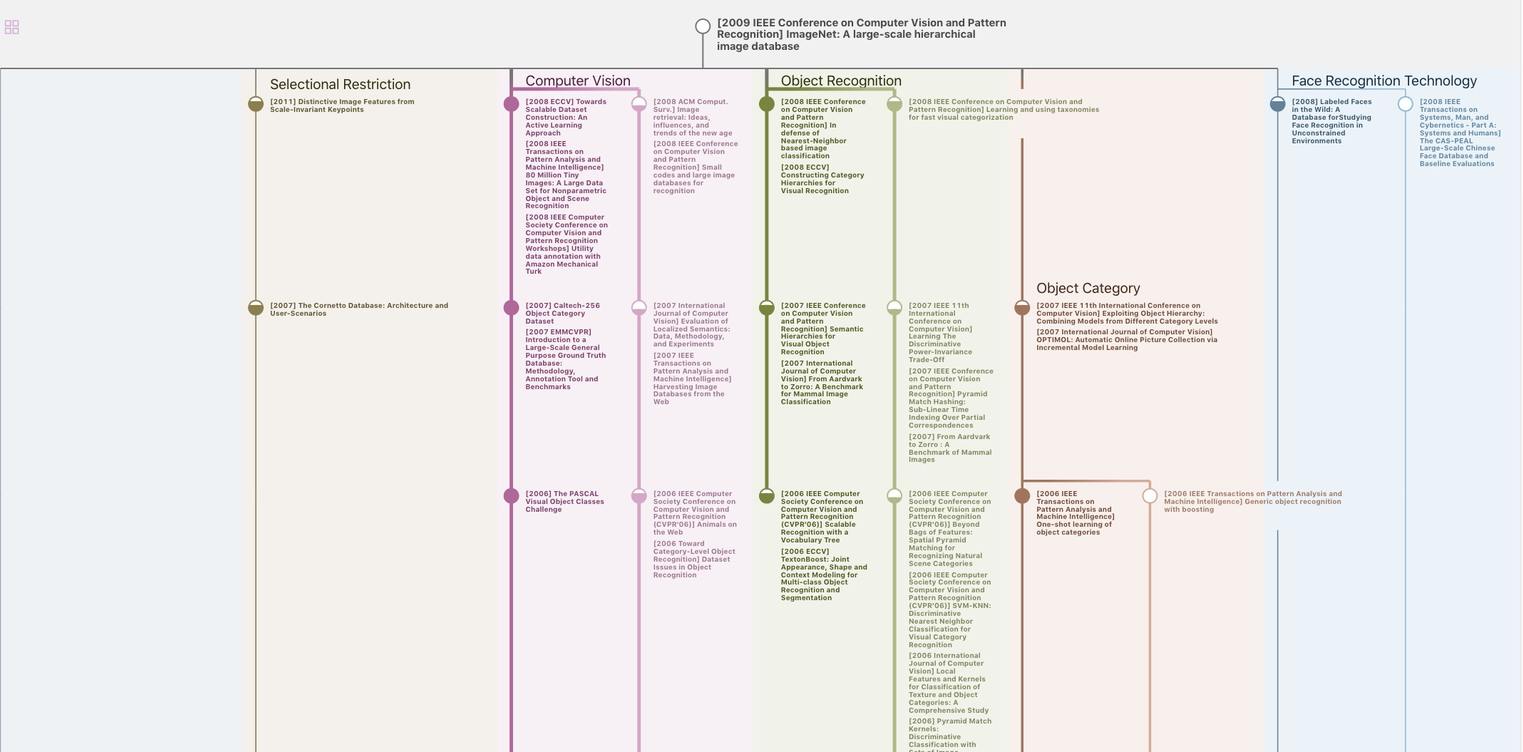
生成溯源树,研究论文发展脉络
Chat Paper
正在生成论文摘要