A deep learning approach to identify new gene targets of a novel therapeutic for human splicing disorders
biorxiv(2020)
摘要
Pre-mRNA splicing is a key control point in human gene expression. Disturbances in splicing due to mutation or aberrant splicing regulatory networks lead to dysregulated protein expression and contribute to a substantial fraction of human disease. Several classes of active and selective splicing modulator compounds have been recently identified, thus proving that pre-mRNA splicing is a viable target for therapy. We describe herein the identification of BPN-15477, a novel splicing modulator compound, that restores correct splicing of exon 20 in the Elongator complex protein 1 gene carrying the major IVS20+6T>C mutation responsible for familial dysautonomia. We then developed a machine learning approach to evaluate the therapeutic potential of BPN-15477 to correct splicing in other human genetic diseases. Using transcriptome sequencing from compound-treated fibroblast cells, we identified treatment responsive sequence signatures, the majority of which center at the 5’ splice site of exons whose inclusion or exclusion is modulated by SMC treatment. We then leveraged this model to identify 155 human disease genes that harbor ClinVar mutations predicted to alter pre-mRNA splicing as potential targets for BPN-15477 treatment. Using splicing assays, we validated representative predictions by demonstrating successful correction of splicing defects caused by mutations in genes responsible for cystic fibrosis (), cholesterol ester storage disease (), Lynch syndrome () and familial frontotemporal dementia (). Our study shows that deep learning techniques can identify a complex set of sequence signatures and predict response to pharmacological modulation, strongly supporting the use of approaches to expand the therapeutic potential of drugs that modulate splicing.
更多查看译文
AI 理解论文
溯源树
样例
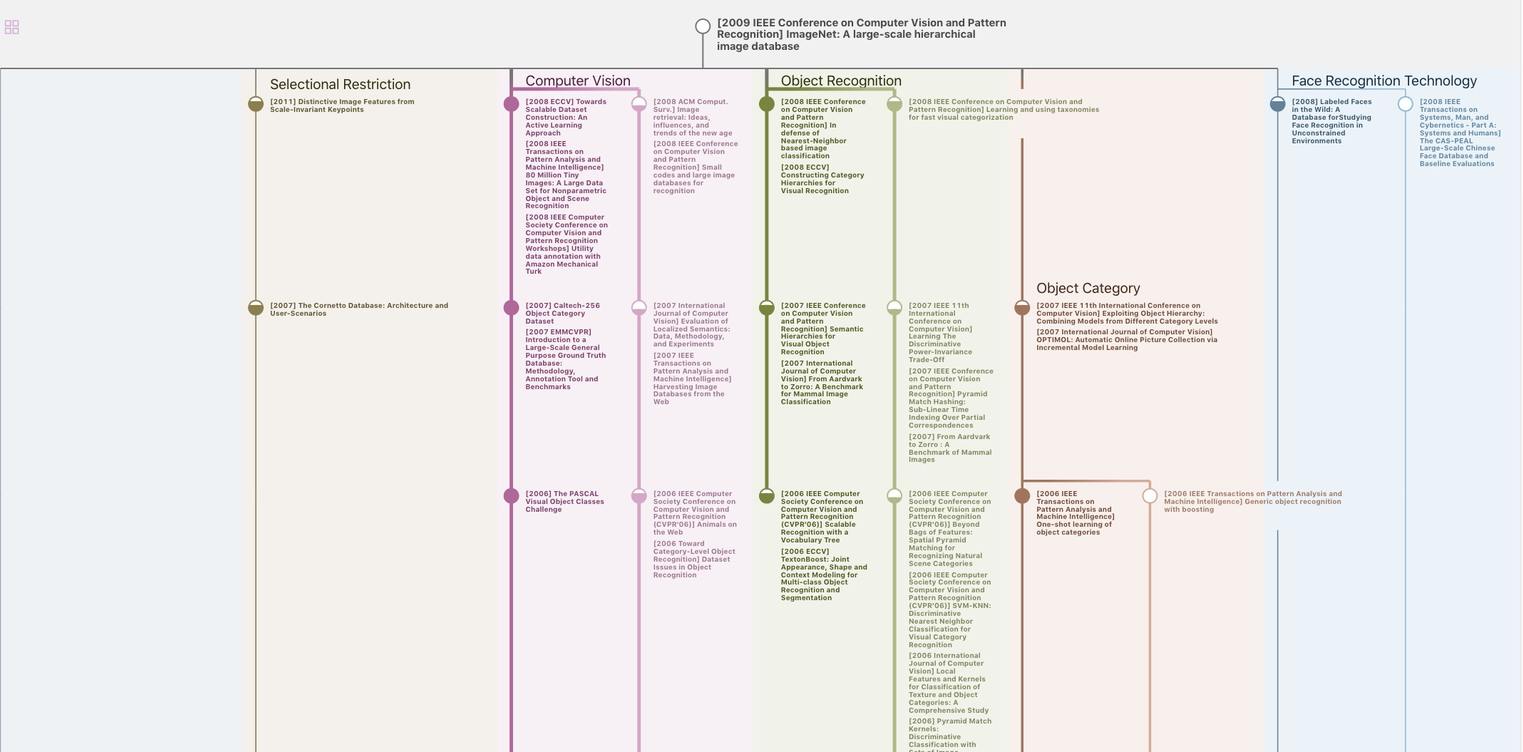
生成溯源树,研究论文发展脉络
Chat Paper
正在生成论文摘要