Towards Autonomous Intra-cortical Brain Machine Interfaces: Applying Bandit Algorithms for Online Reinforcement Learning
ISCAS(2020)
摘要
This paper presents application of Banditron - an online reinforcement learning algorithm (RL) in a discrete state intra-cortical Brain Machine Interface (iBMI) setting. We have analyzed two datasets from non-human primates (NHPs) - NHP A and NHP B each performing a 4-option discrete control task over a total of 8 days. Results show average improvements of ≈ 15%, 6% in NHP A and 15%, 21% in NHP B over state of the art algorithms - Hebbian Reinforcement Learning (HRL) and Attention Gated Reinforcement Learning (AGREL) respectively. Apart from yielding a superior decoding performance, Banditron is also the most computationally friendly as it requires two orders of magnitude less multiply-and-accumulate operations than HRL and AGREL. Furthermore, Banditron provides average improvements of at least 40%, 15% in NHPs A, B respectively compared to popularly employed supervised methods - LDA, SVM across test days. These results pave the way towards an alternate paradigm of temporally robust hardware friendly reinforcement learning based iBMIs.
更多查看译文
关键词
iBMIs,robust hardware friendly reinforcement learning,Hebbian reinforcement learning,autonomous intra-cortical brain machine interfaces,discrete state intra-cortical brain machine interface,gated reinforcement learning,decoding performance,AGREL,HRL,4-option discrete control task,NHP B,NHP A,nonhuman primates,online reinforcement learning,Banditron,bandit algorithms,time 8.0 d
AI 理解论文
溯源树
样例
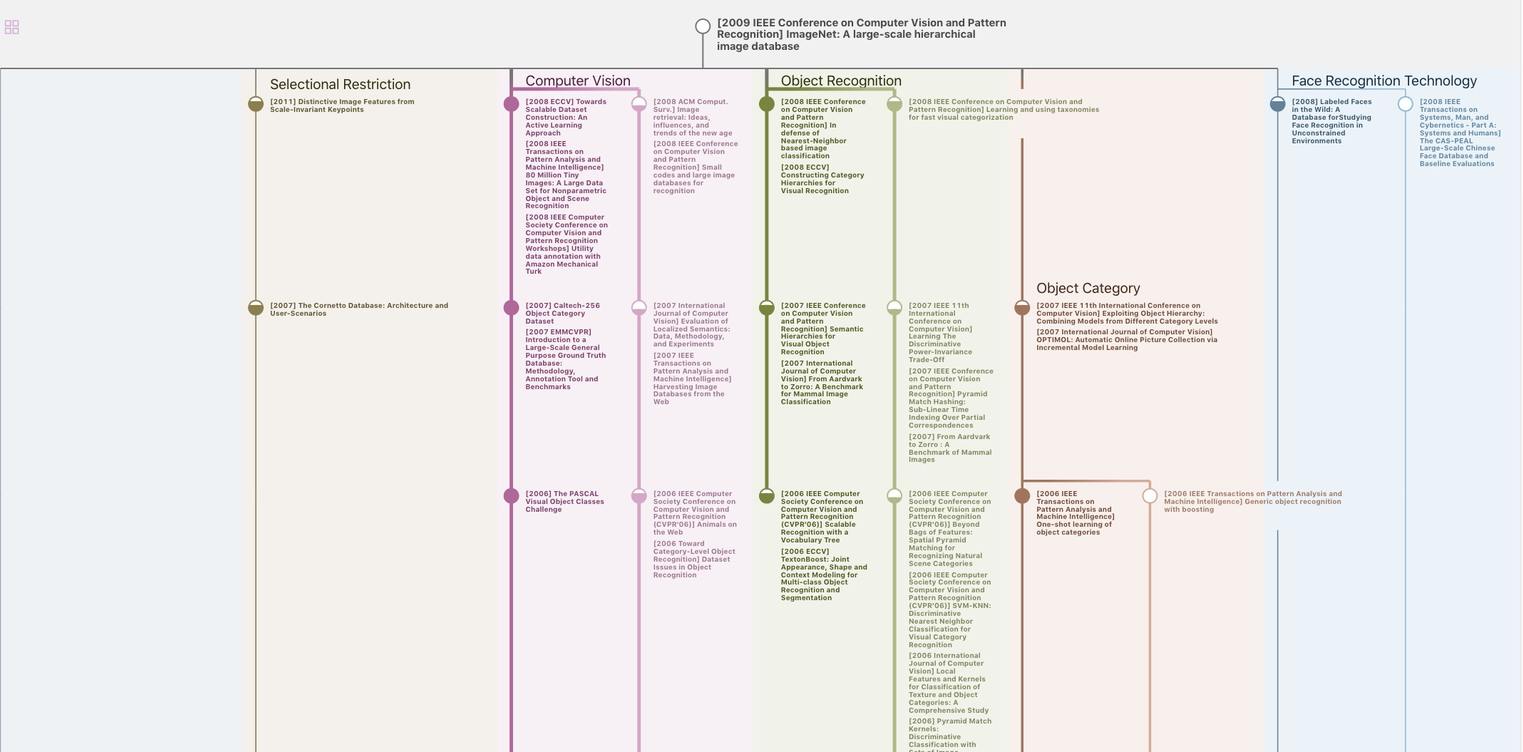
生成溯源树,研究论文发展脉络
Chat Paper
正在生成论文摘要