Explainable AI reveals key changes in skin microbiome associated with menopause, smoking, aging and skin hydration
biorxiv(2020)
摘要
Background: Alterations in the human microbiome have been observed in a variety of conditions such has asthma, depression, gingivitis, dermatitis and cancer, and much remains to be learned about the precise mechanistic links between the microbiome and human health. The fusion of artificial intelligence with rich microbiome datasets has the potential to shed light on the complex interactions between microbes and their host and to offer an improved understanding of the microbiome9s role in our health and wellbeing. To gain actionable insights it is essential to consider both the predictive power and the transparency of the models by providing an explanation for the predictions. We combine the effort of collecting a large corpus of leg skin microbiome samples and amplicon sequencing data of two healthy cohorts of Canadian and UK women with the development of an explainable artificial intelligence approach that provides both accurate predictions of phenotypes and explanations for them. Results: We accurately predict pre/post-menopausal status (F1-score of ~93% on the test set), smoking status (an F1-score of ~95% on the test set), subject9s age (an average error within 4 years of chronological age), and skin hydration from the skin microbiome. The explanations for these predictions link microbial composition signatures to phenotypes by considering changes in the relative abundance of microbes that impact the prediction of diverging phenotypes. The signatures from the leg microbiome associated with smoking are consistent with previous findings from gut and oral microbiomes, indicating that the microbiomes across the entire body are affected by smoking. Similarly, the leg microbiome signatures reflect key aspects of the vaginal microbiome allowing us to use it to predict pre/post-menopausal status. This suggests that easily accessible microbiome samples could be used to investigate health-related phenotypes, offering potential for non-invasive diagnosis and condition monitoring. The identified key changes in microbial composition linked to skin hydration can accelerate the development of suitable personalised treatments for healthy skin, while those associated with age may offer insights into the aging process of skin. Conclusion: The explainability of our phenotype prediction approach sets the stage for new work focused on understanding the complex relationships between microbial communities and phenotypes. Our approach can be extended to predict any conditions from microbiome samples and to accelerate the development of microbiome-based personalised therapeutics and non-invasive diagnostics.
更多查看译文
关键词
skin microbiome,microbial signatures,explainable artificial intelligence
AI 理解论文
溯源树
样例
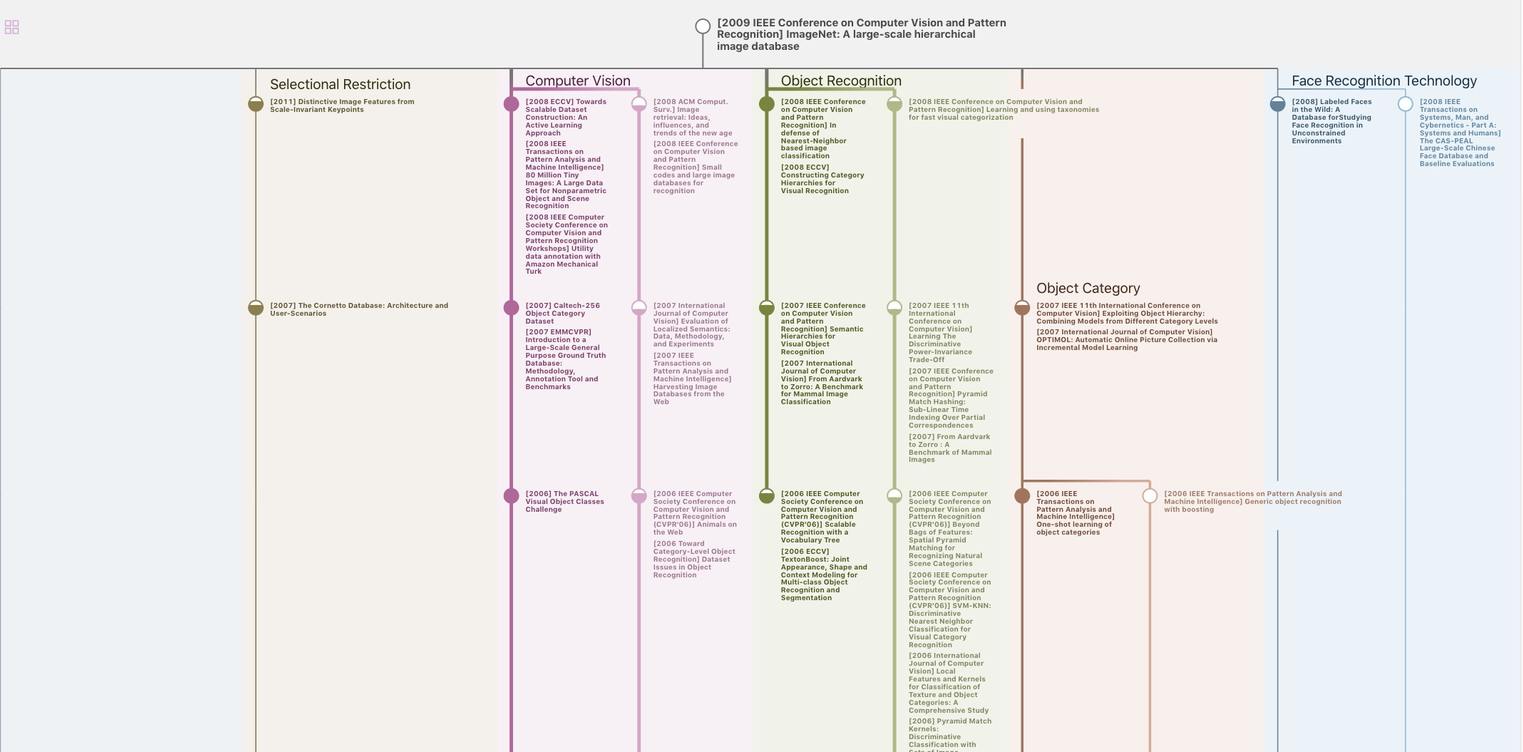
生成溯源树,研究论文发展脉络
Chat Paper
正在生成论文摘要