De novo protein design by deep network hallucination
biorxiv(2021)
摘要
There has been considerable recent progress in protein structure prediction using deep neural networks to predict inter-residue distances from amino acid sequences 1 – 3 . Here we investigate whether the information captured by such networks is sufficiently rich to generate new folded proteins with sequences unrelated to those of the naturally occurring proteins used in training the models. We generate random amino acid sequences, and input them into the trRosetta structure prediction network to predict starting residue–residue distance maps, which, as expected, are quite featureless. We then carry out Monte Carlo sampling in amino acid sequence space, optimizing the contrast (Kullback–Leibler divergence) between the inter-residue distance distributions predicted by the network and background distributions averaged over all proteins. Optimization from different random starting points resulted in novel proteins spanning a wide range of sequences and predicted structures. We obtained synthetic genes encoding 129 of the network-‘hallucinated’ sequences, and expressed and purified the proteins in Escherichia coli ; 27 of the proteins yielded monodisperse species with circular dichroism spectra consistent with the hallucinated structures. We determined the three-dimensional structures of three of the hallucinated proteins, two by X-ray crystallography and one by NMR, and these closely matched the hallucinated models. Thus, deep networks trained to predict native protein structures from their sequences can be inverted to design new proteins, and such networks and methods should contribute alongside traditional physics-based models to the de novo design of proteins with new functions.
更多查看译文
关键词
deep network hallucination,protein
AI 理解论文
溯源树
样例
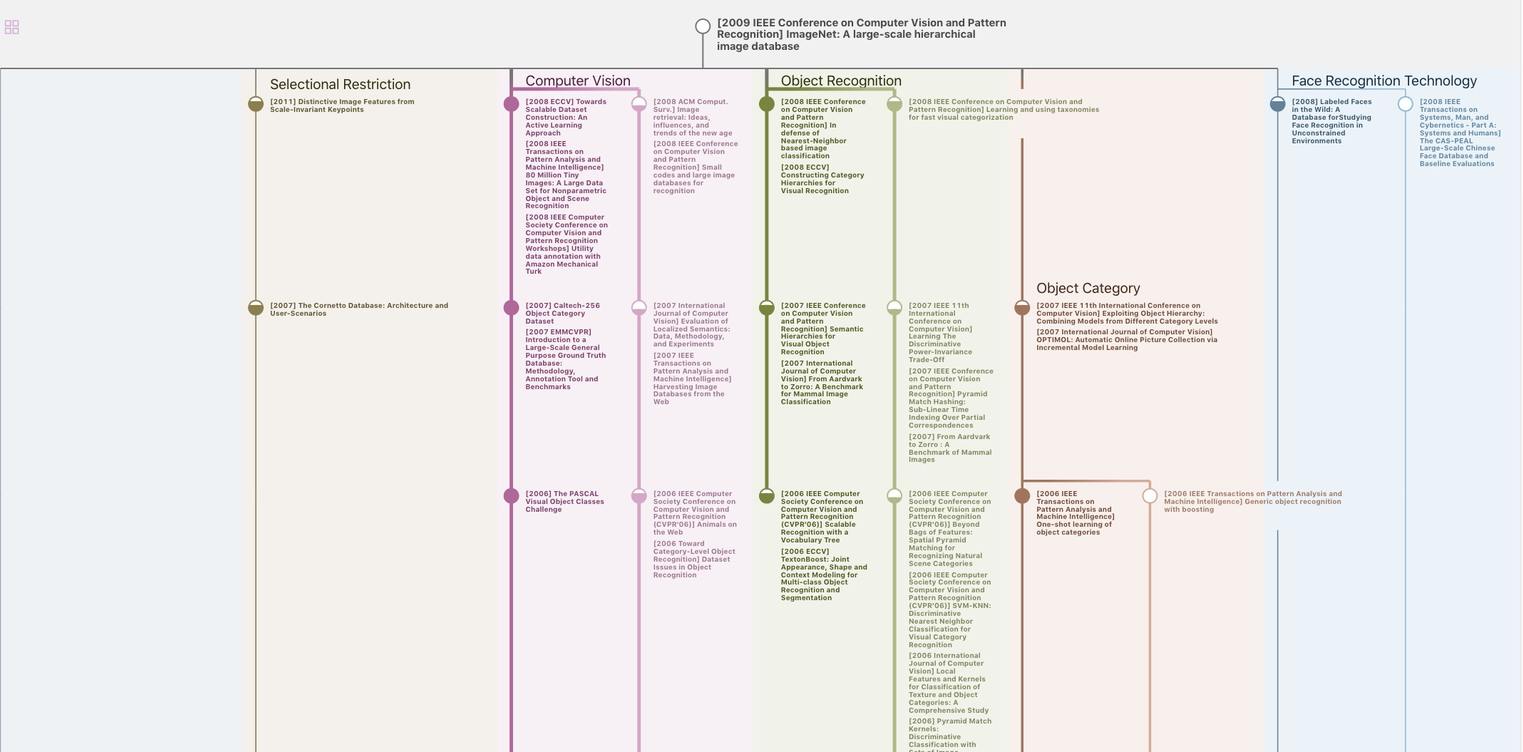
生成溯源树,研究论文发展脉络
Chat Paper
正在生成论文摘要