Optimizing the use of gene expression data to predict metabolic pathway memberships with unsupervised and supervised machine learning
biorxiv(2020)
摘要
Plants produce diverse metabolites via enzymes metabolic pathways important for plant survival, human nutrition and medicine. However, most plant enzyme genes are of unknown pathway membership. While some genes in the same pathways can be identified based on correlated expression, such correlation may exist only under specific spatiotemporal and conditional contexts. By considering 656 combinations of tomato gene expression datasets calculated with eight co-expression measures, we evaluated the performance of naive prediction (based on expression similarities to pathways), unsupervised and supervised learning methods in predicting memberships in 85 metabolic pathways. We found that optimal predictions for different pathways require different dataset, which tend to be associated with the biological processes related to the pathway functions. In addition, naive prediction has significantly lower performance compared to machine learning methods. Interestingly, the unsupervised learning approach has better performance in 52 pathways than the supervised approach, which may be attributed to the need for more data with supervised learning. Furthermore, machine learning clustering/models using gene-to-pathway expression similarities outperform that with gene expression profiles. Altogether, our study highlights the need to extensively explore expression-based features to maximize the utility of expression data for pinpointing pathway membership. Through this detailed exploration, novel connections between pathways and biological processes can also be identified based on the optimal expression dataset used, improving our mechanistic understanding of the metabolic network.
更多查看译文
关键词
plant metabolic pathway memberships,gene expression data,gene expression
AI 理解论文
溯源树
样例
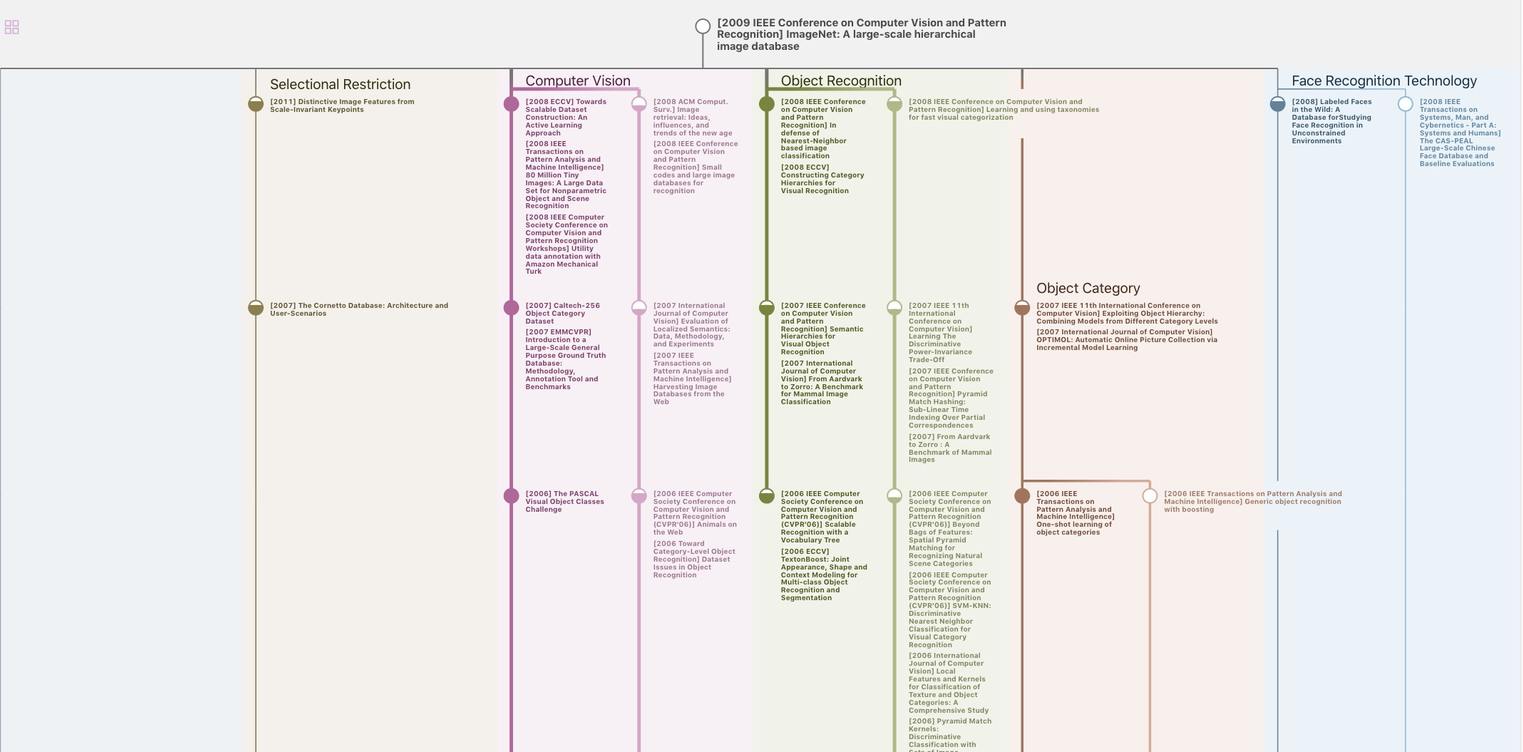
生成溯源树,研究论文发展脉络
Chat Paper
正在生成论文摘要