PRECISE+ predicts drug response in patients by non-linear subspace-based transfer from cell lines and PDX models
biorxiv(2020)
摘要
Pre-clinical models have been the workhorse of cancer research for decades. Albeit powerful, these models do not perfectly recapitulate the complexity of human tumors which has led to a disappointing bench-to-bedside attrition rate. The quest for biomarkers of drug response signatures has been particularly challenging, suffering from poor translatability from pre-clinical models to human tumors. To address this problem, we present a novel computational framework, PRECISE+, that employs nonlinear kernel approaches to capture complex biological processes expressed in both pre-clinical models and human tumors. PRECISE+ builds predictors on cell lines that show improved performance over competing approaches for a set of 7 of drugs in Patient-Derived Xenografts, 5 drugs on TCGA cohorts and show significant association with clinical response for 4 drugs in 226 metastatic tumors from the Hartwig Medical Foundation data set. We used the interpretability of PRECISE+ to validate the approach by identifying known biomarkers to targeted therapies and propose novel putative biomarkers of resistance to Paclitaxel and Gemcitabine.
更多查看译文
AI 理解论文
溯源树
样例
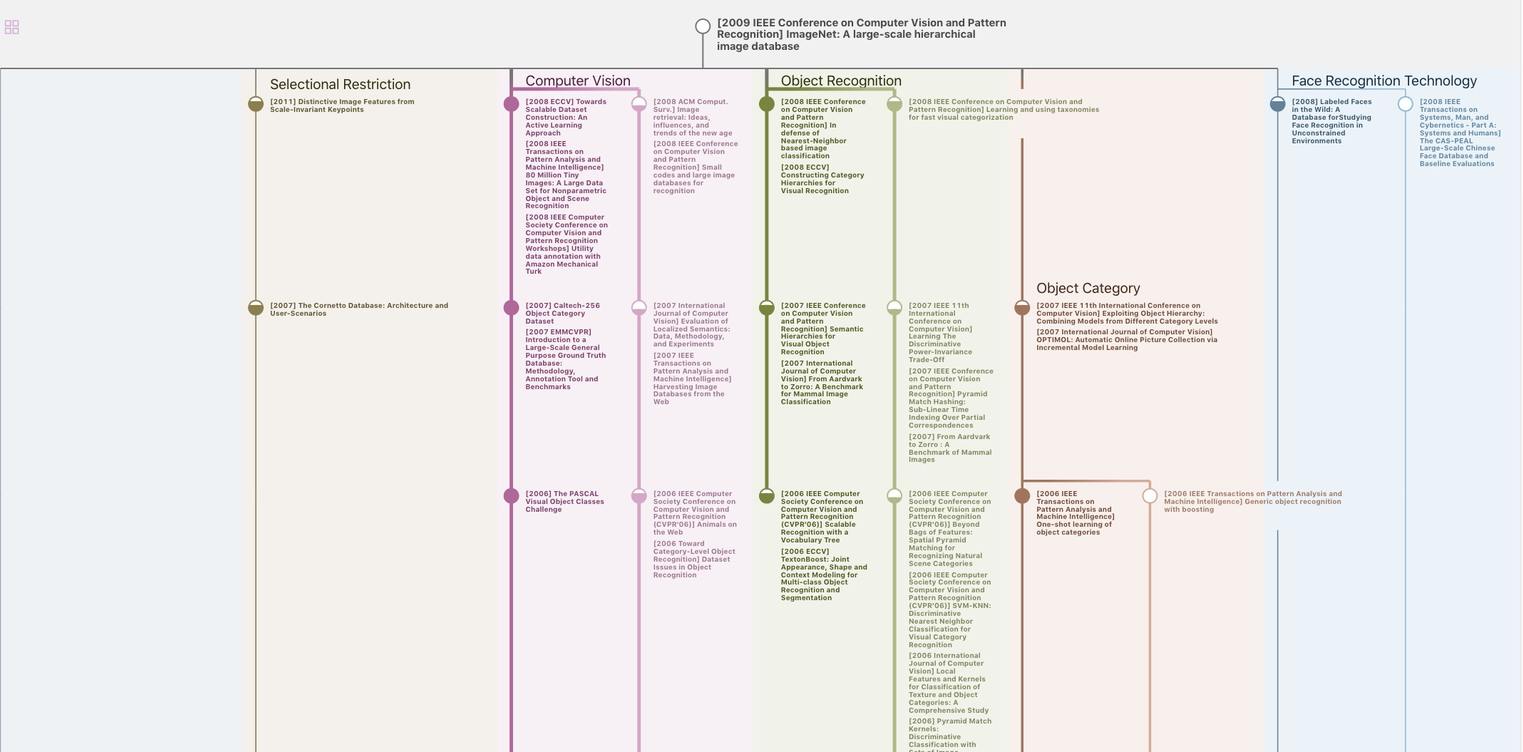
生成溯源树,研究论文发展脉络
Chat Paper
正在生成论文摘要