Learning Peptide Recognition Rules for a Low-Specificity Protein
Protein science(2020)
摘要
Many proteins interact with short linear regions of target proteins. For some proteins, however, it is difficult to identify a well-defined sequence motif that defines its target peptides. To overcome this difficulty, we used supervised machine learning to train a model that treats each peptide as a collection of easily-calculated biochemical features rather than as an amino acid sequence. As a test case, we dissected the peptide-recognition rules for human S100A5 (hA5), a low-specificity calcium binding protein. We trained a Random Forest model against a recently released, high-throughput phage display dataset collected for hA5. The model identifies hydrophobicity and shape complementarity, rather than polar contacts, as the primary determinants of peptide binding specificity in hA5. We tested this hypothesis by solving a crystal structure of hA5 and through computational docking studies of diverse peptides onto hA5. These structural studies revealed that peptides exhibit multiple binding modes at the hA5 peptide interface—all of which have few polar contacts with hA5. Finally, we used our trained model to predict new, plausible binding targets in the human proteome. This revealed a fragment of the protein α-1-syntrophin binds to hA5. Our work helps better understand the biochemistry and biology of hA5, as well as demonstrating how high-throughput experiments coupled with machine learning of biochemical features can reveal the determinants of binding specificity in low-specificity proteins.
更多查看译文
关键词
binding specificity,hydrophobicity,machine learning,peptides,S100 proteins,X-ray crystallography
AI 理解论文
溯源树
样例
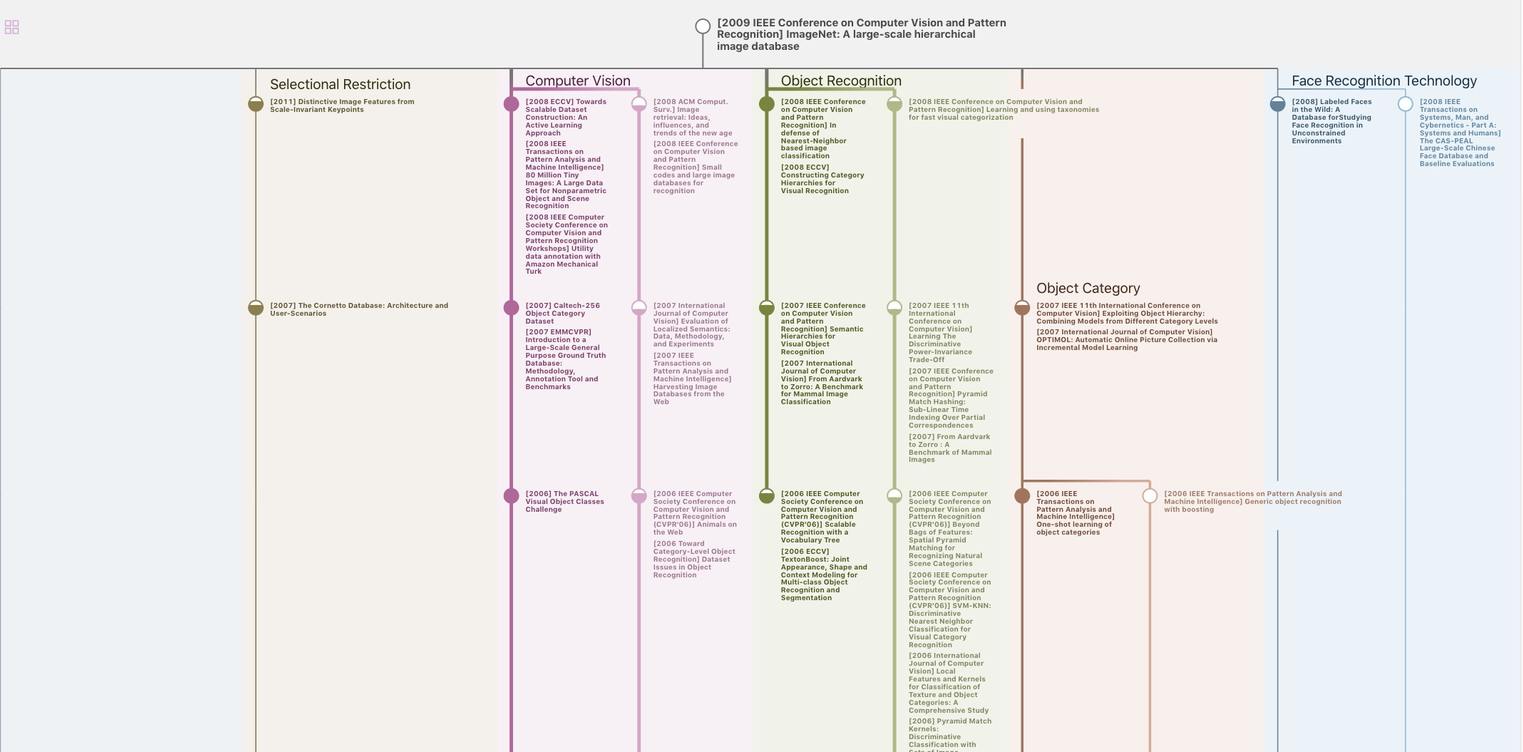
生成溯源树,研究论文发展脉络
Chat Paper
正在生成论文摘要