RNA-seq analyses: Benchmarking differential expression analyses tools reveals the effect of higher number of replicates on performance
biorxiv(2020)
摘要
The introduction of several differential gene expression analysis tools has made it difficult for researchers to settle on a particular tool for RNA-seq analysis. This coupled with the appropriate determination of biological replicates to give an optimum representation of the study population and make biological sense. To address these challenges, we performed a survey of 8 tools used for differential expression in RNA-seq analysis. We simulated 39 different datasets (from 10 to 200 replicates, at an interval of 5) using compcodeR with a maximum of 100 replicates. Our goal was to determine the effect of varying the number of replicates on the performance (F1-score, recall and precision) of the tools. EBSeq and edgeR-glmRT recorded the highest (0.9385) and lowest (0.6505) average F1-score across all replicates, respectively. We also performed a pairwise comparison of all the tools to determine their concordance with each other in identifying differentially expressed genes. We found the greatest concordance to be between and . Finally, we recommend employing edgeR-glmRT for RNA-seq experiments involving 10-50 replicates and edgeR-glmQLF for studies with 55 to 200 replicates.
更多查看译文
关键词
differential expression analyses tools,replicates,differential expression,rna-seq
AI 理解论文
溯源树
样例
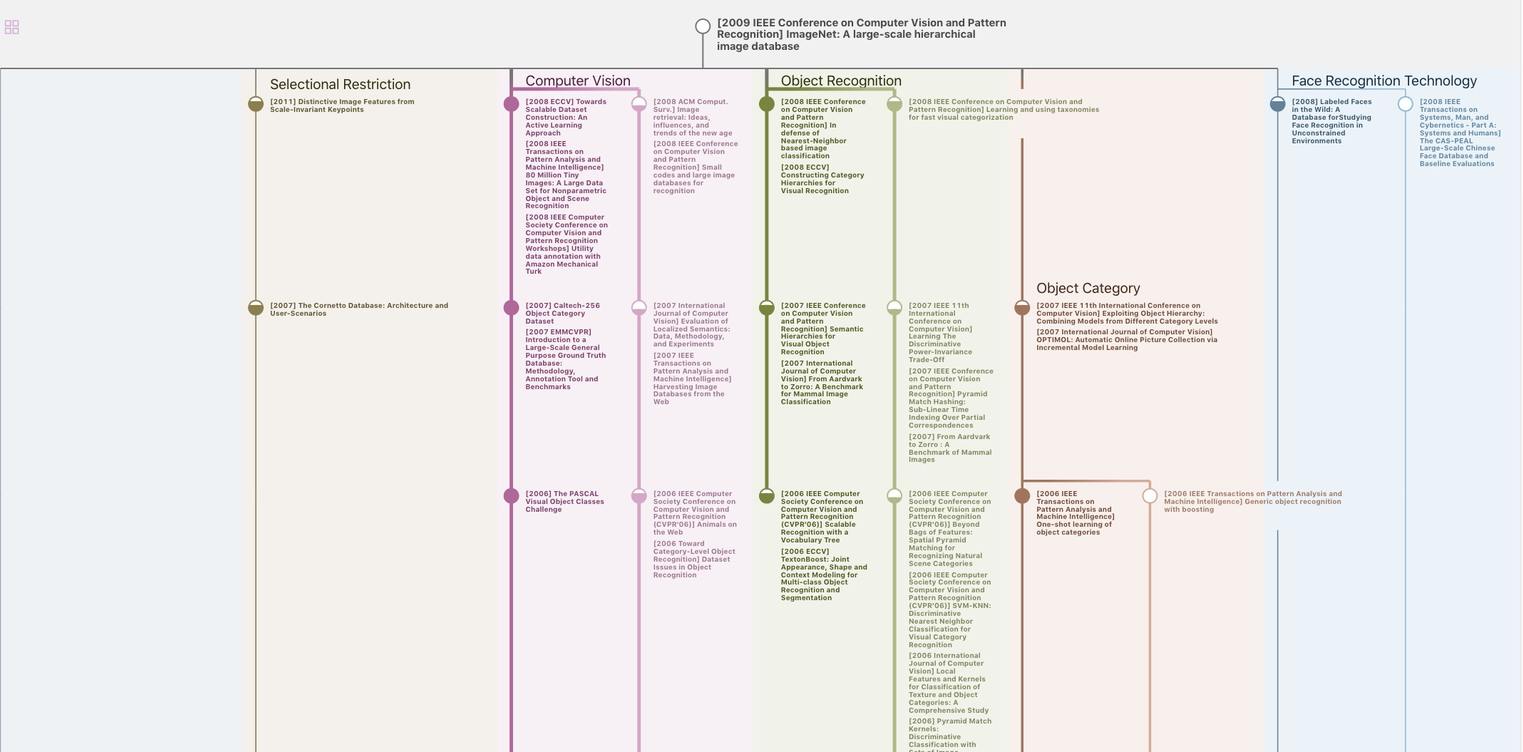
生成溯源树,研究论文发展脉络
Chat Paper
正在生成论文摘要