UNSUPERVISED NEURAL TRACING IN DENSELY LABELED MULTISPECTRAL BRAINBOW IMAGES
2021 IEEE 18TH INTERNATIONAL SYMPOSIUM ON BIOMEDICAL IMAGING (ISBI)(2021)
摘要
Recent advances in imaging technologies for generating large quantities of high-resolution 3D images, especially multispectral labeling technology such as Brainbow, permits unambiguous differentiation of neighboring neurons in a densely labeled brain. This enables, for the first time, the possibility of studying the connectivity between many neurons from a light microscopy image. The lack of reliable automated neuron morphology reconstruction, however, makes data analysis the bottleneck of extracting rich informatics in neuroscience. Supervoxel-based neuron segmentation methods have been proposed to solve this problem, however, previous approaches have been impeded by the large numbers of errors which arise in the final segmentation. In this paper, we present a novel unsupervised approach to trace neurons from multispectral Brainbow images, which prevents segmentation errors and tracing continuity errors using two innovations: First, we formulate a Gaussian mixture model-based clustering strategy to improve the separation of segmented color channels that provides accurate skeletons for the next steps. Then, a skeleton graph approach is proposed to allow the identification and correction of discontinuities in the neuron tree topology. We find that these innovations allow better performance over current state-of-the-art approaches, which results in more accurate neuron tracing results close to human expert annotation.
更多查看译文
关键词
neuron tracing, neuron segmentation, Brainbow images
AI 理解论文
溯源树
样例
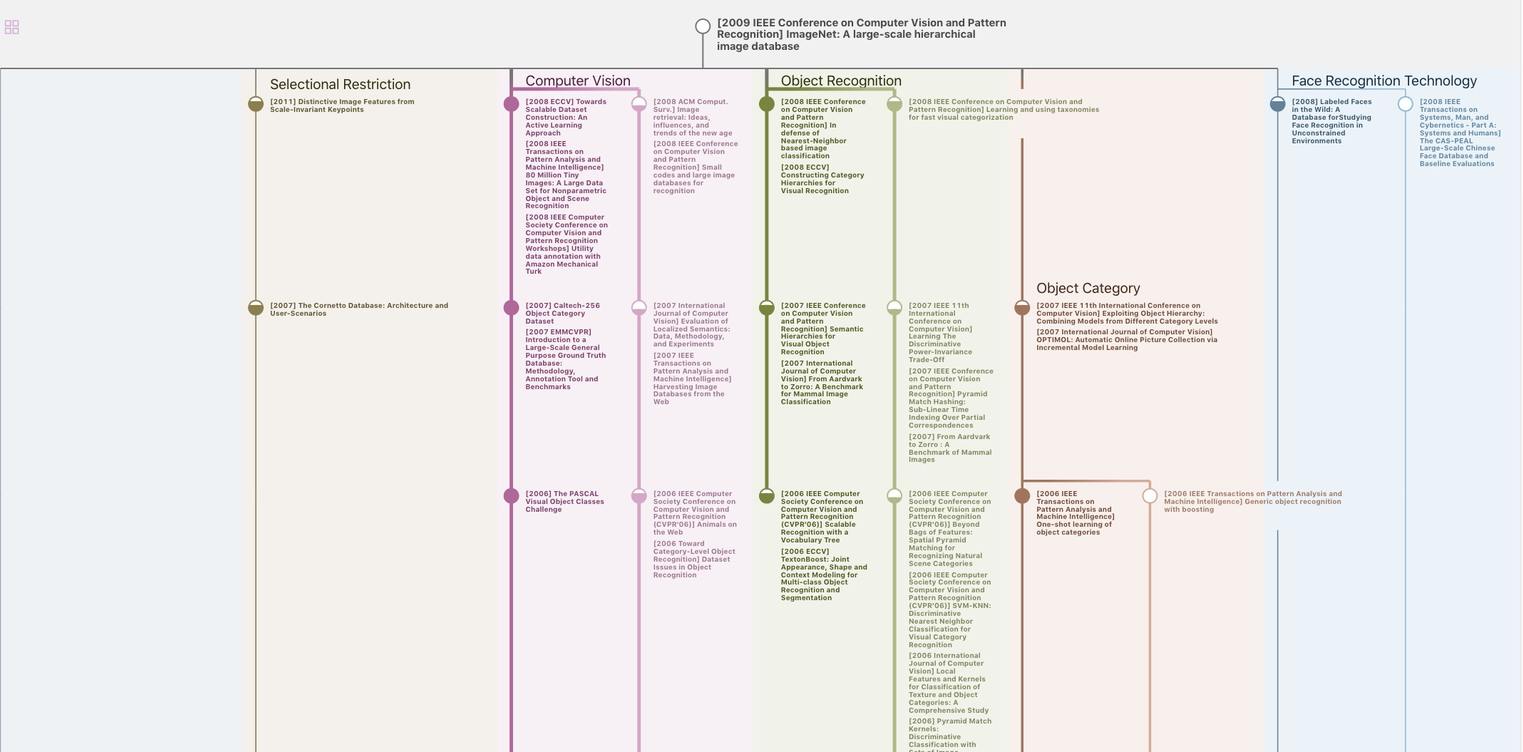
生成溯源树,研究论文发展脉络
Chat Paper
正在生成论文摘要