A unifying model to predict variable drug response for personalised medicine
biorxiv(2020)
摘要
Pharmacogenomics is a key component of personalized medicine. It promises a safer and more effective drug treatment by individualizing the choice of drug and dose based on an individual’s genetic profile. The majority of commonly prescribed drugs are metabolized by a small set of Cytochrome P450 (CYP) enzymes. In clinical practice, genetic biomarkers are being used to categorize patients into predefined *-alleles to predict CYP450 enzyme activity and adjust drug dosages accordingly. Yet, this approach has important limitations as it leaves a large part of variability in drug response unexplained. Here, we present a novel approach and introduce a continuous scale (instead of categorical) assignments to predict metabolic enzyme activity. The proposed strategy uses full gene sequencing data, a neural network model and CYP2D6 mediated tamoxifen metabolism from a prospective study of 561 breast cancer patients. The model explains 79% of the interindividual variability in CYP2D6 activity compared to 5% with the conventional approach. It is capable of assigning accurate enzyme activity to alleles containing previously uncharacterized combinations of variants and were replicated in an independent cohort of tamoxifen treated patients, a cohort of Venlafaxine users as well as in vitro functional assays using HEK cells. These results demonstrate the advantage of a continuous scale and a completely phased genotype for prediction of CYP450 enzyme activity and thereby enables more accurate prediction of individual drug response.
更多查看译文
关键词
Machine learning,pharmacogenomics,CYP2D6,long-read sequencing,Tamoxifen metabolism,breast cancer,Venlafaxine,neural networks
AI 理解论文
溯源树
样例
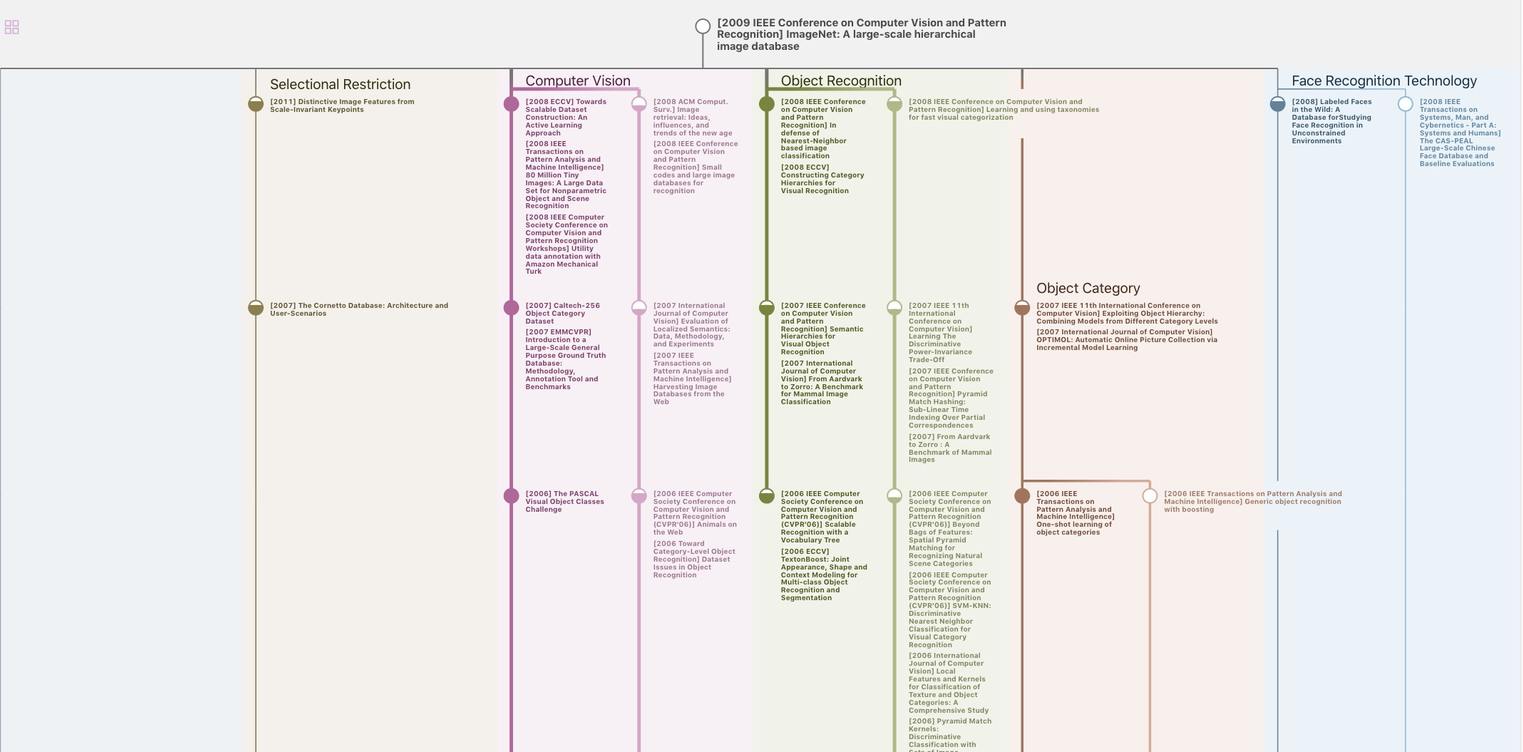
生成溯源树,研究论文发展脉络
Chat Paper
正在生成论文摘要