Disentangling latent representations of single cell RNA-seq experiments
biorxiv(2020)
摘要
Single cell RNA sequencing (scRNA-seq) enables transcriptional profiling at the resolution of individual cells. These experiments measure features at the level of transcripts, but biological processes of interest often involve the complex coordination of many individual transcripts. It can therefore be difficult to extract interpretable insights directly from transcript-level cell profiles. Latent representations which capture biological variation in a smaller number of dimensions are therefore useful in interpreting many experiments. Variational autoencoders (VAEs) have emerged as a tool for scRNA-seq denoising and data harmonization, but the correspondence between latent dimensions in these models and generative factors remains unexplored. Here, we explore training VAEs with modifications to the objective function (i.e. -VAE) to encourage disentanglement and make latent representations of single cell RNA-seq data more interpretable. Using simulated data, we find that VAE latent dimensions correspond more directly to data generative factors when using these modified objective functions. Applied to experimental data of stimulated peripheral blood mononuclear cells, we find better correspondence of latent dimensions to experimental factors and cell identity programs, but impaired performance on cell type clustering.
更多查看译文
关键词
single cell RNA-seq,variational autoencoder,VAE,disentangle
AI 理解论文
溯源树
样例
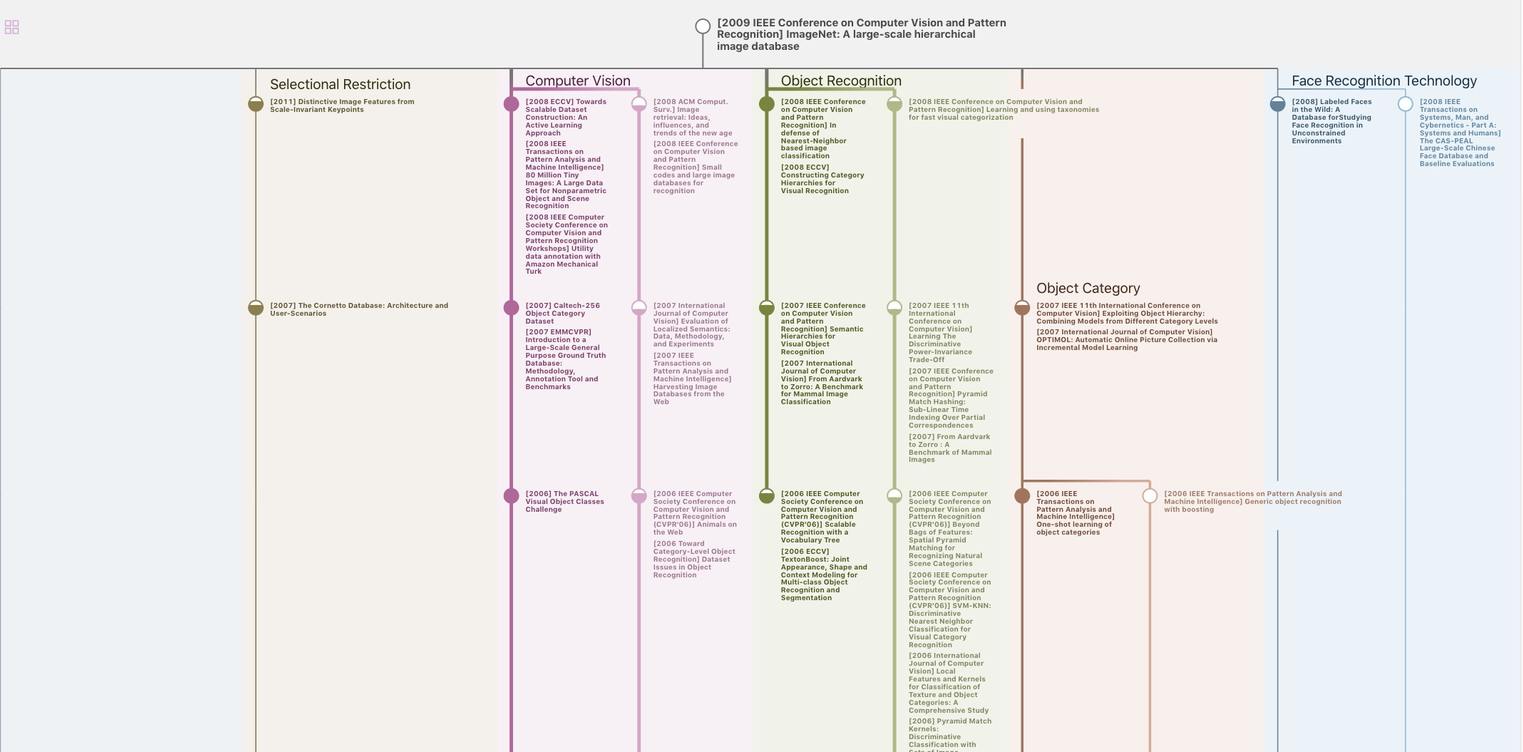
生成溯源树,研究论文发展脉络
Chat Paper
正在生成论文摘要