Data-driven learning how oncogenic gene expression locally alters heterocellular networks
biorxiv(2020)
摘要
Discovering and developing pharmaceutical drugs increasingly relies on mechanistic mathematical modeling and simulation. In immuno-oncology, models that capture causal relations among genetic drivers of oncogenesis, functional plasticity, and host immunity provide an important complement to wet experiments, given the cellular complexity and dynamics within the tumor microenvironment. Unfortunately, formulating such mechanistic cell-level models currently relies on hand curation by experts, which can bias how data is interpreted or the priority of drug targets. In modeling molecular-level networks, rules and algorithms have been developed to limit a priori biases in formulating mechanistic models. To realize an equivalent approach for cell-level networks, we combined digital cytometry with Bayesian network inference to generate causal models that link an increase in gene expression associated with oncogenesis with alterations in stromal and immune cell subsets directly from bulk transcriptomic datasets. To illustrate, we predicted how an increase in expression of Cell Communication Network factor 4 (CCN4/WISP1) altered the tumor microenvironment using data from patients diagnosed with breast cancer and melanoma. Network predictions were then tested using two immunocompetent mouse models for melanoma. In contrast to hand-curated approaches, we posit that combining digital cytometry with Bayesian network inference provides a less biased approach for elaborating mechanistic cell-level models directly from data.
更多查看译文
关键词
Heterocellular networks,digital cytometry,deconvolution,anti-tumor immunity,Bayesian network inference,functional plasticity
AI 理解论文
溯源树
样例
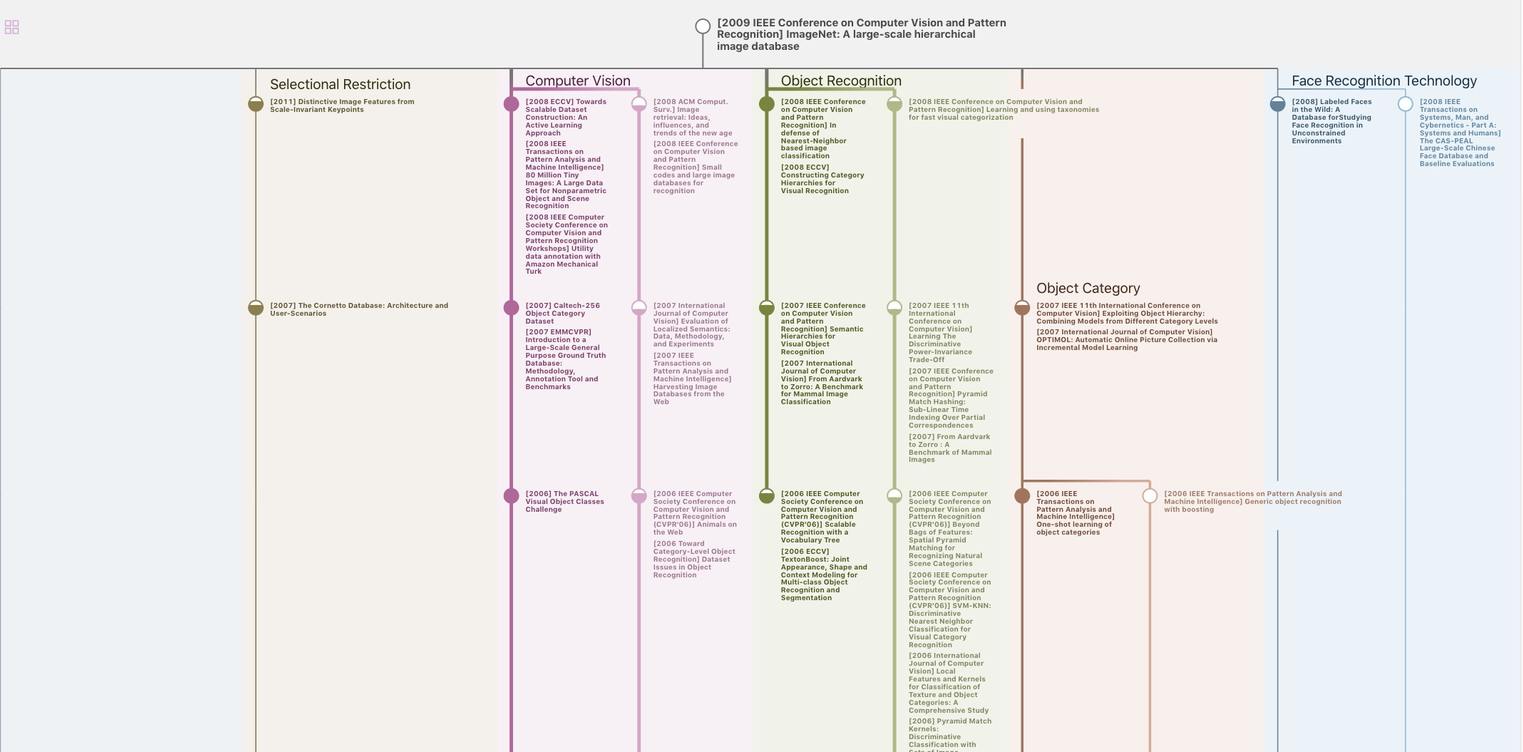
生成溯源树,研究论文发展脉络
Chat Paper
正在生成论文摘要