Identifying behavioral structure from deep variational embeddings of animal motion
Communications Biology(2022)
摘要
Quantification and detection of the hierarchical organization of behavior is a major challenge in neuroscience. Recent advances in markerless pose estimation enable the visualization of high-dimensional spatiotemporal behavioral dynamics of animal motion. However, robust and reliable technical approaches are needed to uncover underlying structure in these data and to segment behavior into discrete hierarchically organized motifs. Here, we present an unsupervised probabilistic deep learning framework that identifies behavioral structure from deep variational embeddings of animal motion (VAME). By using a mouse model of beta amyloidosis as a use case, we show that VAME not only identifies discrete behavioral motifs, but also captures a hierarchical representation of the motif’s usage. The approach allows for the grouping of motifs into communities and the detection of differences in community-specific motif usage of individual mouse cohorts that were undetectable by human visual observation. Thus, we present a robust approach for the segmentation of animal motion that is applicable to a wide range of experimental setups, models and conditions without requiring supervised or a-priori human interference.
更多查看译文
关键词
animal motion,deep variational embeddings,behavioral structure
AI 理解论文
溯源树
样例
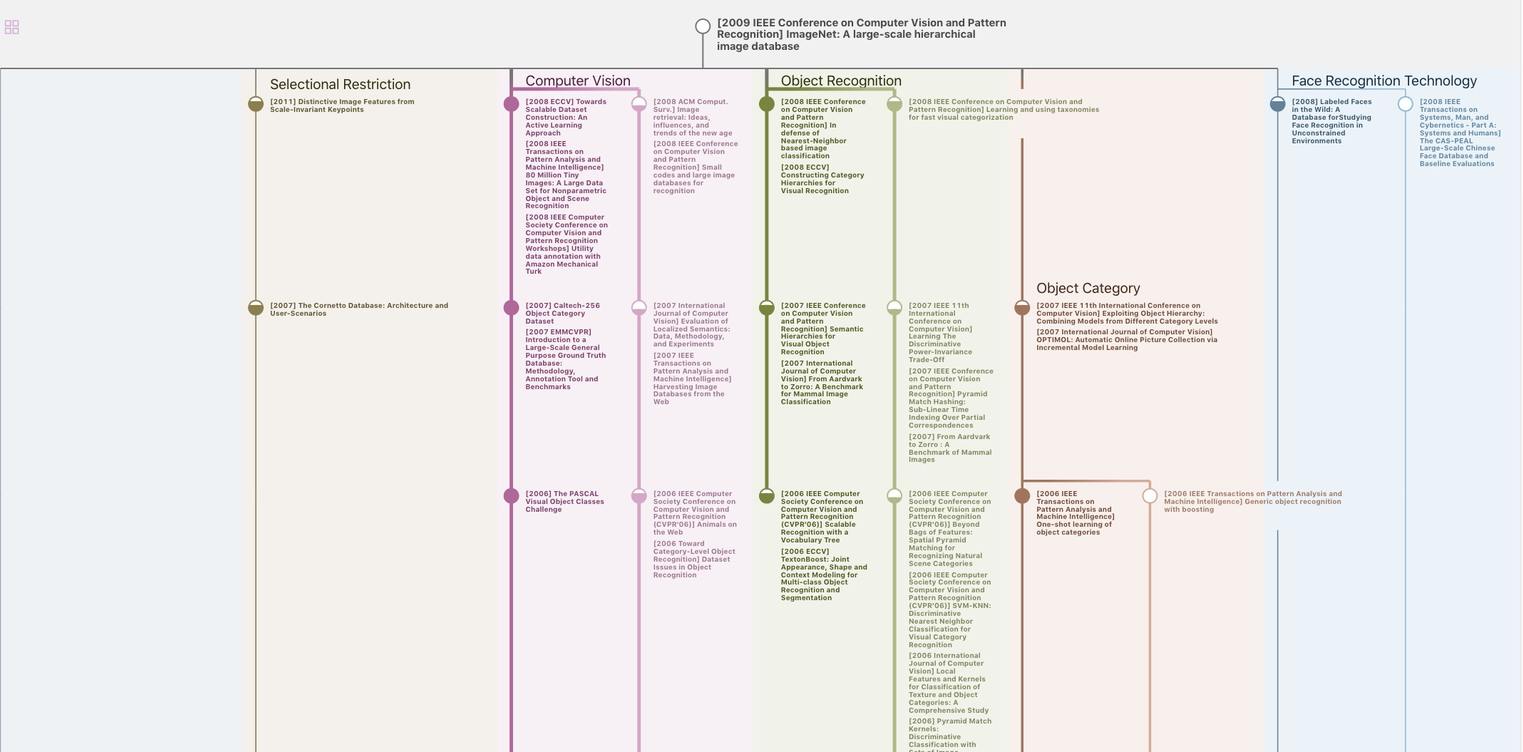
生成溯源树,研究论文发展脉络
Chat Paper
正在生成论文摘要