Resilient In-Season Crop Type Classification in Multispectral Satellite Observations using Growth Stage Normalization
CoRR(2020)
摘要
Crop type classification using satellite observations is an important tool for providing insights about planted area and enabling estimates of crop condition and yield, especially within the growing season when uncertainties around these quantities are highest. As the climate changes and extreme weather events become more frequent, these methods must be resilient to changes in domain shifts that may occur, for example, due to shifts in planting timelines. In this work, we present an approach for within-season crop type classification using moderate spatial resolution (30 m) satellite data that addresses domain shift related to planting timelines by normalizing inputs by crop growth stage. We use a neural network leveraging both convolutional and recurrent layers to predict if a pixel contains corn, soybeans, or another crop or land cover type. We evaluated this method for the 2019 growing season in the midwestern US, during which planting was delayed by as much as 1-2 months due to extreme weather that caused record flooding. We show that our approach using growth stage-normalized time series outperforms fixed-date time series, and achieves overall classification accuracy of 85.4% prior to harvest (September-November) and 82.8% by mid-season (July-September).
更多查看译文
关键词
multispectral satellite observations,growth stage normalization,classification,in-season
AI 理解论文
溯源树
样例
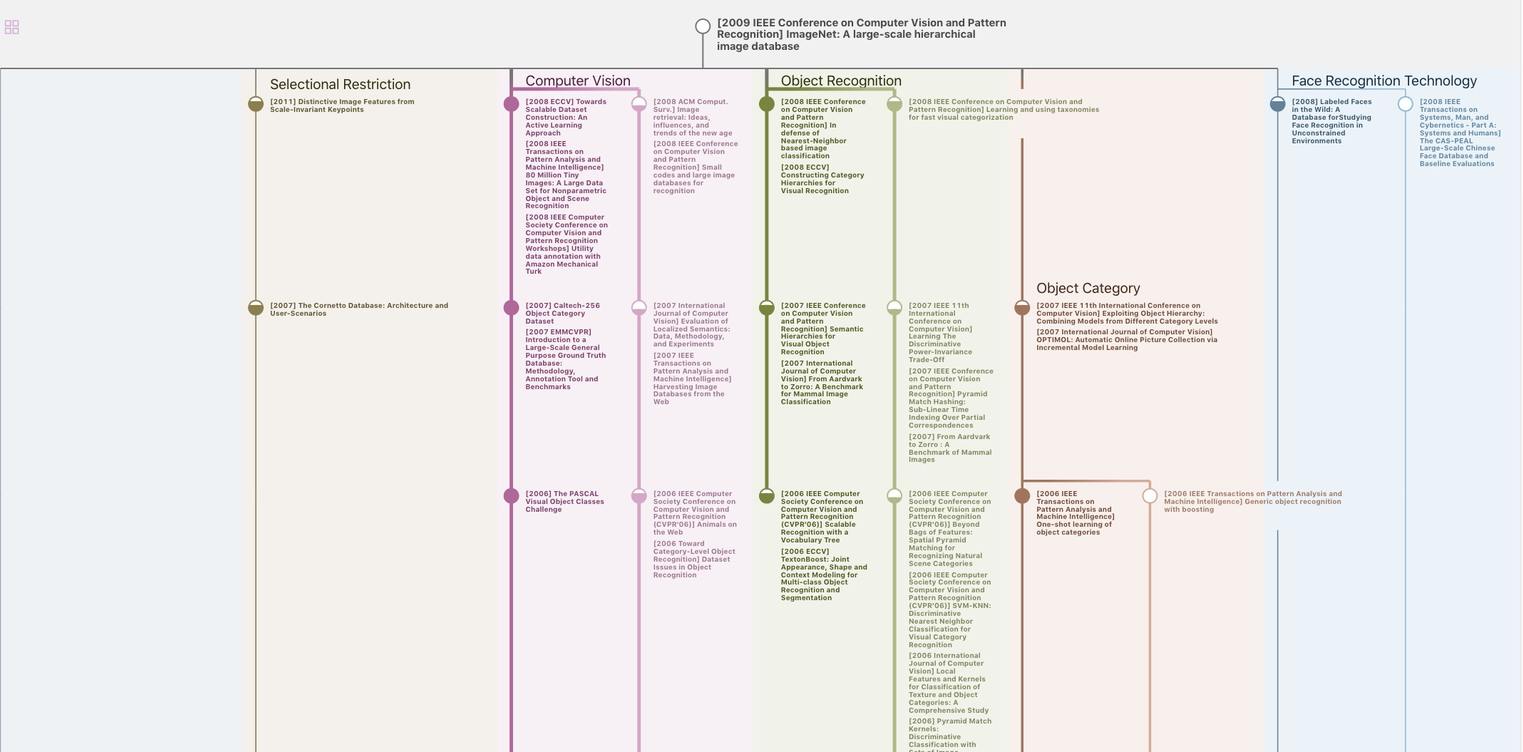
生成溯源树,研究论文发展脉络
Chat Paper
正在生成论文摘要