Pennsyn2real: Training Object Recognition Models Without Human Labeling
IEEE ROBOTICS AND AUTOMATION LETTERS(2021)
摘要
Scalable training data generation is a critical problem in deep learning. We propose PennSyn2Real - a photo-realistic synthetic dataset consisting of more than 100 000 4K images of more than 20 types of micro aerial vehicles (MAVs). The dataset can be used to generate arbitrary numbers of training images for high-level computer vision tasks such as MAV detection and classification. Our data generation framework bootstraps chroma-keying, a mature cinematography technique, with a motion tracking system providing artifact-free and curated annotated images. Our system, therefore, allows object orientations and lighting to be controlled. This framework is easy to set up and can be applied to a broad range of objects, reducing the gap between synthetic and real-world data. We show that synthetic data generated using this framework can be directly used to train CNN models for common object recognition tasks such as detection and segmentation. We demonstrate competitive performance in comparison with training using only real images. Furthermore, bootstrapping the generated synthetic data in few-shot learning can significantly improve the overall performance, reducing the number of required training data samples to achieve the desired accuracy.
更多查看译文
关键词
Object detection, segmentation and categorization, aerial systems, perception and autonomy, surveillance robotic systems, data sets for robotic vision
AI 理解论文
溯源树
样例
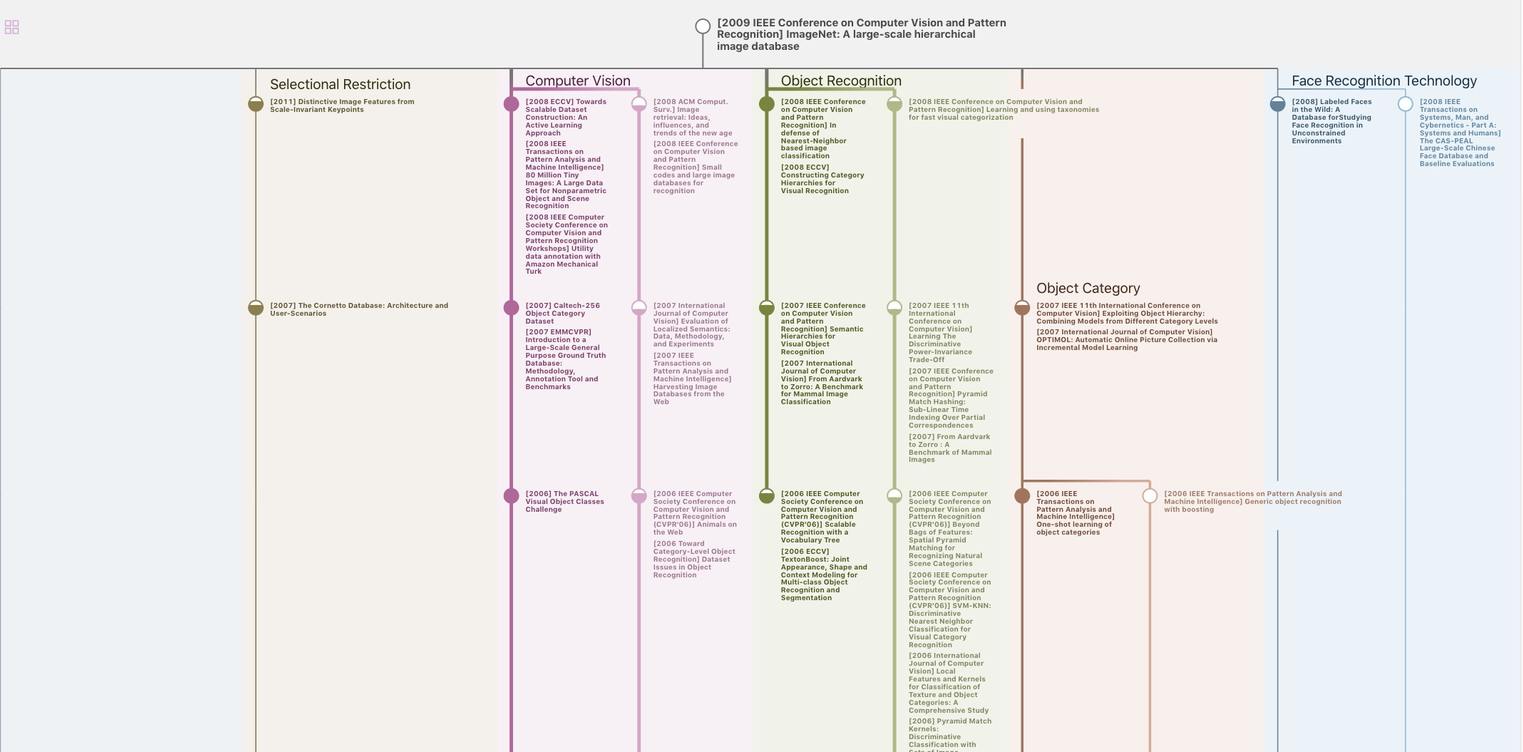
生成溯源树,研究论文发展脉络
Chat Paper
正在生成论文摘要