Partially Observable Online Change Detection via Smooth-Sparse Decomposition
arxiv(2020)
摘要
We consider online change detection of high dimensional data streams with sparse changes, where only a subset of data streams can be observed at each sensing time point due to limited sensing capacities. On the one hand, the detection scheme should be able to deal with partially observable data and meanwhile have efficient detection power for sparse changes. On the other, the scheme should be able to adaptively and actively select the most important variables to observe to maximize the detection power. To address these two points, in this paper, we propose a novel detection scheme called CDSSD. In particular, it describes the structure of high dimensional data with sparse changes by smooth-sparse decomposition, whose parameters can be learned via spike-slab variational Bayesian inference. Then the posterior Bayes factor, which incorporates the learned parameters and sparse change information, is formulated as a detection statistic. Finally, by formulating the statistic as the reward of a combinatorial multi-armed bandit problem, an adaptive sampling strategy based on Thompson sampling is proposed. The efficacy and applicability of our method in practice are demonstrated with numerical studies and a real case study.
更多查看译文
关键词
observable online change detection,smooth-sparse
AI 理解论文
溯源树
样例
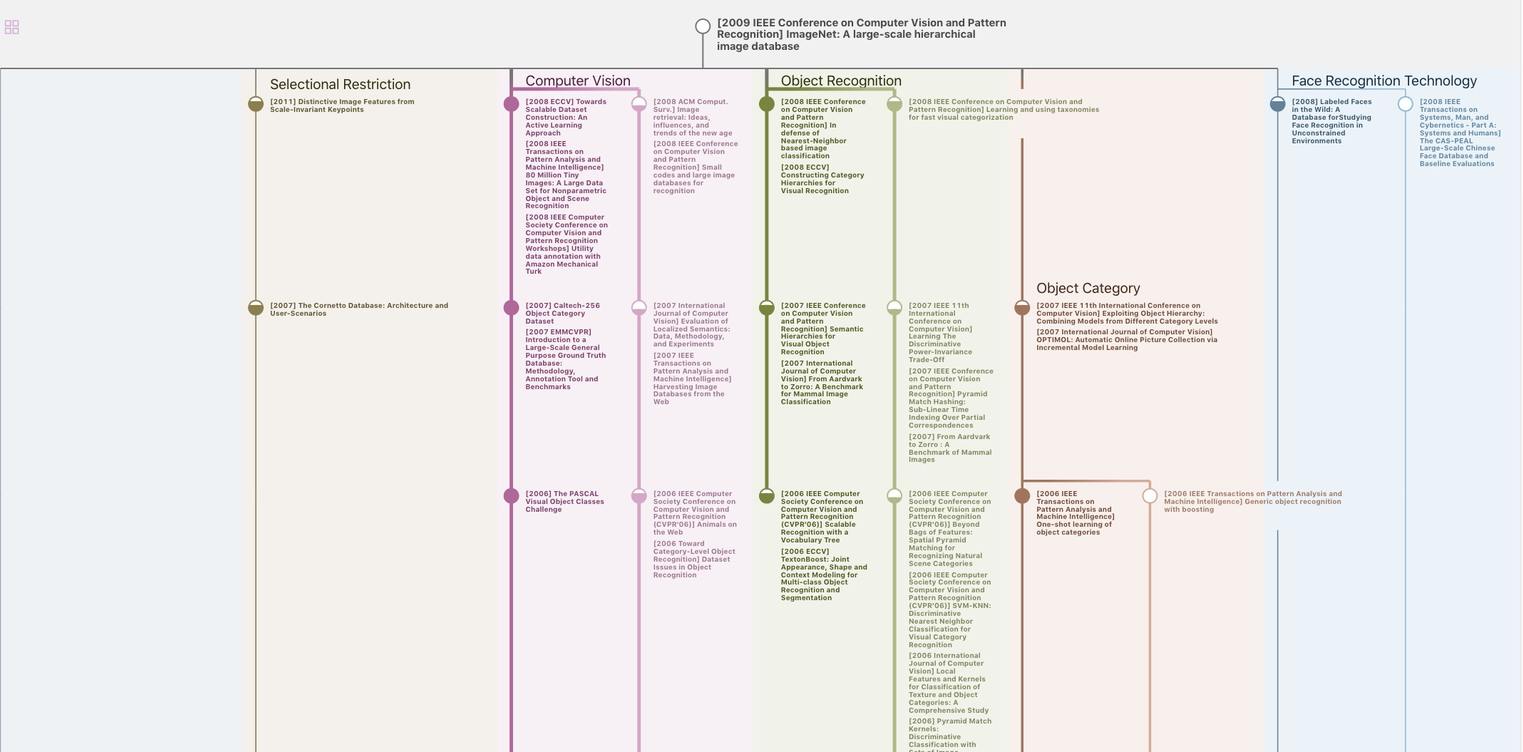
生成溯源树,研究论文发展脉络
Chat Paper
正在生成论文摘要