Wheat Diseases Classification and Localization Using Convolutional Neural Networks and GradCAM Visualization
2020 International Conference on Intelligent Systems and Computer Vision (ISCV)(2020)
摘要
The world has been witnessing a population boom that has several implications including food security. Wheat is one of the world’s most important crops in terms of production and consumption, and demand for it is increasing. On the other hand, diseases can damage the abundance and the quality of the crop, so this needs to be revealed through advanced methods. In recent years, along with the various technological developments, using Convolutional Neural Networks (CNN) has proved to be showing great results in many image classification tasks. However, deep learning models are generally considered as black boxes and it is difficult to understand what the model has learned. The purpose of this article is to detect diseases from wheat images using CNNs and to use visualization methods to understand what these models have learned. For this reason, a wheat database has been collected by CRA-W (Walloon Agricultural Research Center), which contains 1163 images and is classified into two groups namely sick and healthy. Moreover, we propose to use the mask R-CNN for segmentation and extraction of wheat spikes from the background. Furthermore, a visualization and interpretation method, namely Gradient-weighted Class Activation Mapping (GradCAM), is used to locate the disease on the wheat spikes in a non-supervised way. GradCAM is actually used generally to highlight the most important regions from the CNN model’s viewpoint that are used to perform the classification.
更多查看译文
关键词
Diseases,Agriculture,Task analysis,Image segmentation,Convolutional neural networks,Machine learning,Databases
AI 理解论文
溯源树
样例
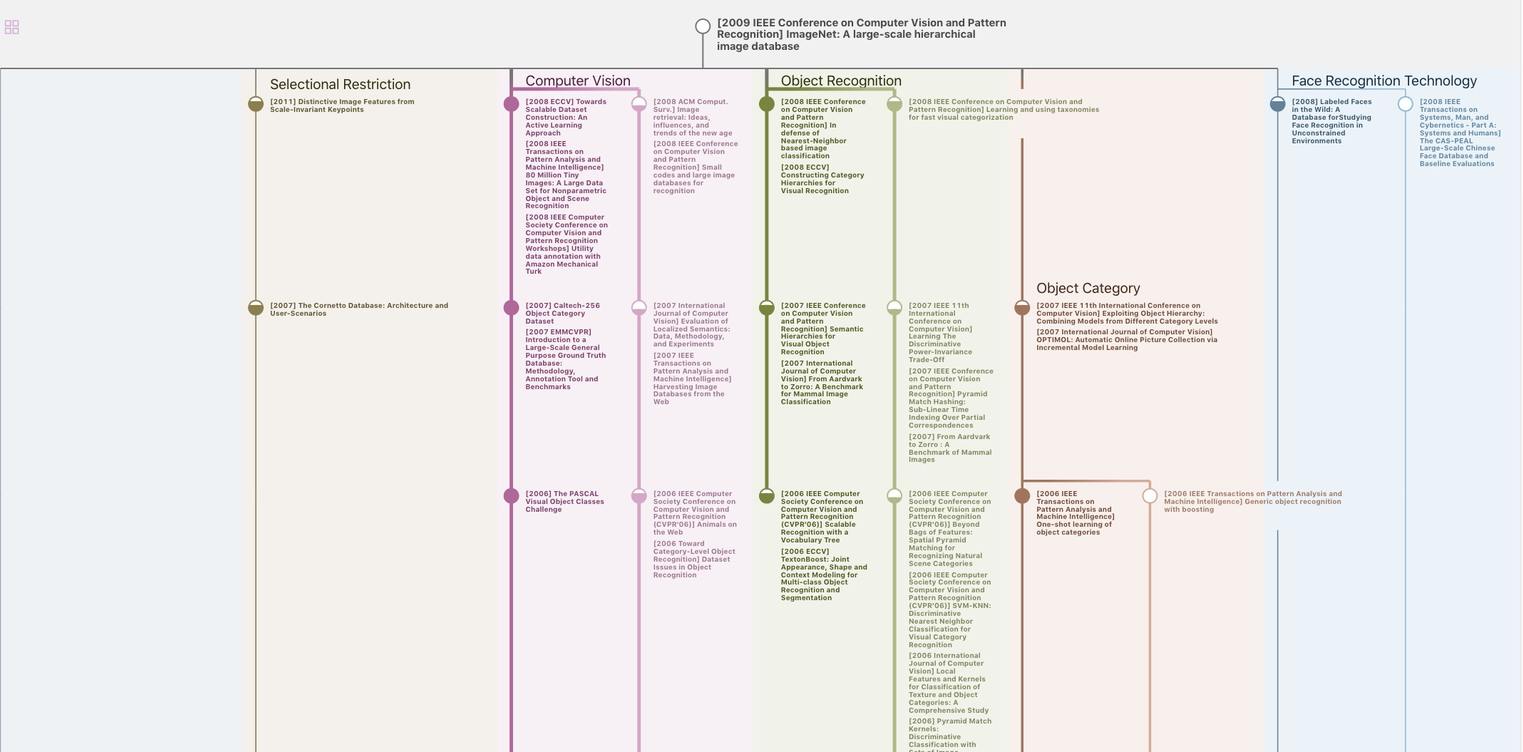
生成溯源树,研究论文发展脉络
Chat Paper
正在生成论文摘要