Dynamic Similarity Search on Integer Sketches
2020 IEEE International Conference on Data Mining (ICDM)(2020)
摘要
Similarity-preserving hashing is a core technique for fast similarity searches, and it randomly maps data points in a metric space to strings of discrete symbols (i.e., sketches) in the Hamming space. While traditional hashing techniques produce binary sketches, recent ones produce integer sketches for preserving various similarity measures. However, most similarity search methods are designed for binary sketches and inefficient for integer sketches. Moreover, most methods are either inapplicable or inefficient for dynamic datasets, although modern real-world datasets are updated over time. We propose dynamic filter trie (DyFT), a dynamic similarity search method for both binary and integer sketches. An extensive experimental analysis using large real-world datasets shows that DyFT performs superiorly with respect to scalability, time performance, and memory efficiency. For example, on a huge dataset of 216 million data points, DyFT performs a similarity search 6,000 times faster than a state-of-the-art method while reducing to one-thirteenth in memory.
更多查看译文
关键词
Trie, Hamming distance, Data structure, Information retrieval
AI 理解论文
溯源树
样例
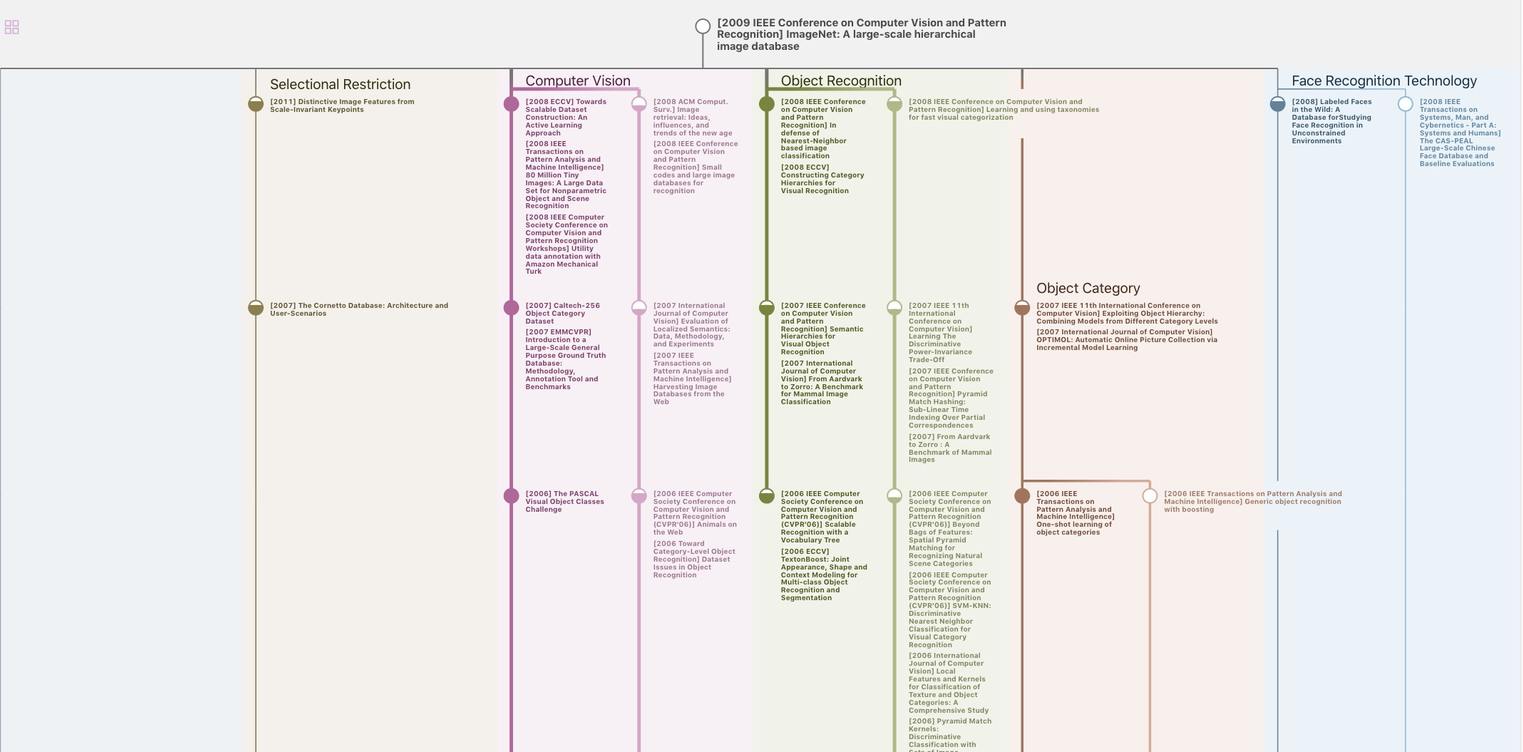
生成溯源树,研究论文发展脉络
Chat Paper
正在生成论文摘要