Adaptive Multi-Type Fingerprint Indoor Positioning and Localization Method Based on Multi-Task Learning and Weight Coefficients K-Nearest Neighbor.
Italian National Conference on Sensors(2020)
摘要
The complex indoor environment makes the use of received fingerprints unreliable as an indoor positioning and localization method based on fingerprint data. This paper proposes an adaptive multi-type fingerprint indoor positioning and localization method based on multi-task learning (MTL) and Weight Coefficients K-Nearest Neighbor (WCKNN), which integrates magnetic field, Wi-Fi and Bluetooth fingerprints for positioning and localization. The MTL fuses the features of different types of fingerprints to search the potential relationship between them. It also exploits the synergy between the tasks, which can boost up positioning and localization performance. Then the WCKNN predicts another position of the fingerprints in a certain class determined by the obtained location. The final position is obtained by fusing the predicted positions using a weighted average method whose weights are the positioning errors provided by positioning error prediction models. Experimental results indicated that the proposed method achieved 98.58% accuracy in classifying locations with a mean positioning error of 1.95 m.
更多查看译文
关键词
multi-type fingerprints,indoor positioning and localization,multi-task learning,weight coefficients K-Nearest neighbor
AI 理解论文
溯源树
样例
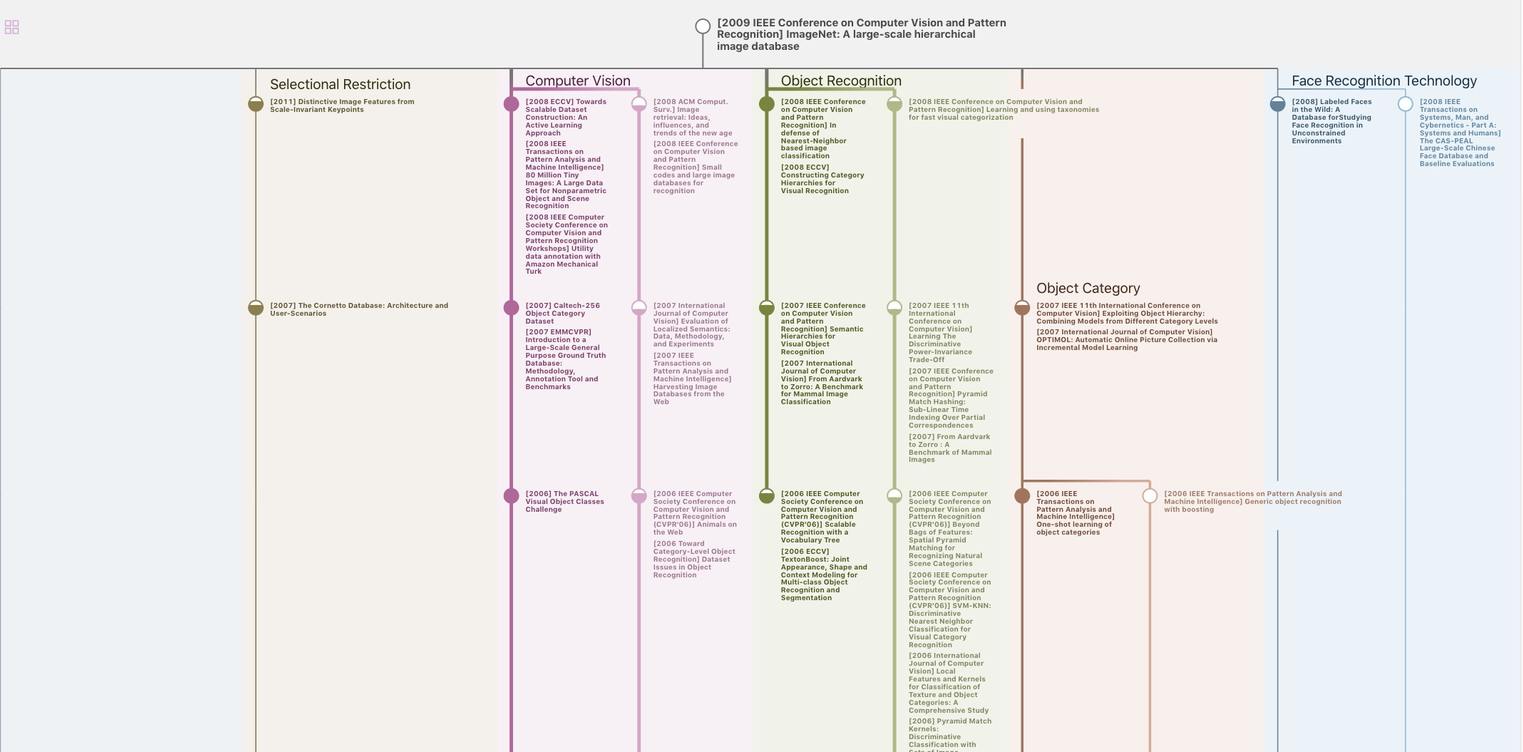
生成溯源树,研究论文发展脉络
Chat Paper
正在生成论文摘要