A Little Goes a Long Way: Improving Toxic Language Classification Despite Data Scarcity
arXiv (Cornell University)(2020)
摘要
Detection of some types of toxic language is hampered by extreme scarcity of labeled training data. Data augmentation - generating new synthetic data from a labeled seed dataset - can help. The efficacy of data augmentation on toxic language classification has not been fully explored. We present the first systematic study on how data augmentation techniques impact performance across toxic language classifiers, ranging from shallow logistic regression architectures to BERT - a state-of-the-art pre-trained Transformer network. We compare the performance of eight techniques on very scarce seed datasets. We show that while BERT performed the best, shallow classifiers performed comparably when trained on data augmented with a combination of three techniques, including GPT-2-generated sentences. We discuss the interplay of performance and computational overhead, which can inform the choice of techniques under different constraints.
更多查看译文
关键词
Part-of-Speech Tagging,Natural Language Processing,Language Modeling,Statistical Machine Translation
AI 理解论文
溯源树
样例
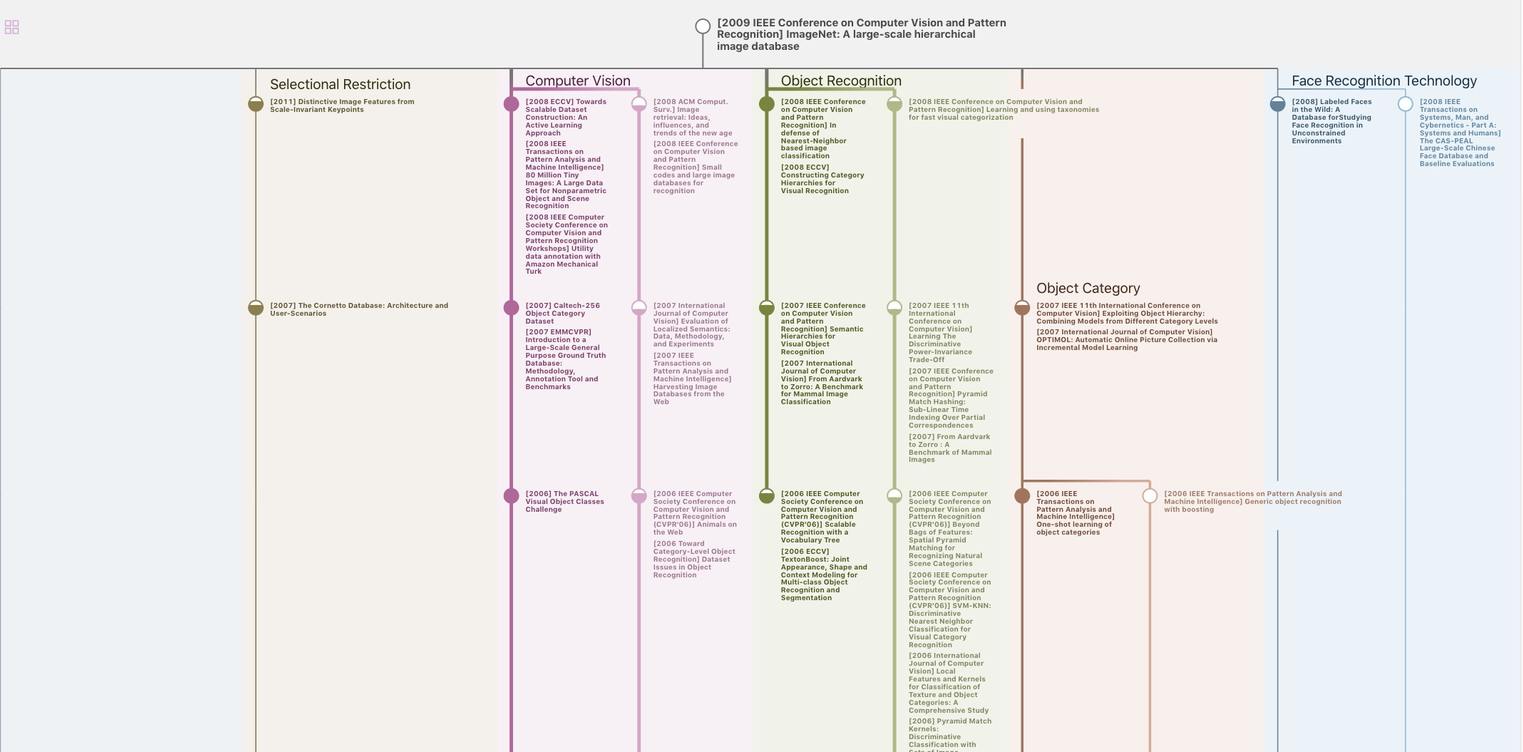
生成溯源树,研究论文发展脉络
Chat Paper
正在生成论文摘要