Multi-Agent Bootstrapped Deep Q-Network for Large-Scale Traffic Signal Control
2020 IEEE Conference on Control Technology and Applications (CCTA)(2020)
摘要
Deep reinforcement learning (RL) has demonstrated promising performance for adaptive traffic signal control (ATSC) in simulated environments. However, it is infeasible to apply Deep RL for real-world traffic systems mainly due to the lack of a large amount of high-quality data. In this paper, we introduce efficient exploration to improve sample-efficiency and robustness of RL training, making deep RL more practical for large-scale ATSC. Specifically, we first adopt the bootstrapped Deep Q-Network (DQN) algorithm to induce exploration via an ensemble of behavior policies, and it outperforms the vanilla DQN in both efficiency and robustness on a handcrafted asymmetric isolated intersection. Further, we develop a multi-agent DQN structure that enables conditional parameter sharing of bootstrapped DQN for large-scale problems. Finally, we demonstrate the effectiveness of our multi-agent approach on a large-scale
$\mathbf{5}\times \mathbf{5}$
synthetic traffic grid with 25 intersections.
更多查看译文
关键词
Training,Additives,Learning (artificial intelligence),Mathematical model,Data models,Neural networks,Robustness
AI 理解论文
溯源树
样例
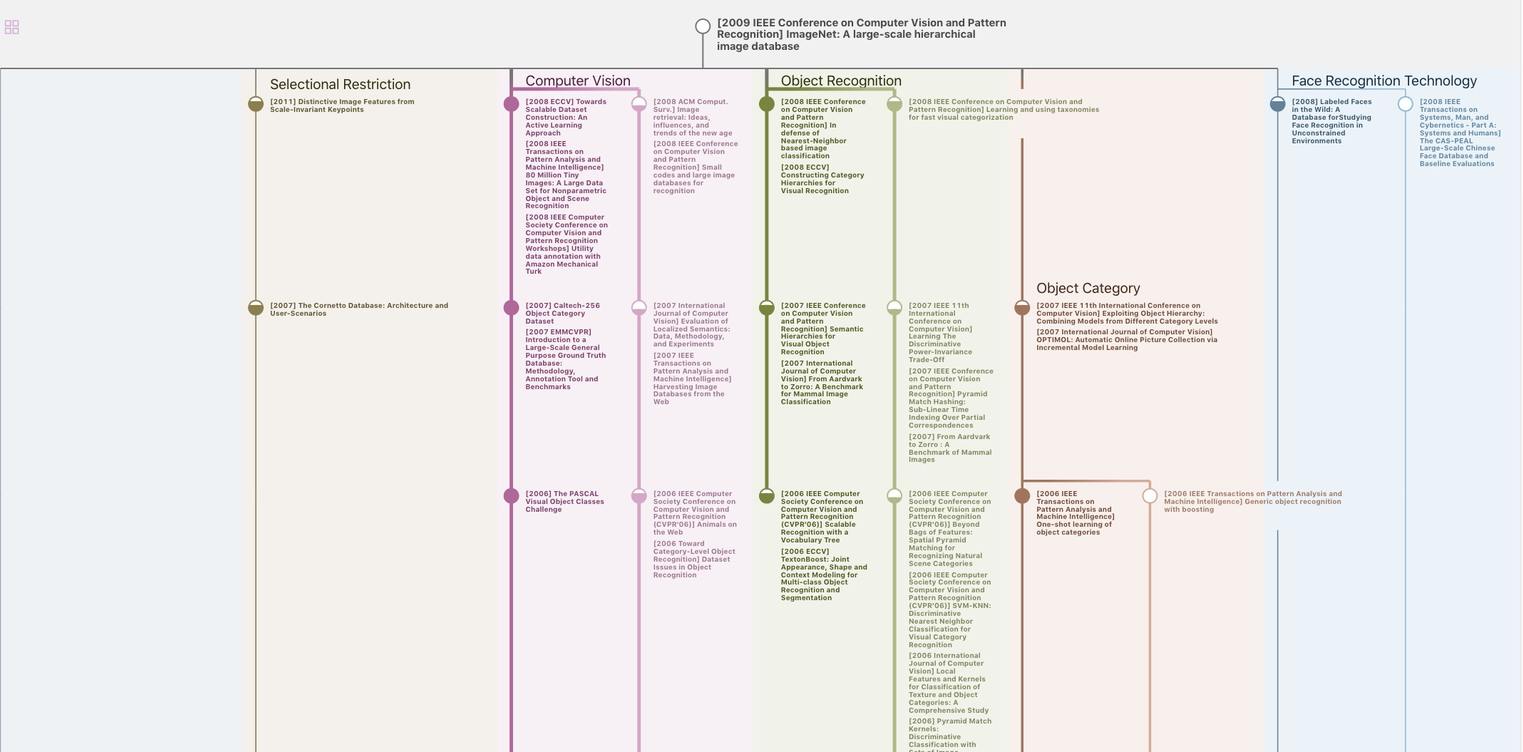
生成溯源树,研究论文发展脉络
Chat Paper
正在生成论文摘要