Dynamic Global-Local Attention Network Based On Capsules for Text Classification
2020 International Joint Conference on Neural Networks (IJCNN)(2020)
摘要
Text classification requires a comprehensive consideration of global and local information for the text. However, most methods only treat the global and local features of the text as two separate parts and ignore the relationship between them. In this paper, we propose a Dynamic Global-Local Attention Network based on Capsules (DGLA) that can use global features to dynamically adjust the importance of local features (e.g., sentence-level features or phrase-level features). The global features of the text are extracted by the capsule network, which can capture the mutual positional relationship of the input features to mine more hidden information. Furthermore, we have designed two global-local attention mechanisms within DGLA to measure the importance of two different local features and effectively leverage the advantages of these two attention mechanisms through the residual network. The performance of the model was evaluated on seven benchmark text classification datasets, and DGLA achieved the highest accuracy on all datasets. Ablation experiments show that the global-local attention mechanism can significantly improve the performance of the model.
更多查看译文
关键词
Feature extraction,Semantics,Routing,Convolutional neural networks,Data mining,Natural language processing
AI 理解论文
溯源树
样例
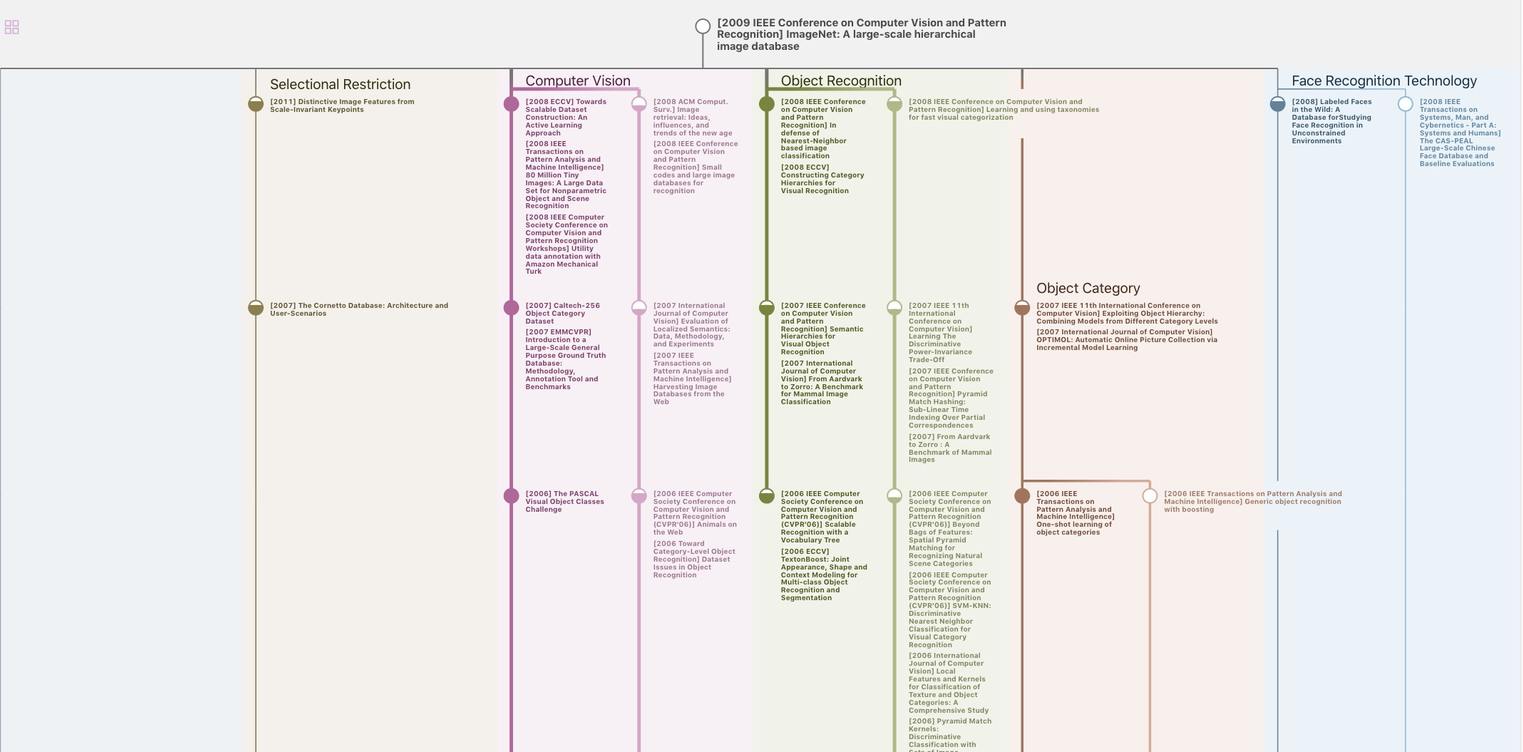
生成溯源树,研究论文发展脉络
Chat Paper
正在生成论文摘要