Gradients Of Connectivity As Graph Fourier Bases Of Brain Activity
NETWORK NEUROSCIENCE(2021)
摘要
The application of graph theory to model the complex structure and function of the brain has shed new light on its organization, prompting the emergence of network neuroscience. Despite the tremendous progress that has been achieved in this field, still relatively few methods exploit the topology of brain networks to analyze brain activity. Recent attempts in this direction have leveraged on the one hand graph spectral analysis (to decompose brain connectivity into eigenmodes or gradients) and the other graph signal processing (to decompose brain activity "coupled to" an underlying network in graph Fourier modes). These studies have used a variety of imaging techniques (e.g., fMRI, electroencephalography, diffusion-weighted and myelin-sensitive imaging) and connectivity estimators to model brain networks. Results are promising in terms of interpretability and functional relevance, but methodologies and terminology are variable. The goals of this paper are twofold. First, we summarize recent contributions related to connectivity gradients and graph signal processing, and attempt a clarification of the terminology and methods used in the field, while pointing out current methodological limitations. Second, we discuss the perspective that the functional relevance of connectivity gradients could be fruitfully exploited by considering them as graph Fourier bases of brain activity.
更多查看译文
关键词
Graph signal processing, Connectivity gradients, Graph Fourier transform, Laplacian, Network neuroscience, Neuroimaging
AI 理解论文
溯源树
样例
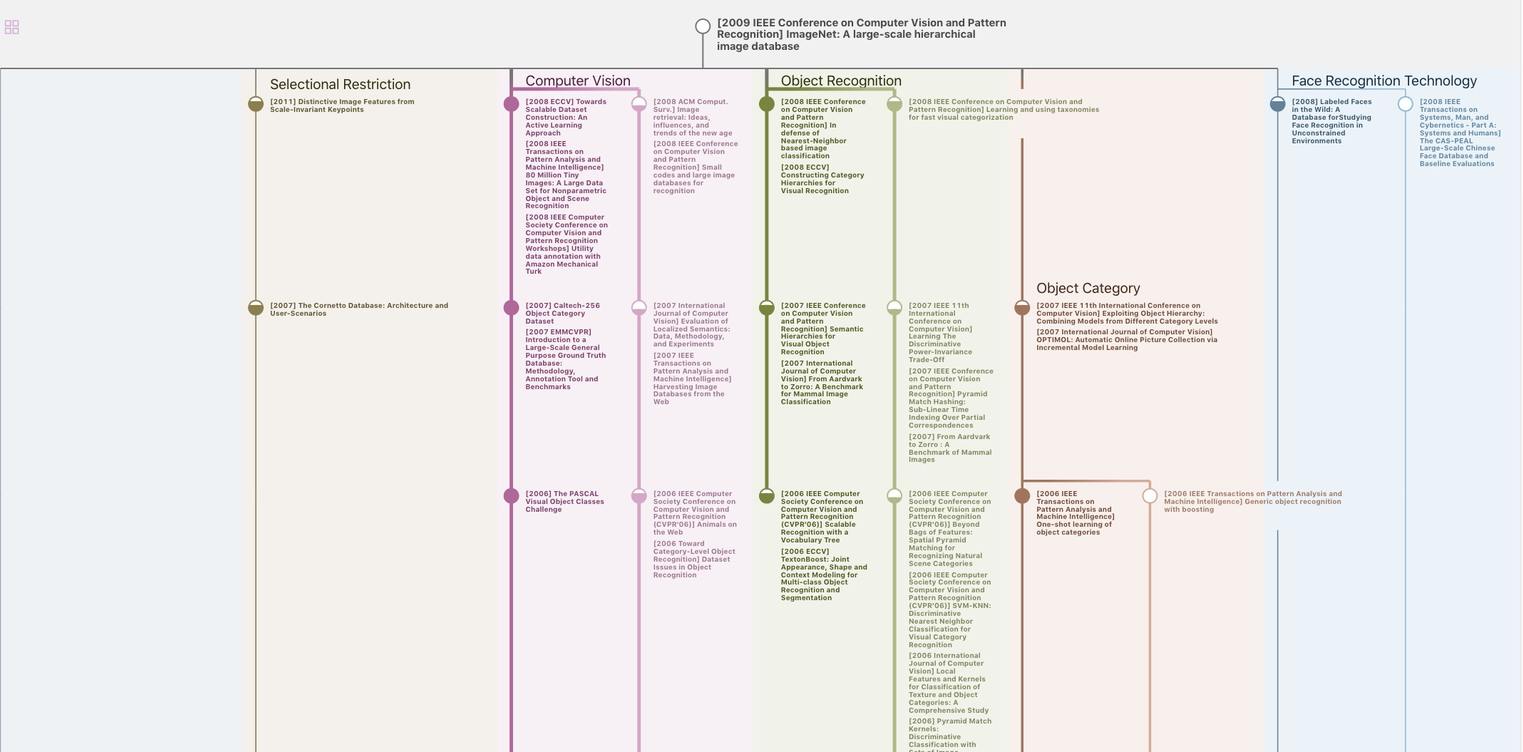
生成溯源树,研究论文发展脉络
Chat Paper
正在生成论文摘要