A New Method Based on Matrix Completion and Non-Negative Matrix Factorization for Predicting Disease-Associated Mirnas.
IEEE/ACM transactions on computational biology and bioinformatics(2022)
摘要
Numerous studies have shown that microRNAs are associated with the occurrence and development of human diseases. Thus, studying disease-associated miRNAs is significantly valuable to the prevention, diagnosis and treatment of diseases. In this paper, we proposed a novel method based on matrix completion and non-negative matrix factorization (MCNMF)for predicting disease-associated miRNAs. Due to the information inadequacy on miRNA similarities and disease similarities, we calculated the latter via two models, and introduced the Gaussian interaction profile kernel similarity. In addition, the matrix completion (MC)was employed to further replenish the miRNA and disease similarities to improve the prediction performance. And to reduce the sparsity of miRNA-disease association matrix, the method of weighted K nearest neighbor (WKNKN)was used, which is a pre-processing step. We also utilized non-negative matrix factorization (NMF)using dual L2,1-norm, graph Laplacian regularization, and Tikhonov regularization to effectively avoid the overfitting during the prediction. Finally, several experiments and a case study were implemented to evaluate the effectiveness and performance of the proposed MCNMF model. The results indicated that our method could reliably and effectively predict disease-associated miRNAs.
更多查看译文
关键词
L-2,(1)-norm,matrix completion,miRNA-disease association,non-negative matrix factorization
AI 理解论文
溯源树
样例
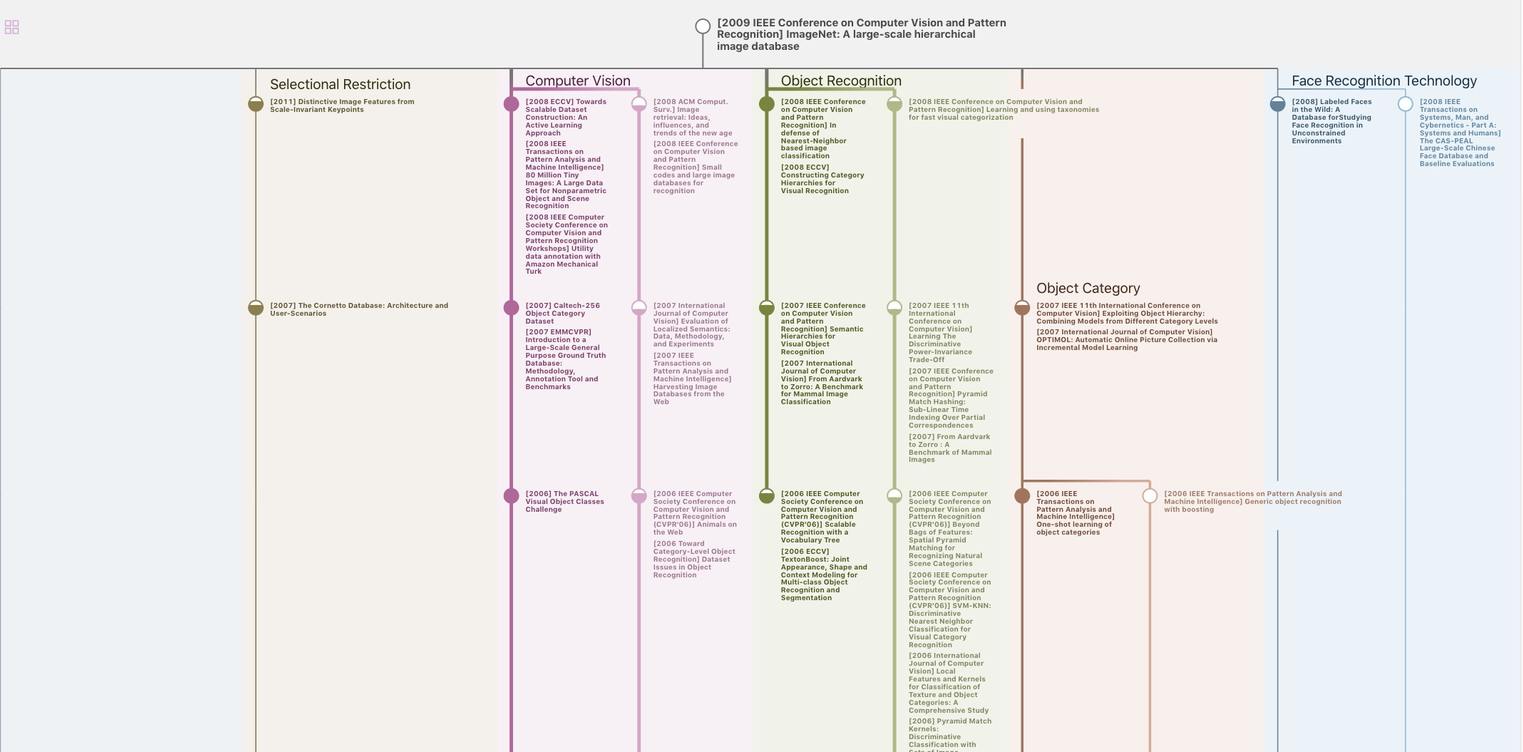
生成溯源树,研究论文发展脉络
Chat Paper
正在生成论文摘要