Kernel learning approaches for summarising and combining posterior similarity matrices
arXiv (Cornell University)(2020)
摘要
When using Markov chain Monte Carlo (MCMC) algorithms to perform inference for Bayesian clustering models, such as mixture models, the output is typically a sample of clusterings (partitions) drawn from the posterior distribution. In practice, a key challenge is how to summarise this output. Here we build upon the notion of the posterior similarity matrix (PSM) in order to suggest new approaches for summarising the output of MCMC algorithms for Bayesian clustering models. A key contribution of our work is the observation that PSMs are positive semi-definite, and hence can be used to define probabilistically-motivated kernel matrices that capture the clustering structure present in the data. This observation enables us to employ a range of kernel methods to obtain summary clusterings, and otherwise exploit the information summarised by PSMs. For example, if we have multiple PSMs, each corresponding to a different dataset on a common set of statistical units, we may use standard methods for combining kernels in order to perform integrative clustering. We may moreover embed PSMs within predictive kernel models in order to perform outcome-guided data integration. We demonstrate the performances of the proposed methods through a range of simulation studies as well as two real data applications. R code is available at https://github.com/acabassi/combine-psms.
更多查看译文
关键词
similarity,kernel,posterior,learning
AI 理解论文
溯源树
样例
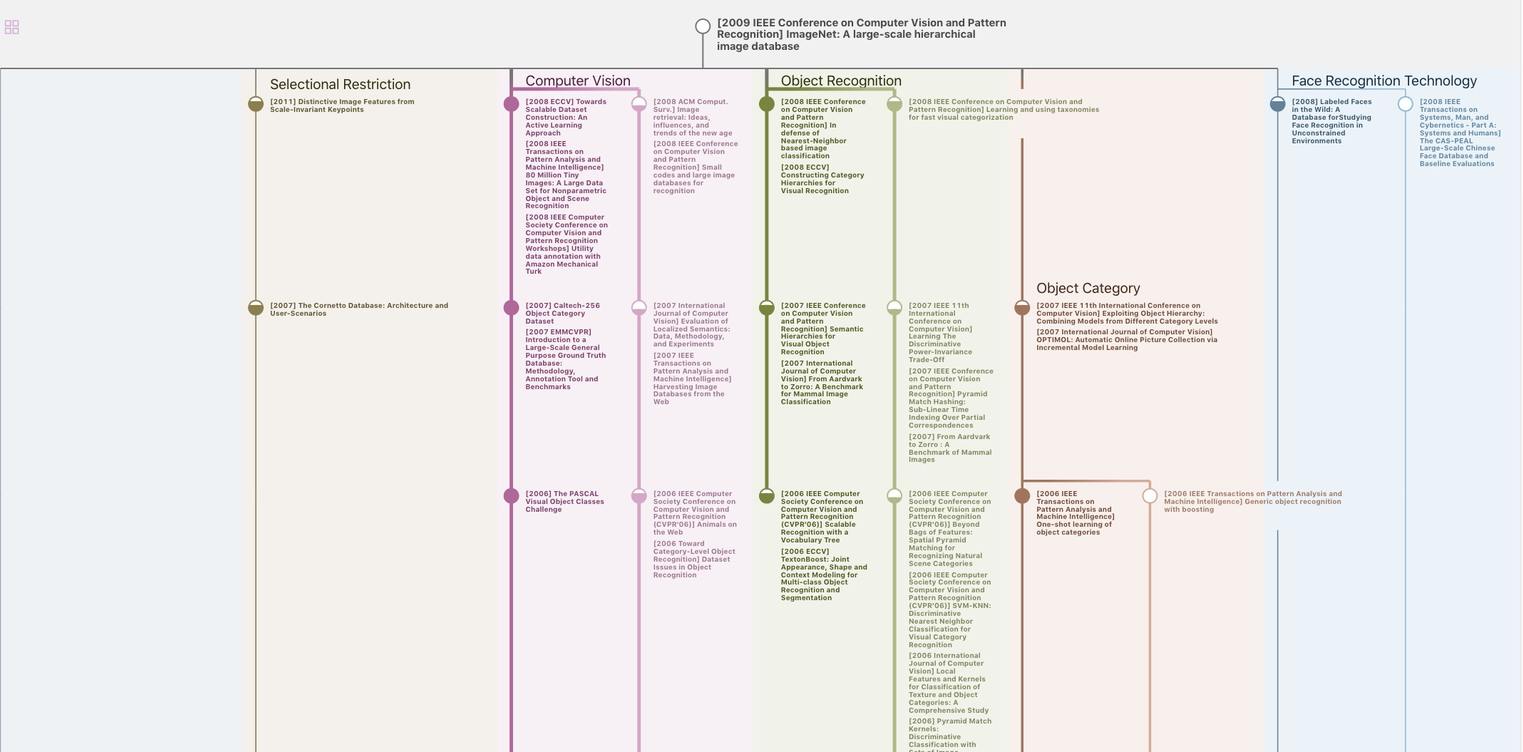
生成溯源树,研究论文发展脉络
Chat Paper
正在生成论文摘要